Next-Cycle Optimal Dilute Combustion Control via Online Learning of Cycle-to-Cycle Variability Using Kernel Density Estimators
IEEE Transactions on Control Systems Technology(2022)
摘要
Dilute combustion using exhaust gas recirculation (EGR) presents a cost-effective method for increasing the efficiency of spark-ignition (SI) engines. However, the maximum amount of EGR that can be used at a given condition is limited by a rapid increment of cycle-to-cycle variability (CCV). This study describes a methodology to design a model-based stochastic optimal controller to adjust the cycle-to-cycle fuel injection quantity in order to reduce CCV and further extend the dilute limit. Given the complexity and chaotic nature of combustion events, the controller was enhanced with online learning in order to identify the statistical properties of combustion efficiency, which are needed to generate predictions for next-cycle events. This study showed that a kernel density estimator (KDE) can be used to learn the combustion properties in real time and can be incorporated into the feedback policy in order to calculate the optimal control command. Experimental results suggested that the dilute limit can be extended from 18.5% to 21% EGR fraction at an operating condition relevant for highway cruising. Additionally, the proposed controller can achieve a large CCV reduction with less fuel enrichment compared to previous methods, overall contributing to an increase in 0.2% indicated fuel conversion efficiency.
更多查看译文
关键词
Internal combustion engines,kernel density estimation,optimal control,statistical learning,stochastic systems
AI 理解论文
溯源树
样例
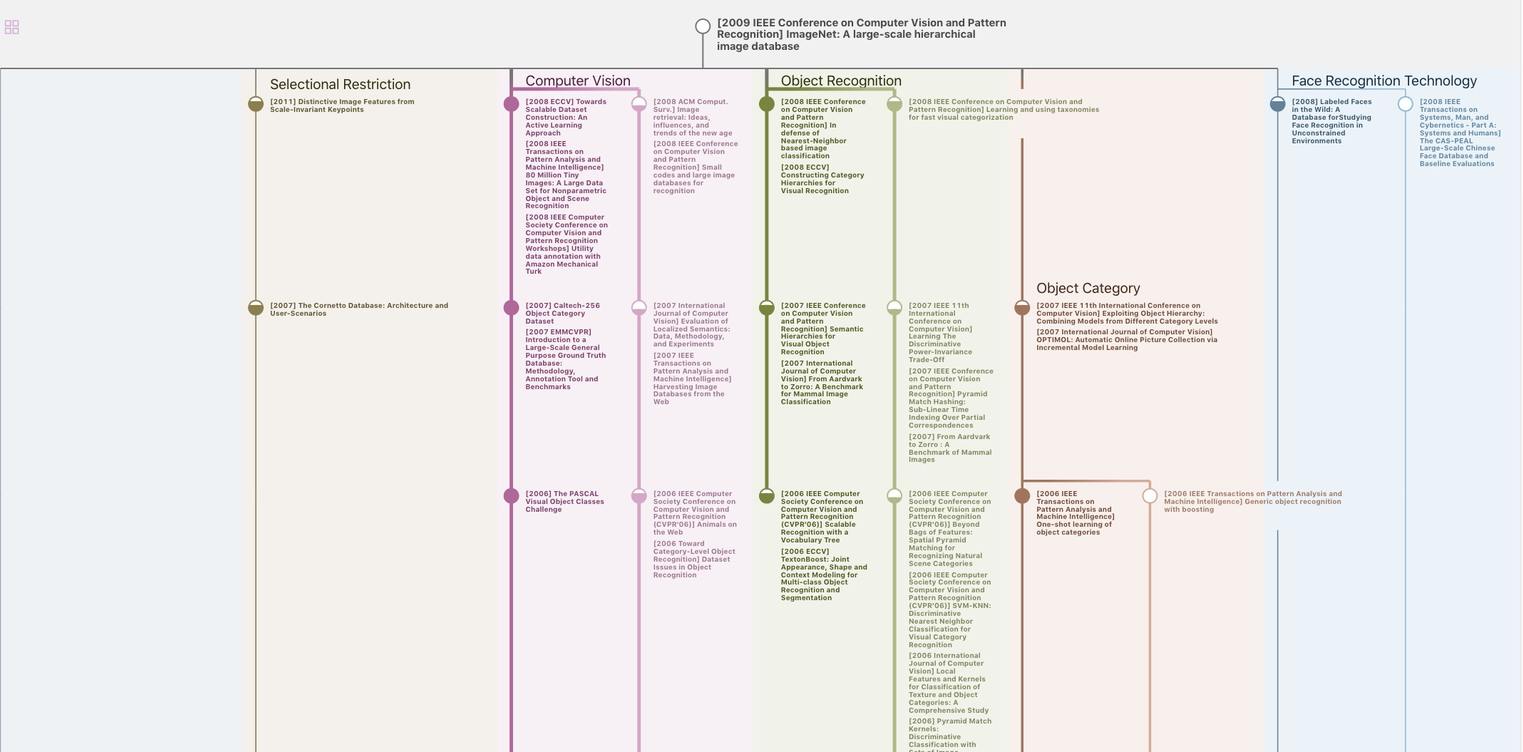
生成溯源树,研究论文发展脉络
Chat Paper
正在生成论文摘要