JASTE: An Efficient Multi-Task Model for Aspect Sentiment Triplet Extraction
Frontiers in Artificial Intelligence and ApplicationsDesign Studies and Intelligence Engineering(2022)
摘要
Aspect sentiment triplet extraction (ASTE) is a relative difficult and novel research, which is a subtask of aspect-based sentiment analysis (ABSA). ASTE is a task that extracts triplets of aspects being discussed, relevant opinion entities and sentiment polarities from a given sentence. Existing approaches mainly deal with this problem by pipeline or simple multi-task structure, which do not take full advantage of the strong correlation among the three elements of the triplet. In this work, we adopt two special tagging schemes, AOBIO and Pair Tagging Scheme (PTS), and propose an efficient end-to-end multi-task model named Joint Aspect Sentiment Triplet Extraction (JASTE) to address ASTE task. JASTE is composed of three modules: aspect and opinion extraction module, relation module and sentiment module. Specially, the relation module is designed to capture the relationship between aspect and opinion properly. The three modules interact with each other by sharing the same embedding. Extensive experimental results on different benchmark datasets show that JASTE can significantly outperform state-of-the-art performances.
更多查看译文
关键词
aspect sentiment triplet extraction,multi-task
AI 理解论文
溯源树
样例
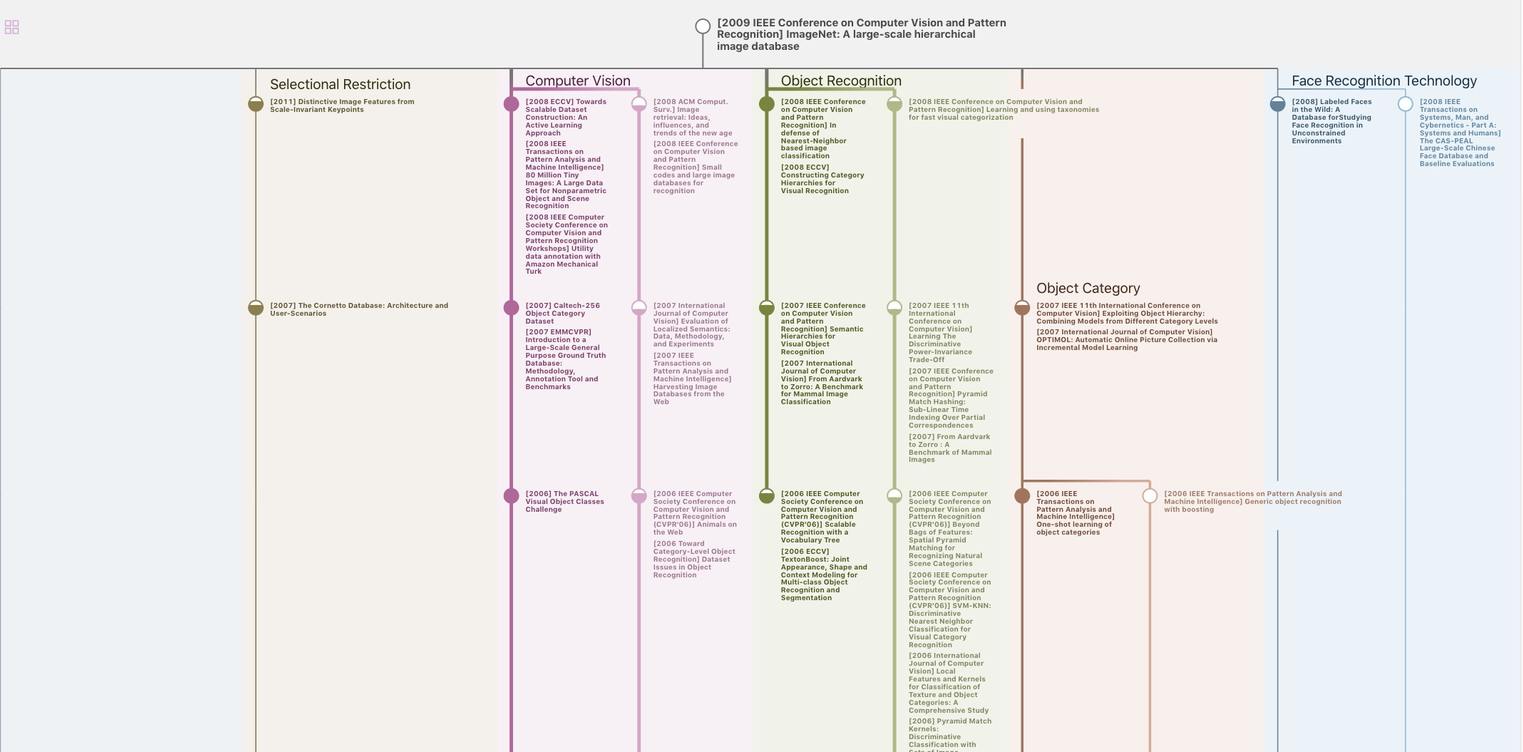
生成溯源树,研究论文发展脉络
Chat Paper
正在生成论文摘要