Multi-task deep learning model based on hierarchical relations of address elements for semantic address matching
Neural Computing and Applications(2022)
摘要
Address matching, which aims to match unstructured addresses with standard addresses in an address database, is a key part of geocoding. The core problem of address matching corresponds to text matching in natural language processing. Existing rule-based methods require human-designed templates and thus, have limited applicability. Machine learning and deep learning-based methods ignore the hierarchical relations between address elements, which easily misclassify semantically similar but geographically different locations. We note that the hierarchy of address elements can fill the semantic gap in address matching. Inspired by how humans discriminate addresses, we propose a multi-task learning approach. The approach jointly recognises the address elements and matches the addresses to incorporate the hierarchical relations between the address elements into the neural network. Simultaneously, we introduce a priori information on the hierarchical relationship of address elements through the conditional random field model. Experimental results on the benchmark datasets Shenzhen Address Database and Jiangsu-Hunan Address Dataset demonstrate the effectiveness of our approach. We achieved state-of-the-art F 1 scores (i.e. the harmonic mean of precision and recall) of 99.0 and 94.2 on the two datasets, respectively.
更多查看译文
关键词
Address matching, Multi-task learning, Recognizes the address elements, Hierarchical relations between address elements
AI 理解论文
溯源树
样例
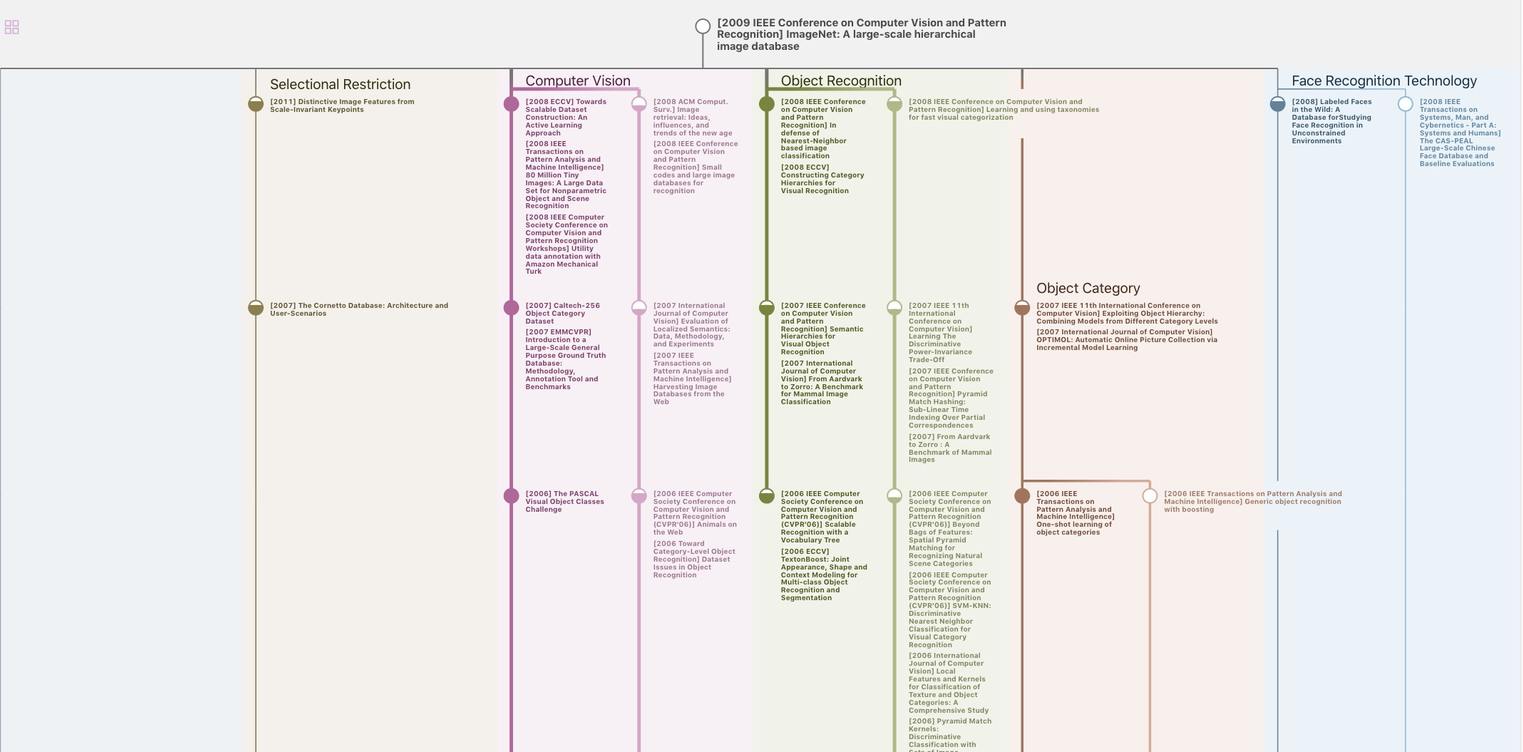
生成溯源树,研究论文发展脉络
Chat Paper
正在生成论文摘要