A semi-supervised learning method for surface defect classification of magnetic tiles
Machine Vision and Applications(2022)
摘要
Surface defect inspection is a crucial step to ensure the quality of magnetic tiles. Recently, deep learning methods have shown excellent performance on many vision tasks. Some deep learning-based methods have been applied to the surface defect inspection of magnetic tiles as well. However, related methods are based on supervised learning, which requires plenty of labeled samples to train deep neural networks. In industrial application scenarios, the annotation of large labeled datasets is extremely expensive, time-consuming, and error-prone. A semi-supervised learning method based on pseudo-labeling is proposed in this paper to address the problem of surface defect classification of magnetic tiles with limited labeled samples. The proposed method consists of two models: the teacher model and the student model. The training procedure is divided into two stages: pseudo-label generation and student model training. In the pseudo-label generation stage, the teacher model parameters and the pseudo-labels of unlabeled samples are alternatively optimized based on the idea of transductive learning. Curriculum learning is employed to reduce the impact of label noise so that high-quality pseudo-labels can be obtained. In the student model training stage, labeled samples and unlabeled samples with pseudo-labels are jointly used to train the classifier, with mixup to achieve information fusion and regularization. The experimental results show that the proposed method outperforms the supervised-only and semi-supervised baselines. With only 4.4% of labeled samples in the training set, the proposed method can still achieve the defect classification accuracy of 90.13%.
更多查看译文
关键词
Defect inspection,Magnetic tile,Pseudo-labeling,Semi-supervised learning
AI 理解论文
溯源树
样例
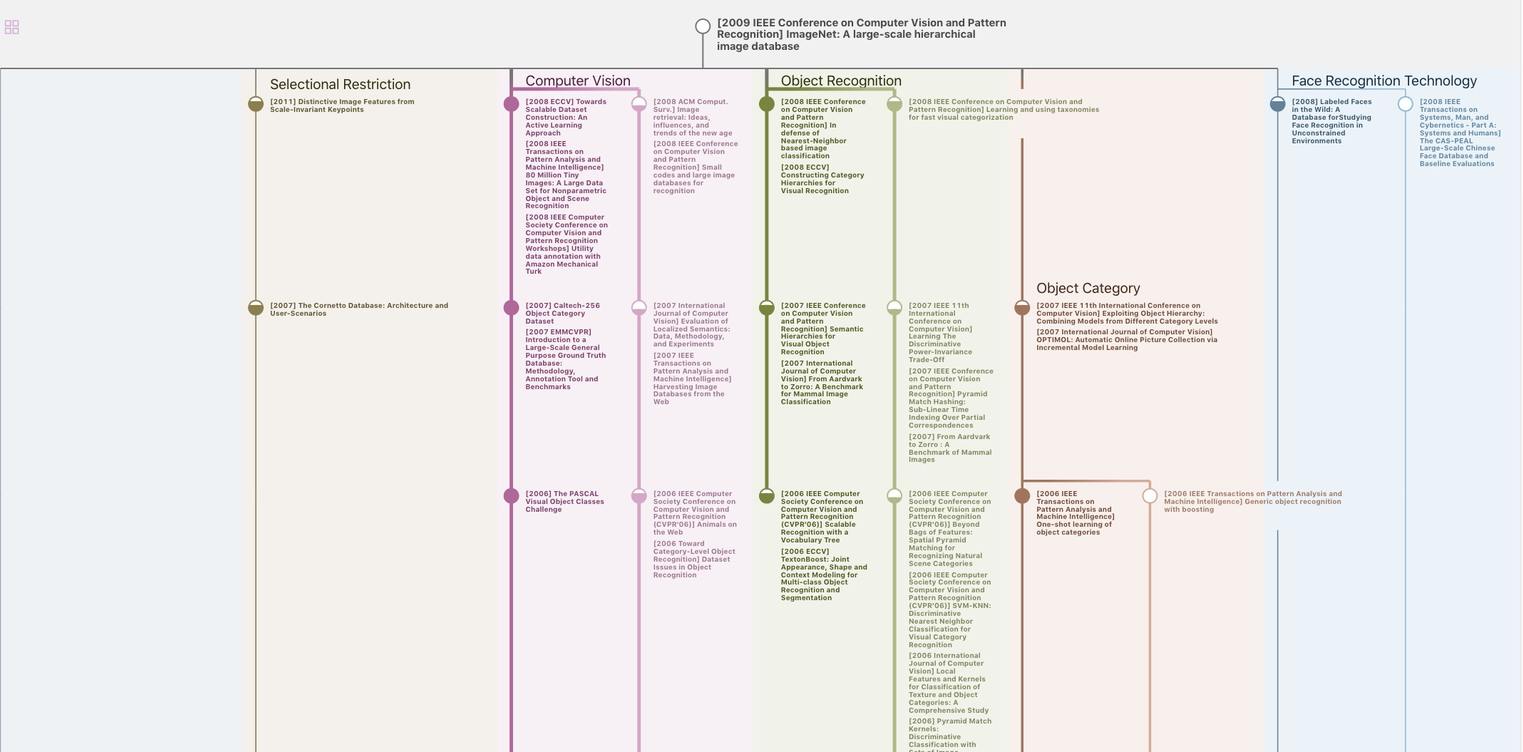
生成溯源树,研究论文发展脉络
Chat Paper
正在生成论文摘要