Entropy-based kernel graph cut for textural image region segmentation
Multimedia Tools and Applications(2022)
摘要
Recently, image segmentation based on graph cut methods has shown impressive performance on a set of image data. Although kernel graph cut provides more comprehensive performance, its performance is largely dependent on intensity values of the input image. Meanwhile kernel graph cut is not well-performed for textural images. This paper investigated entropy-based kernel graph cut image segmentation. The method consists of incorporating 2-layer feature space (1-layer gray level and 1-layer entropy feature) and minimizing an objective function to have localized and intensity-based comparison. By taking the advantage of a new feature space, the objective function comprises a data term to assess the transformed data deviation within each region of segmented image and a boundary regularization term. The proposed method supersedes modeling of the non-textural and complex textural images efficiently while taking advantage of the graph cuts computational profits. Experimentations were carried out over a collection of (real and synthetic) datasets to demonstrate the superior performance of the entropy-based kernel as compared to the state-of-the-art methods in energy-based image segmentation. The texture of the artificial images was created manually using the Color Brodatz, Fabric and DTD datasets. The simulation results report the maximum accuracy of the proposed solution on artificial and real images as 96.90% and 92.45%, respectively.
更多查看译文
关键词
Image segmentation,Kernel graph cut,Entropy,Texture
AI 理解论文
溯源树
样例
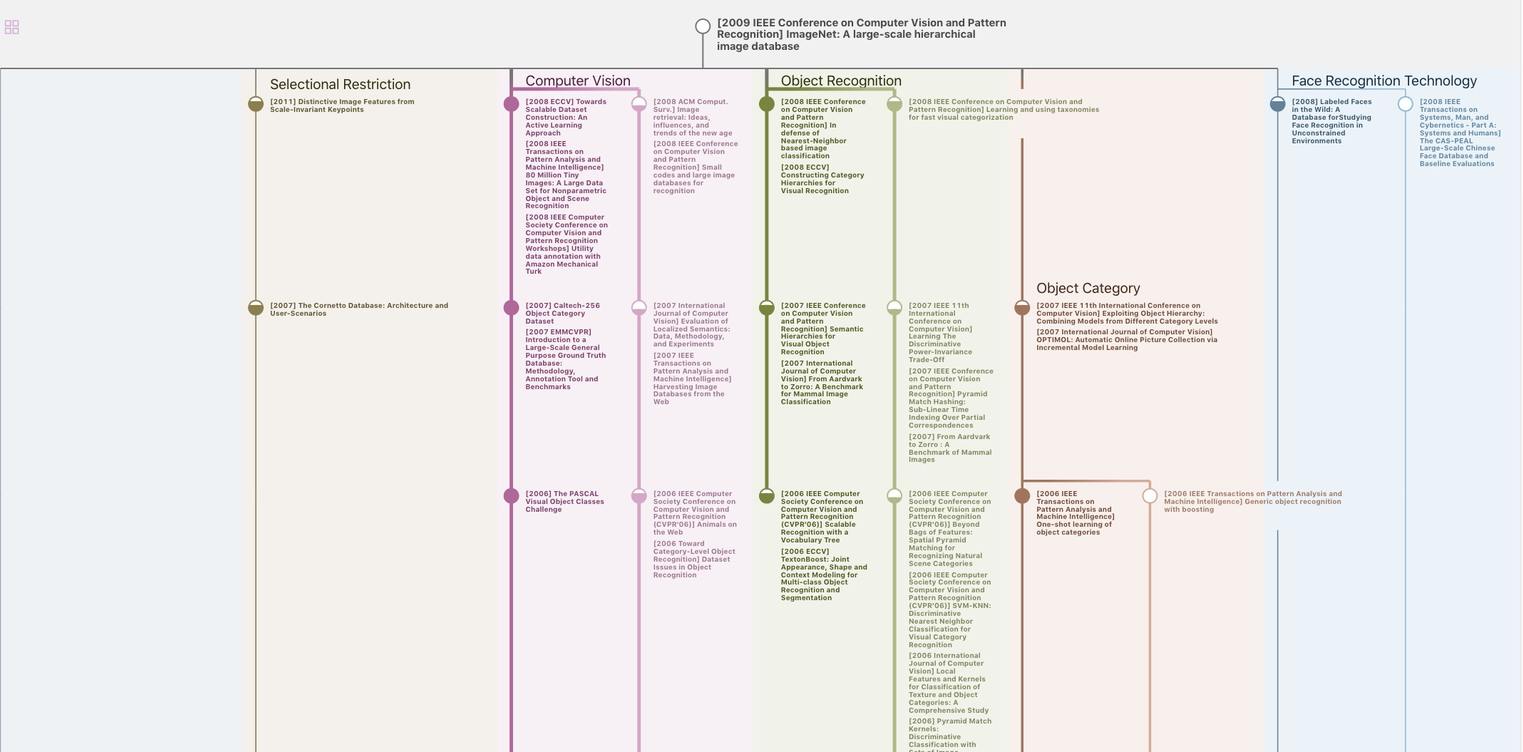
生成溯源树,研究论文发展脉络
Chat Paper
正在生成论文摘要