Multi-omics fusion analysis models with machine learning predict survival of HER2-negative metastatic breast cancer: a multicenter prospective observational study
SSRN Electronic Journal(2023)
摘要
To the Editor: Oncology precision medicine aims to identify patients most likely to respond effectively to therapies. Efforts to establish a survival prediction model using a single platform have not yet met the precision medicine goals.[1] Circulating tumor DNA (ctDNA), assessed by liquid biopsy, is representative of tumor heterogeneity, and ctDNA levels hold the potential for monitoring treatment response.[2] Moreover, circulating tumor cells (CTCs) in the metastatic cascade have been identified as clinical factors associated with poor prognosis.[3] The added value obtained from multi-omics fusion analysis to improve predictive models requires further investigation.[4] This study was approved by the Independent Ethics Committee of the National Cancer Center (CH-BC-023), and written informed consent was obtained from all patients before their enrolment. In this study, we characterized the biological parameters extracted from a subset of enrolled patients with human epithelial growth factor receptor-2 (HER2) negative metastatic breast cancer (MBC) in the CAMELLIA study (ClinicalTrials.gov: NCT01917279). Seventy patients were treated with the docetaxel plus capecitabine (TX) chemotherapy regimen during the initial period, that is, capecitabine (1000 mg/m2, twice daily, days 1–14, every three weeks) in combination with docetaxel (75 mg/m2, day 1, every three weeks) for six cycles [Supplementary Table 1, https://links.lww.com/CM9/B458]. All patients underwent contrast-enhanced Computer Tomography (CT) (Discovery CT750/ GoldSeal Optima CT660, GE HealthCare Life Sciences, Chicago, IL, United States) with a scanning layer thickness of 5 mm and an interval of 0. Peripheral blood samples (5 mL, two tubes) were prospectively collected at baseline to perform next-generation sequencing of ctDNA and CTC analyses. We optimized CanPatrol®CTC enrichment technology (SurExam Bio-Tech Co., Ltd, Guangzhou, China) to detect CTC clusters. Targeted region capture and next-generation sequencing customized probes (Integrated DNA Technologies, Coralville, IA, United States) covering −1.5 Mbp genome and 1021 cancer-related genes were used to detect somatic variants in ctDNA [Supplementary Table 2, https://links.lww.com/CM9/B458]. Mutations were enriched using R package clusterProfiler (version 3.18.1, https://www.r-project.org) by querying the following annotated databases: Gene Ontology (GO) and Kyoto Encyclopedia of Genes and Genomes (KEGG) [Supplementary Figures 1 and 2, https://links.lww.com/CM9/B458]. We combined ctDNA and CTC features by a fusion method based on canonical correlation analysis (CCA) to obtain a single feature matrix. corr(X*,Y*)=cov(X*,Y*)var(X*)⋅var(Y*) where X*=WxTX, Y*=WyTY. X and Y are two feature matrices; Wx and Wy are transformation matrices; cov(X*,Y*)=WxTSxyWy, var(X*)=WxTSxxWx, var(Y*)=WyTSyyWy. In this study, we used the summation method to obtain the fused feature vectors with the minimum rank of the original feature matrices: Z=X*+Y*=WxTX+WyTY where Z denotes the canonical correlation discriminant features. A total of 1218 quantitative CT radiomic features were extracted from regions of interest and manually segmented using Pyradiomics (version 3.0.1, https://github.com/Radiomics/pyradiomics) in Python (Python Software Foundation. Python Language Reference, version 2.7, available at http://www.python.org). Each patient feature was normalized to Z-scores, and dimension reduction was ensured by limiting the variance to a 0.010 threshold. We selected the features using the Wilcoxon–Mann–Whitney test with P value < 0.20, then conducted single-factor Cox regression, and selected the features with a concordance index (C-index) value >0.50. We performed a descriptive analysis of progression-free survival and overall survival (OS) as valid survival endpoints. Considering the restricted sample size, we performed leave-one-out cross-validation. The Youden index was chosen to divide the risk scores into high or low signatures. The Harrell C-index and time-dependent receiver operating characteristic (TD-ROC) curves, with quantified areas under the curves (AUCs), were calculated to evaluate and verify model performance. A flowchart of the process is shown in Supplementary Figure 3A, https://links.lww.com/CM9/B458. Seventy patients were enrolled in the study. Clinical characteristics are summarized in Supplementary Table 1, https://links.lww.com/CM9/B458. A total of 180 mutations were identified. GO and KEGG enrichment analysis showed 25 dimensions of breast cancer-associated genes with P = 1.91 × 10–18; Padjust = 3.96 × 10–16 [Supplementary Figure 4, https://links.lww.com/CM9/B458]. In the entire cohort, 950 CTCs were detected. Correlation analysis of CTCs showed more textural features than first-order and shaped features [Supplementary Table 3, https://links.lww.com/CM9/B458]. Supplementary Figure 5, https://links.lww.com/CM9/B458 shows the close association between the selected radiomic features and CTC/ctDNA genetic alterations. A series of prediction models were developed: (1) clinical features only, (2) clinical + CCA model, and (3) multi-omics fusion model. The C-index of the multi-omics fusion model was 0.725 (Hazard ratio [HR] 3.812, 95% confidence interval [CI] 2.245–6.472, P < 0.0001), which was superior to that of the other two models (P = 0.0086 and P = 0.0137, respectively) [Supplementary Tables 4–6 and Supplementary Figure 3B, https://links.lww.com/CM9/B458]. TD-ROC analysis assessed the accuracy of prognosis at specific time points of 1, 2, and 3 years, with an AUC ranging between 0.866 and 0.918 [Supplementary Figure 3B, https://links.lww.com/CM9/B458]. For OS prediction, the multi-omics fusion model also showed statistically significantly better performance, with a C-index of 0.811 (HR 5.992, 95% CI 3.145–11.419, P < 0.0001) [Supplementary Table 4 and Supplementary Figure 3B, https://links.lww.com/CM9/B458]. C-index comparison was performed to statistically validate the improvement of the multi-omics fusion model over the other two models (P < 0.0001, P < 0.0001) [Supplementary Tables 5–6, https://links.lww.com/CM9/B458]. In the TD-ROC analysis, the AUC of OS of multi-omics model was 0.815–0.989 [Supplementary Table 4 and Supplementary Figure 3B, https://links.lww.com/CM9/B458]. Further evaluation of the multi-omics fusion model also showed good predictive ability in the following subgroups: age <50 years, regardless of the number of metastatic organs, liver and lung metastases, and those who received prior adjuvant therapy and first-line endocrine therapy for metastasis [Supplementary Figures 6–8 https://links.lww.com/CM9/B458]. The response to treatment is modulated by a tumor ecosystem whose multi-omics landscape can be integrated into a predictive model by machine learning. Previously, efforts to identify predictive characteristics largely ignored this issue.[1] Taking advantage of important breakthroughs in CTC/ctDNA detection,[2,3] our pilot study features the innovative application of radiomics combined with liquid biopsy technique (ctDNA/CTC assay) to noninvasively provide more comprehensive information beyond visual perception. In the clinical workflow, predictive models can advance the clinical management of MBC and guide therapeutic strategies, ultimately leading to improved survival outcomes.[4] The tumor evolution in patients can be precisely predicted at a macroscopic level, represented by clinicopathological phenotypes and radiomics, as well as at the cellular level, represented by CTCs in liquid-based biopsies, and at the molecular level, represented by ctDNA. Although ctDNA and CTCs are seldom used simultaneously,[3] we hypothesized that serial analysis of both peripheral biomarkers would appeal more to patients. Here, we optimized the data fusion process, and established an efficient and accurate survival prediction algorithm model. These multi-omics fusion predictive models can help identify MBC candidates who may benefit from palliative chemotherapy. This study has some limitations. First, it was limited by its small sample size. A larger cohort study would help to optimize the cutoff values. Second, the prediction model may require further external prospective intervention studies to validate. In conclusion, our pretreatment multi-omics fusion models with machine learning effectively predicted the survival of HER2− MBC patients with good discrimination power and outperformed conventional clinicopathological models. This framework highlights the importance of data integration with machine learning and is widely used to generate predictors by including various newer features. Acknowledgements We sincerely thank the patients who participated and all the staff who assisted in this study. Funding This study was supported by the National Key Research and Development Program of China (2021YFF1201300 and 2021YFF1201003), the National Natural Science Foundation of China (Nos. 81971662, 92259301, 92159301 and 92059103), the Natural Science Foundation of Beijing City (7202105), and the Key Project of Beijing Hope Marathon Special Fund from the China Cancer Foundation (LC2018A20). Conflicts of interest None.
更多查看译文
关键词
breast cancer,fusion,machine learning,multi-omics
AI 理解论文
溯源树
样例
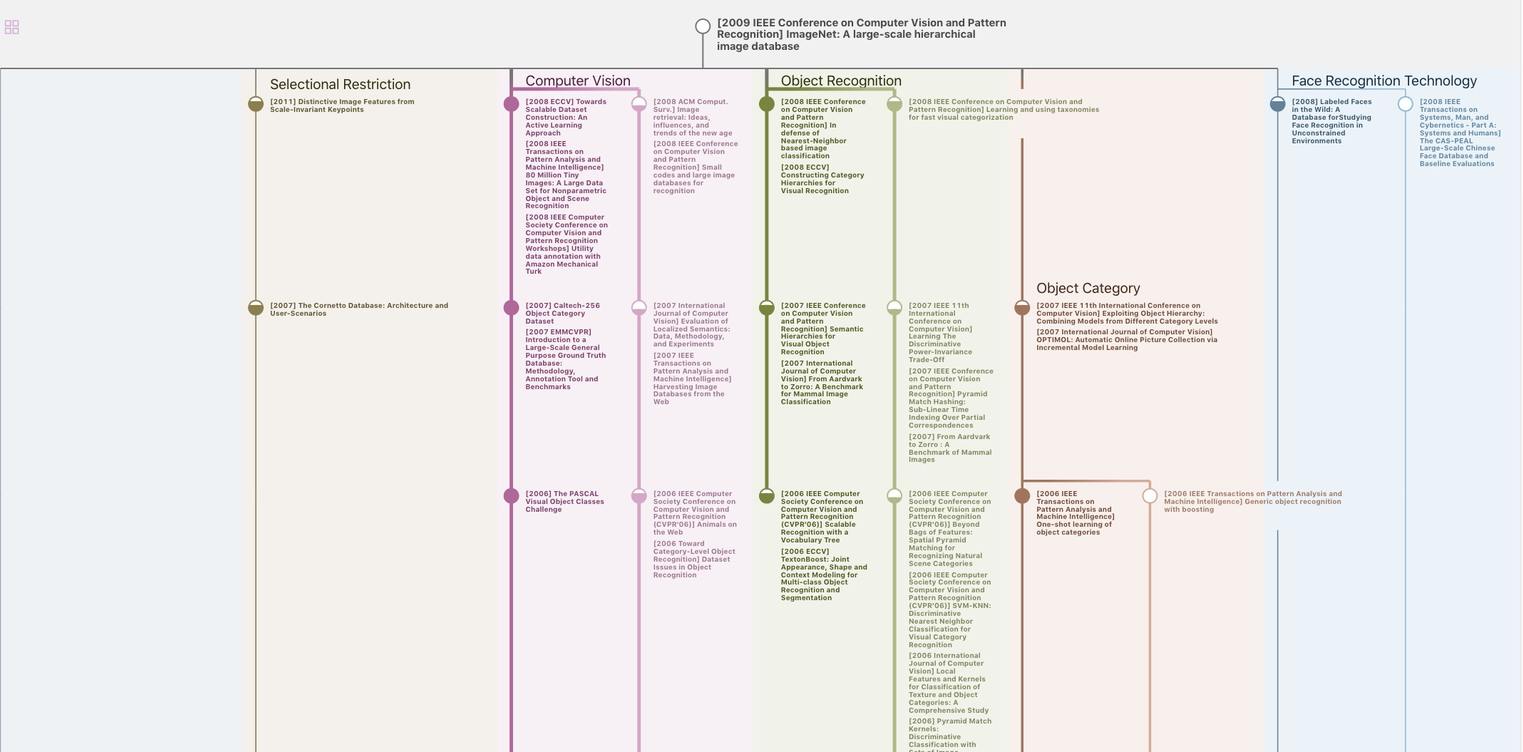
生成溯源树,研究论文发展脉络
Chat Paper
正在生成论文摘要