Attentive fine-grained recognition for cross-domain few-shot classification
Neural Computing and Applications(2022)
摘要
Cross-domain few-shot classification aims to recognize images in the new categories and domains that only contain few but unacquainted images. Considering the problems of fine-grained recognition in cross-domain few-shot classification including marginal overall-discrepancy in feature distribution and obvious fine-grained difference in dataset, this paper proposes a simple and effective attentive fine-grained recognition (AFGR) model. Specifically, the residual attention module is stacked into the feature encoder based on the residual network, which can linearly enhance different semantic feature information to help the metric function better locate the fine-grained feature information of the image. In addition, a bilinear metric function structure is proposed to learn and fuse different fine-grained image features, respectively, since the weights of bilinear measurement functions are not shared. Eventually, the final classification result is obtained by merging the recognition of bilinear metric function through posterior probability multiplication. In this paper, ablation experiments and comparative experiments are carried out with the typical few-shot dataset mini-ImageNet as the training domain and the CUB, Cars, Places and Plantae datasets as the test domain. The experimental results demonstrate that the proposed AFGR method is effective, with the highest increase in recognition accuracy 13.82% and 7.95% compared with the latest results under the experimental settings of 5-way1-shot and 5-way5-shot, respectively, which also proves the problems of fine-grained recognition in cross-domain small sample classification.
更多查看译文
关键词
Cross-domain, Few-shot classification, Fine-grained, Residual attention module, Metric function
AI 理解论文
溯源树
样例
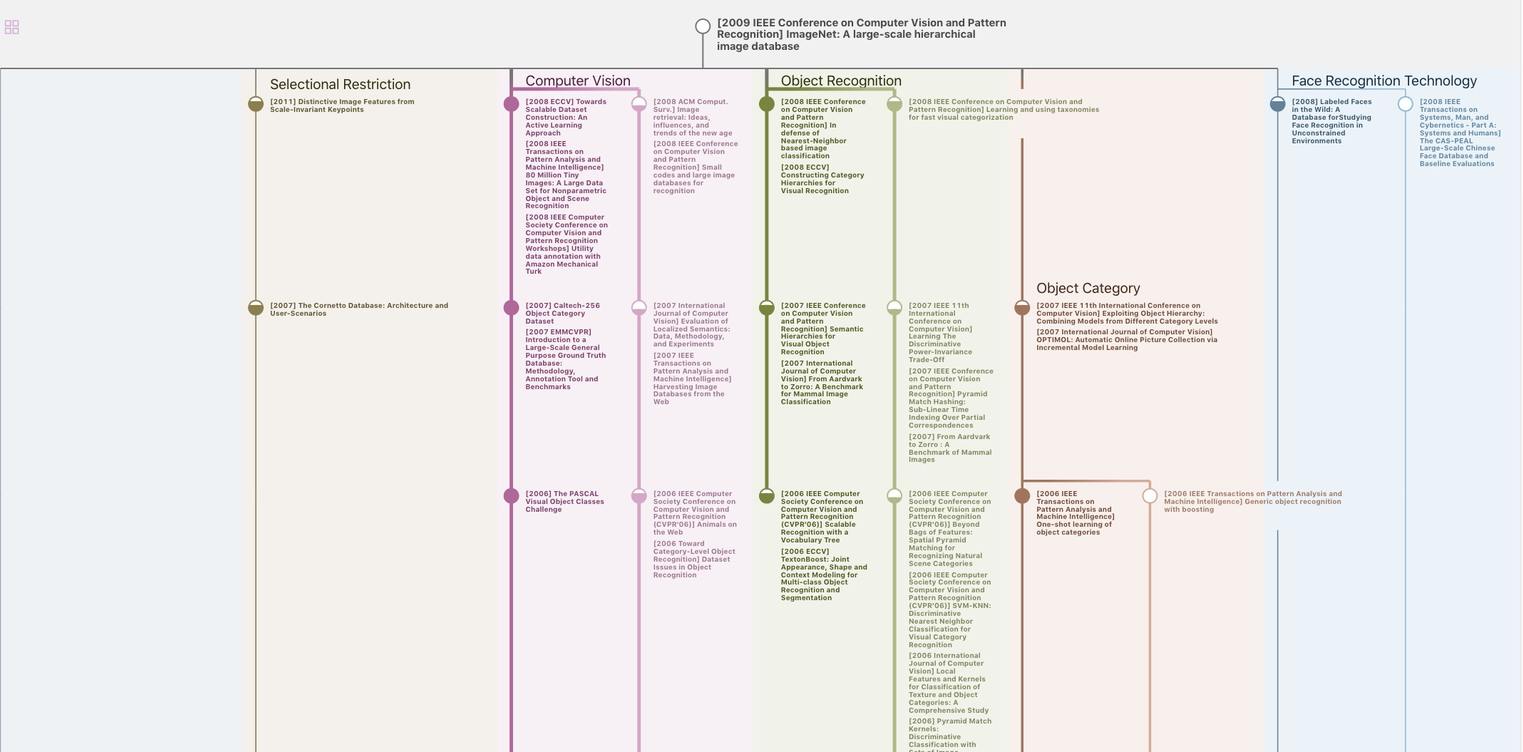
生成溯源树,研究论文发展脉络
Chat Paper
正在生成论文摘要