Response Generation by Jointly Modeling Personalized Linguistic Styles and Emotions
ACM TRANSACTIONS ON MULTIMEDIA COMPUTING COMMUNICATIONS AND APPLICATIONS(2022)
摘要
Natural language generation (NLG) has been an essential technique for various applications, like Xiaolce and Ski, and engaged increasing attention recently. To improve the user experience, several emotion-aware NLG methods have been developed to generate responses coherent with a pre-designated emotion (e.g., the positive or negative). Nevertheless, existing methods cannot generate personalized responses as they frequently overlook the personalized linguistic style. Apparently, different human responsers tend to have different linguistic styles. Inspired by this, in this work, we focus on a novel research theme of personalized emotion-aware NLG (PENLG), whereby the generated responses should be coherent with the linguistic style of a pre-designated responser and emotion. In particular, we study PENLG under a scenario of generating personalized emotion-aware response for social media post. Yet it faces certain research challenges: (1) the user linguistic styles are implicit and complex by nature, and hence it is hard to learn their representations; and (2) linguistic styles and emotions are usually expressed in different manners in a response, and thus how to convey them properly in the generated responses is not easy. Toward this end, we present a novel scheme of PENLG, named CRobot, which consists of a personalized emotion-aware response generator and two discriminators, i.e., general discriminator and personalized emotion-aware discriminator. To be more specific, the post-based and avatar-based user linguistic style modeling methods are incorporated into the encoder-decoder-based generator, while the discriminators are devised to ensure that the generated response is fluent and consistent with both the emotion and the linguistic style of the user. Different from the traditional adversarial networks, we embed adversarial learning under the umbrella of reinforcement learning. In this way, the response generation problem can be tackled by the generator taking a sequence of actions on selecting the proper word of each timestep for output. To justify our model, we construct a large-scale response generation dataset based on Twitter, consisting of 6,763 tweets with a corresponding 1,461,713 response created by 153,664 users. Extensive experiments demonstrate that CRobot surpasses the state-of-the-art baselines regarding both subjective and objective evaluation.
更多查看译文
关键词
Social media dataset construction,response generation,personalized and emotionalized response
AI 理解论文
溯源树
样例
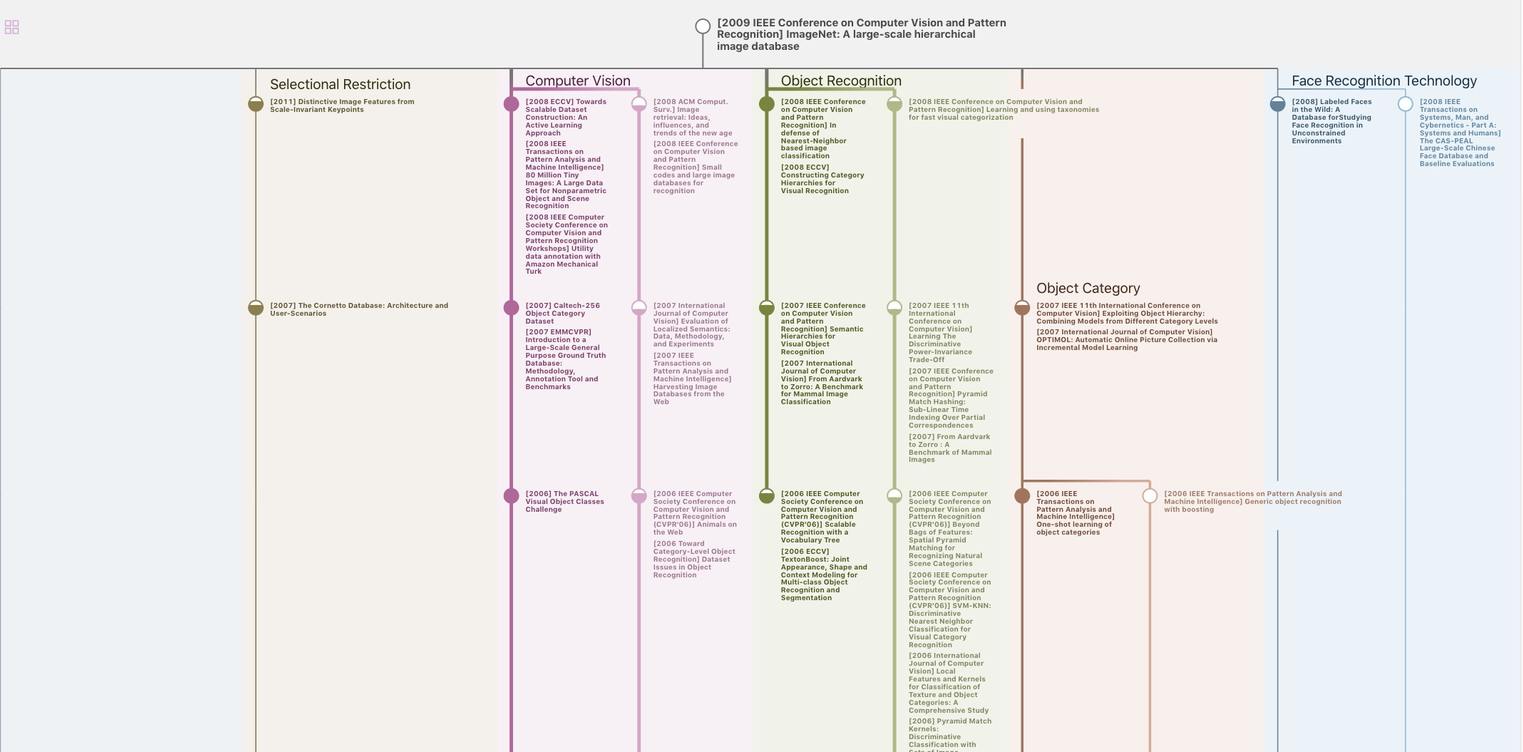
生成溯源树,研究论文发展脉络
Chat Paper
正在生成论文摘要