Mixture optimisation for cement-soil mixtures with embedded GFRP tendons
Journal of Materials Research and Technology(2022)
摘要
The glass fiber-reinforced polymer (GFRP) rebar reinforced cemented soil is widely employed to solve the weak foundation problem led by sludge particularly. The robustness of this structure is highly dependent on the interface bond strength between the GFRP tendon and cemented soils. However, its application is obstructed owing to the deficient studies on the influence factors. Therefore, this study investigates the effects of water content (Cw: 50%–90%), cement proportion (Cc: 6%–30%), and curing period (Tc: 28–90 days) on peak and residual interface bond strengths (Tp and Tt), as well as the unconfined compression strength (UCS). Results indicated that mechanical properties were positively responded to Tc and Cc, while negatively correlated to Cw. Besides, Random Forest (RF), one of the machine learning (ML) models, was developed with its hyperparameters tuned by the firefly algorithm (FA) based on the experimental dataset. The pullout strength was predicted by the ML model for the first time. High correlation coefficients and low root-mean-square errors verified the accuracy of established RF-FA models in this study. Subsequently, a coFA-based multi-objective optimisation firefly algorithm (MOFA) was introduced to optimise tri-objectives between UCS, Tp (or Tt), and cost. The Pareto fronts were successfully acquired for optimal mixture designs, which contributes to the application of GFRP tendon reinforced cemented soil in practice. In addition, the sensitivity of input variables was evaluated and ranked.
更多查看译文
关键词
Cemented soil,Interface bond strength,Glass fiber reinforced polymer reinforcement,Machine learning,Multi-objective optimisation
AI 理解论文
溯源树
样例
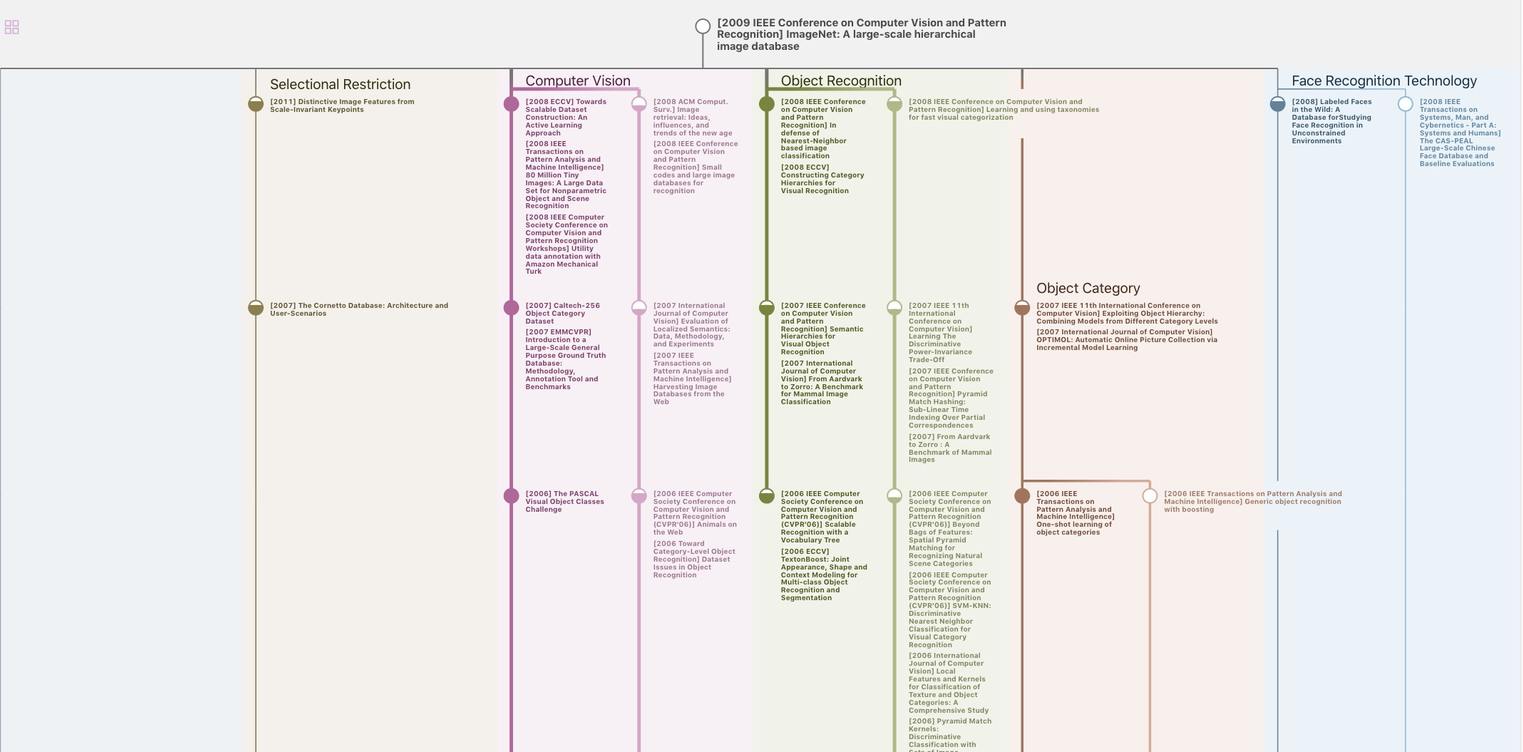
生成溯源树,研究论文发展脉络
Chat Paper
正在生成论文摘要