Cell type annotation of single-cell chromatin accessibility data via supervised Bayesian embedding
Nature Machine Intelligence(2022)
摘要
Recent advances in single-cell technologies have enabled the characterization of epigenomic heterogeneity at the cellular level. Computational methods for automatic cell type annotation are urgently needed given the exponential growth in the number of cells. In particular, annotation of single-cell chromatin accessibility sequencing (scCAS) data, which can capture the chromatin regulatory landscape that governs transcription in each cell type, has not been fully investigated. Here we propose EpiAnno, a probabilistic generative model integrated with a Bayesian neural network, to annotate scCAS data automatically in a supervised manner. We systematically validate the superior performance of EpiAnno for both intra- and inter-dataset annotation on various datasets. We further demonstrate the advantages of EpiAnno for interpretable embedding and biological implications via expression enrichment analysis, partitioned heritability analysis, enhancer identification, cis -coaccessibility analysis and pathway enrichment analysis. In addition, we show that EpiAnno has the potential to reveal cell type-specific motifs and facilitate scCAS data simulation.
更多查看译文
关键词
Classification and taxonomy,Computational models,Statistical methods,Engineering,general
AI 理解论文
溯源树
样例
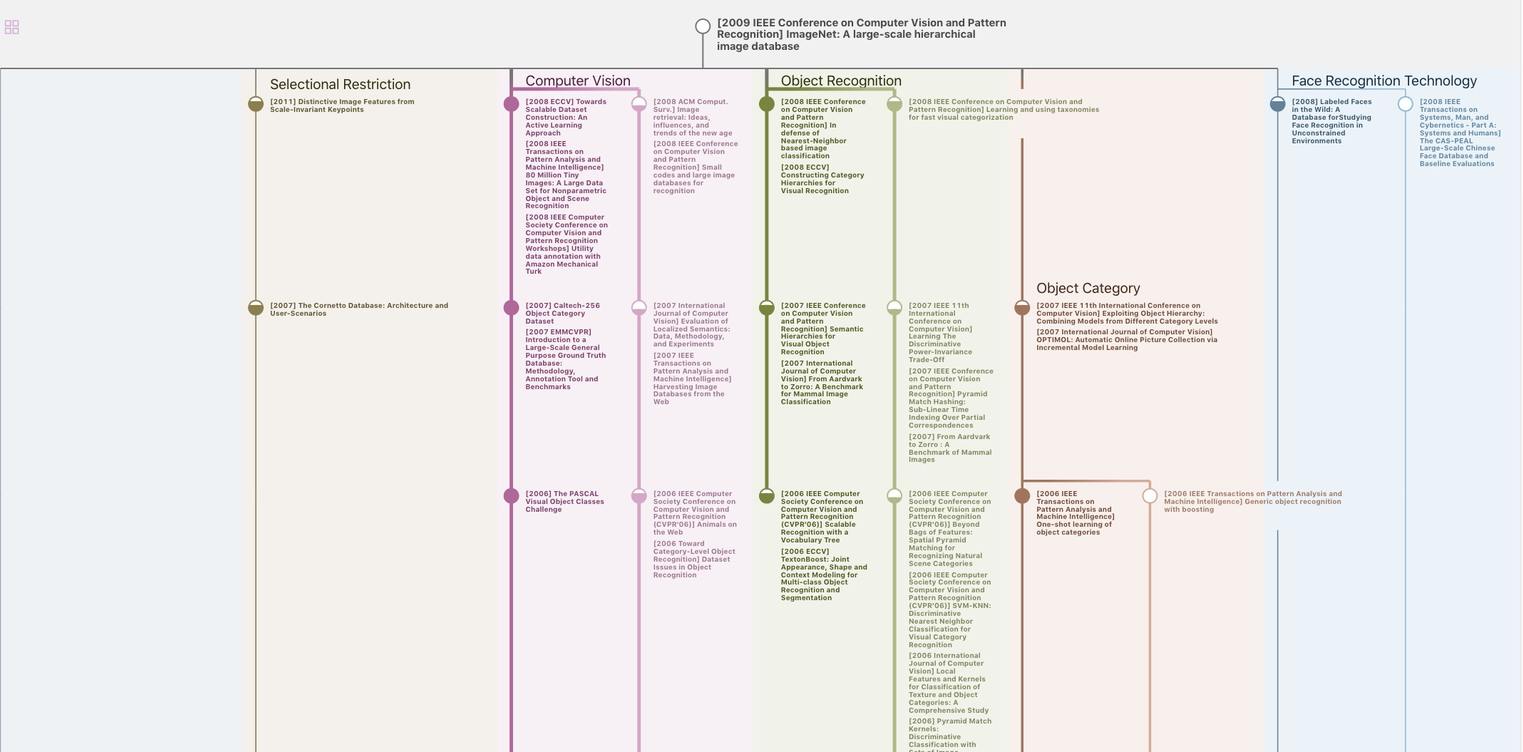
生成溯源树,研究论文发展脉络
Chat Paper
正在生成论文摘要