RL/DRL Meets Vehicular Task Offloading Using Edge and Vehicular Cloudlet: A Survey
IEEE Internet of Things Journal(2022)
摘要
The last two decades have seen a clear trend toward crafting intelligent vehicles based on the significant advances in communication and computing paradigms, which provide a safer, stress-free, and more enjoyable driving experience. Moreover, emerging applications and services necessitate massive volumes of data, real-time data processing, and ultrareliable and low-latency communication (URLLC). However, the computing capability of current intelligent vehicles is minimal, making it challenging to meet the delay-sensitive and computation-intensive demand of such applications. In this situation, vehicular task/computation offloading toward the edge cloud (EC) and vehicular cloudlet (VC) seems to be a promising solution to improve the network’s performance and applications’ Quality of Service (QoS). At the same time, artificial intelligence (AI) has dramatically changed people’s lives. Especially for vehicular task offloading applications, AI achieves state-of-the-art performance in various vehicular environments. Motivated by the outstanding performance of integrating reinforcement learning (RL)/deep RL (DRL) to the vehicular task offloading systems, we present a survey on various RL/DRL techniques applied to vehicular task offloading. Precisely, we classify the vehicular task offloading works into two main categories: 1) RL/ DRL solutions leveraging EC and 2) RL/DRL solutions using VC computing. Moreover, the EC section-based RL/DRL solutions are further subcategorized into multiaccess edge computing (MEC) server, nearby vehicles, and hybrid MEC (HMEC). To the best of our knowledge, we are the first to cover RL/DRL-based vehicular task offloading. Also, we provide lessons learned and open research challenges in this field and discuss the possible trend for future research.
更多查看译文
关键词
Artificial intelligence (AI),deep reinforcement learning (DRL),machine learning,reinforcement learning (RL),task offloading,vehicle to roadside infrastructure (V2I),vehicle to vehicle (V2V),V2X communications,vehicular edge and cloudlet computing
AI 理解论文
溯源树
样例
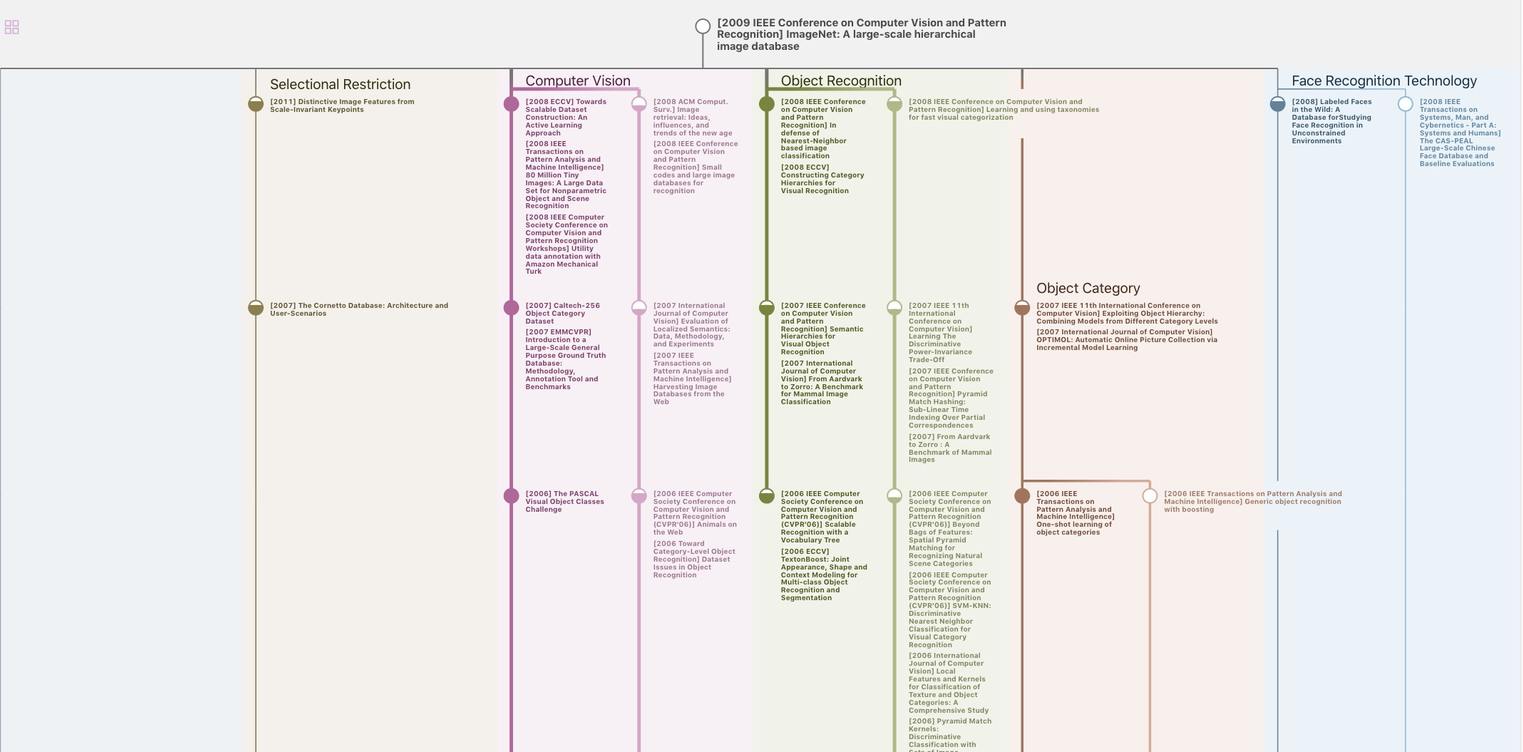
生成溯源树,研究论文发展脉络
Chat Paper
正在生成论文摘要