A Fast Optimization Algorithm Based on Multi-RBF For High-Dimensional and Computationally Expensive Problems
2021 4th International Conference on Algorithms, Computing and Artificial Intelligence(2021)
摘要
Optimization problems of the numerical model in the engineering field usually involve many undetermined parameters and computationally expensive simulations. Using evolutionary algorithms alone is inefficient because it takes up thousands of engineering simulations to obtain a good solution. Recently, surrogate-assisted evolutionary algorithms have been widely researched, which train a surrogate model (GP, RBF, SVM) to replace mostly computation of origin engineering model. But most surrogate model-based algorithms still require more than 1000 times evaluations to get reasonable solutions by origin model. To further improve optimization efficiency, a new ensemble surrogate modeling-based and some novel in-fill strategies-assisted fast optimization algorithm (ESMO) is proposed for solving high-dimensional and computationally expensive problems in this work. ESMO employs Radial Basis Function Neural Network (RBF) as a surrogate model. ESMO prepares three different methods to build multi-RBF and corresponding in-fill strategies to trade-off exploration and exploitation. To guarantee fast optimization, a constraint-region method is also applied. Empirical studies demonstrate that ESMO shows much better performance than other state-of-the-art algorithms within 500 function evaluations.
更多查看译文
AI 理解论文
溯源树
样例
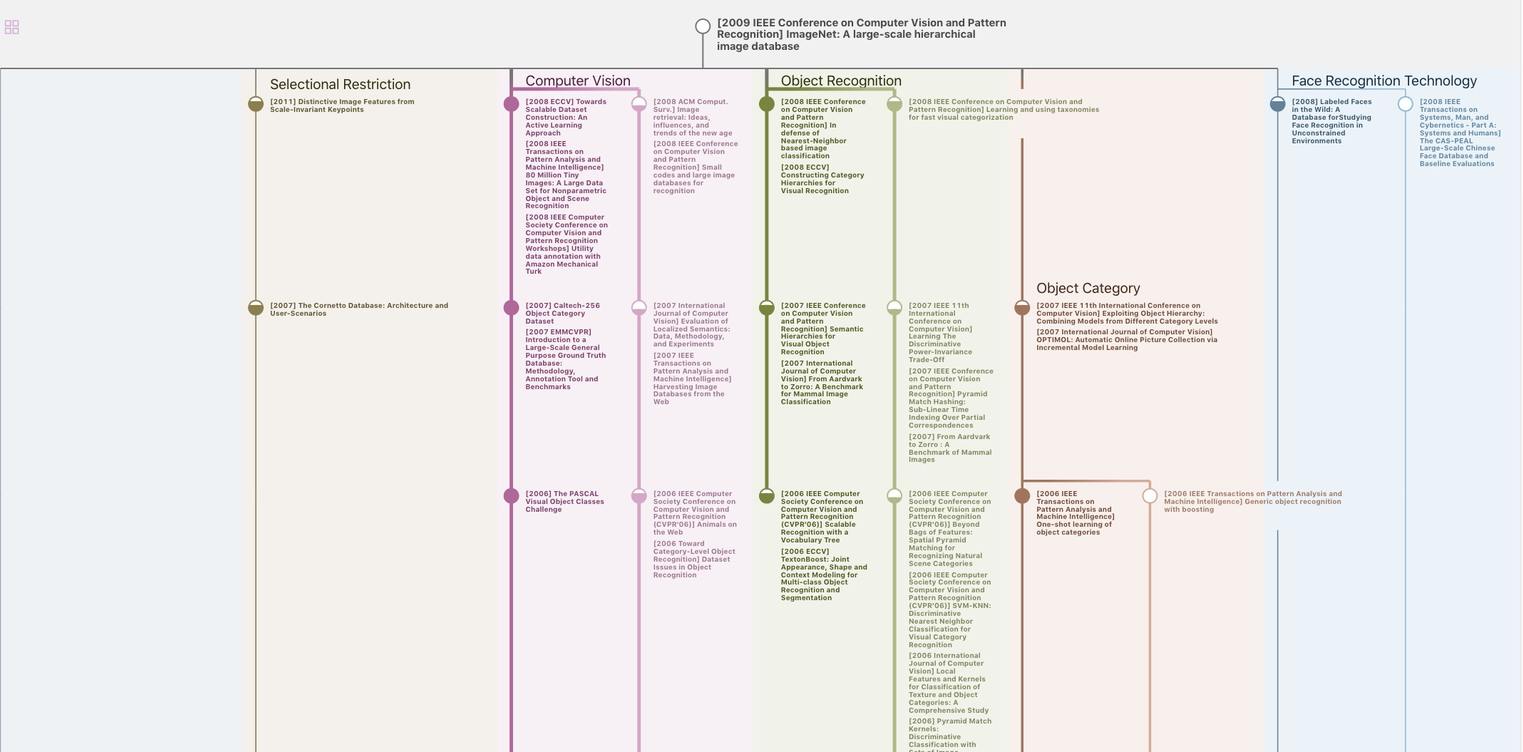
生成溯源树,研究论文发展脉络
Chat Paper
正在生成论文摘要