Optimal Cross‐Validation Strategies for Selection of Spatial Interpolation Models for the Canadian Forest Fire Weather Index System
Earth and Space Science(2022)
摘要
The Canadian forest fire weather index (FWI) system requires spatially continuous, gridded weather data for temperature, relative humidity, wind speed, and precipitation. Reliable estimates of the Canadian FWI system components are needed to ensure the safety of communities, resources, and ecosystems. The quality of the interpolated input weather variables are typically evaluated using error estimates from cross-validation. These error estimates are used for selecting between spatial interpolation methods for generating the continuous weather surfaces. Leave-one-out cross-validation (LOOCV) is the most commonly used method, but it is biased in spatially clustered weather station networks. Accurate error estimation is important for selecting the optimal interpolation method and evaluating how well an interpolated surface represents true patterns in a weather variable. Other cross-validation methods may better account for bias relating to clustered weather station networks. We present a comparison of cross-validation methods for evaluating spatial interpolation models of weather variables for generating the inputs to the Canadian FWI system with the objective of determining whether they identify the same spatial interpolation model as having the lowest error. We found that LOOCV, shuffle-split, stratified shuffle-split, and a modified buffered leave-one-out procedure generally identified the same spatial interpolation models as having the lowest error. Spatial k-fold favored spatial interpolation models with extrapolation ability. Our findings indicate that the most computationally efficient cross-validation approach can be used for automatically selecting spatial interpolation models for weather surface generation, which will improve the quality of historical daily FWI maps. Plain Language Summary Fire weather forecasting using the Canadian forest fire weather index (FWI) system requires high quality weather maps of temperature, relative humidity, wind speed, and precipitation to ensure the safety of communities and ecosystems. These maps are created using weather stations to estimate values for different weather variables across a study area. They are evaluated using crossvalidation, where certain weather stations are left out when producing the maps to estimate error using the observed and predicted values. These error estimates are used to select between maps created using different methods. Leave-one-out cross-validation (LOOCV), where each station is omitted sequentially, is a common approach. LOOCV may not be able to accurately estimate error if there are clustered stations. Other crossvalidation methods, such as stratified shuffle-split, may better account for the impact of clustered stations on the error estimate. Here, we compare different cross-validation strategies for evaluating weather maps for calculating the components of the Canadian FWI system. We found that many of the tested strategies selected the same method of generating the weather maps, which means that the fastest one can be used for generating historical Canadian FWI system maps.
更多查看译文
关键词
spatial interpolation, cross-validation, Canadian forest fire weather index system
AI 理解论文
溯源树
样例
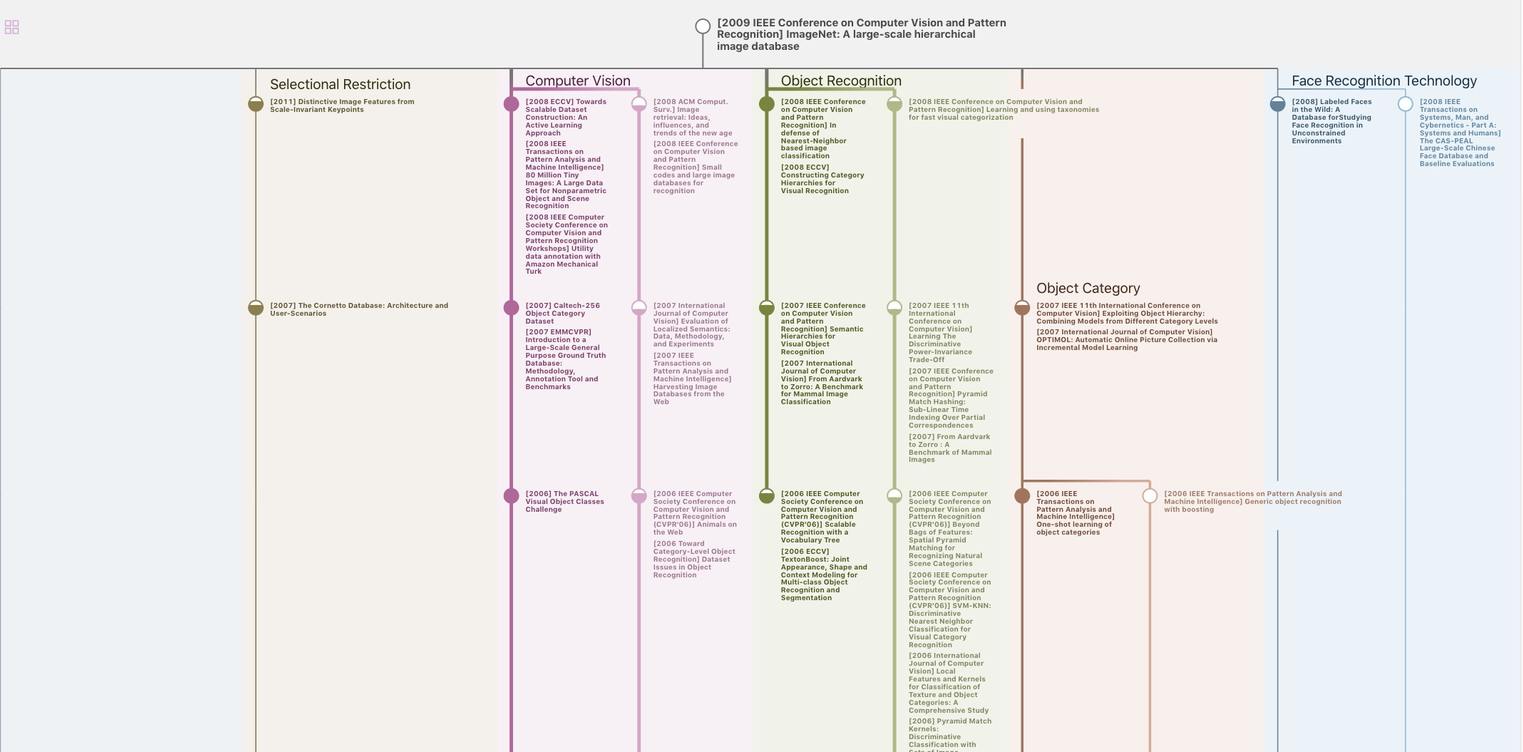
生成溯源树,研究论文发展脉络
Chat Paper
正在生成论文摘要