Automated Concept Drift Handling for Fault Prediction in Edge Clouds Using Reinforcement Learning
IEEE Transactions on Network and Service Management(2022)
摘要
Fault management systems that use real-time analytics based on Machine Learning (ML) help provide the reliability required in edge clouds, though they suffer from frequent changes in data distribution (concept drift) caused by highly dynamic traffic in edge clouds, requiring frequent adaptations of the ML model. We propose an automated concept drift handling framework for fault prediction in edge clouds using Reinforcement Learning (RL) to select the most appropriate drift adaptation method as well as the amount of data needed for adaptation, while considering edge cloud operator’s requirements. We implemented an edge cloud testbed and introduced infrastructure and network faults to it in the presence of abrupt and incremental concept drift. According to the obtained results, our proposed framework achieves up to 40% higher accuracy compared to a system without drift handling, and up to
$13\times $
and
$30\times $
less regret for selecting adaptation methods and amount of data, respectively, compared to other approaches.
更多查看译文
关键词
Fault prediction,edge cloud,concept drift,machine learning,reinforcement learning
AI 理解论文
溯源树
样例
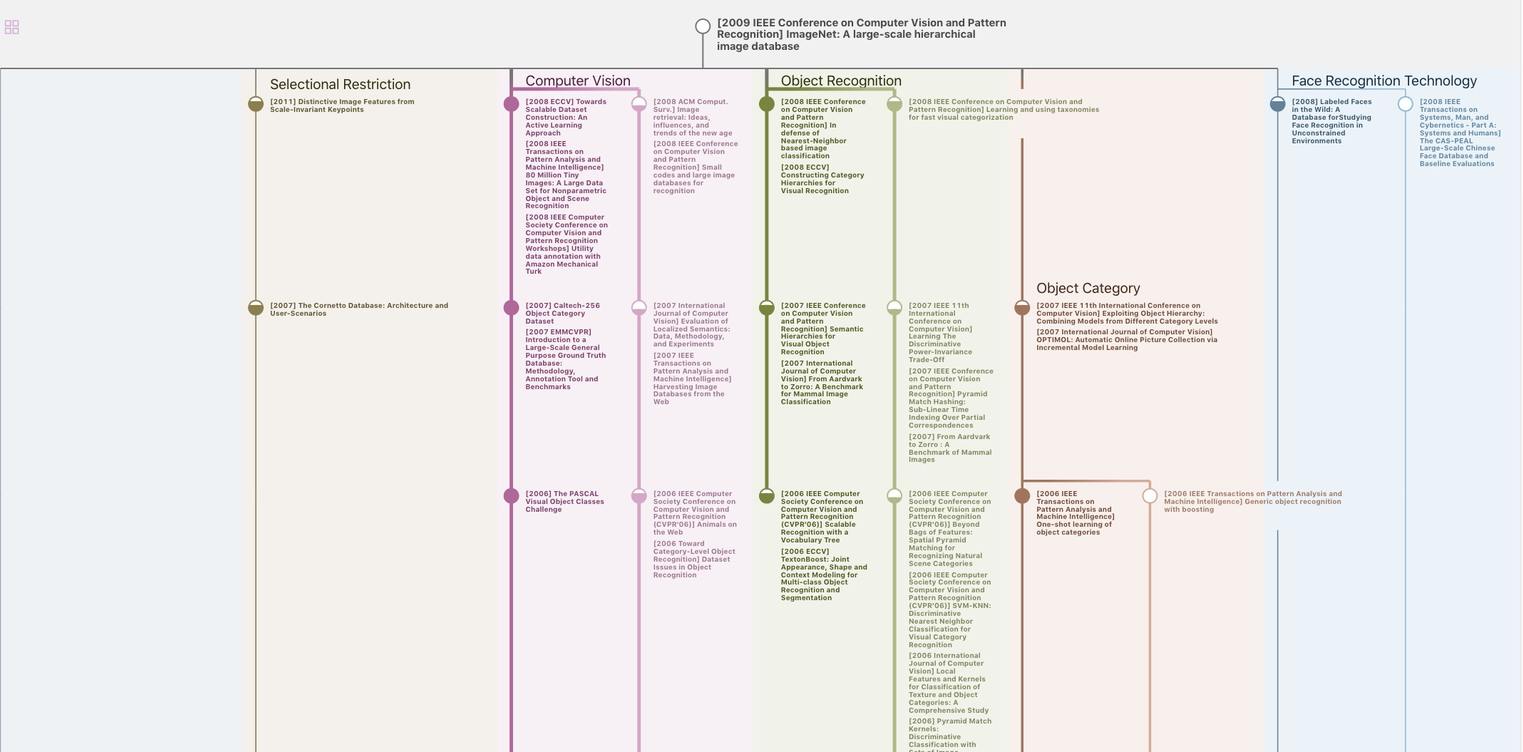
生成溯源树,研究论文发展脉络
Chat Paper
正在生成论文摘要