Concrete Crack Quantification Using Voxel-Based Reconstruction and Bayesian Data Fusion
IEEE Transactions on Industrial Informatics(2022)
摘要
Concrete cracks are one of the most apparent indicators for possible structural deterioration and need to be periodically inspected. However, for current image-based automated crack inspection techniques, accurate and detailed crack quantification and assessment remain a challenging task. Most of these techniques require high-quality input images, which may be difficult to ensure in practice. Besides, simply merging crack detections from multiple images to generate a large crack map may result in an inaccurate outcome for crack severity assessment. In this article, a novel crack quantification framework is proposed to identify complete crack geometric properties utilizing a set of unordered inspection images. To realize this, cracks in images are detected by an instance segmentation convolutional neural network. Subsequently, the crack segmentations from multiple separate images are systematically aggregated through voxel-based reconstruction and Bayesian data fusion. This framework outputs a crack model that can retrieve accurate geometric properties of each crack segment by recognizing the crack's inherent branching patterns. The capability and performance of the proposed crack quantification framework are validated on cracked concrete specimens in a laboratory setting. Also, a field test on a cracked concrete wall was carried out using images captured by a UAV to demonstrate the efficacy of the proposed framework in practical conditions.
更多查看译文
关键词
Bayesian data fusion,crack quantification,instance segmentation network,voxel-based reconstruction
AI 理解论文
溯源树
样例
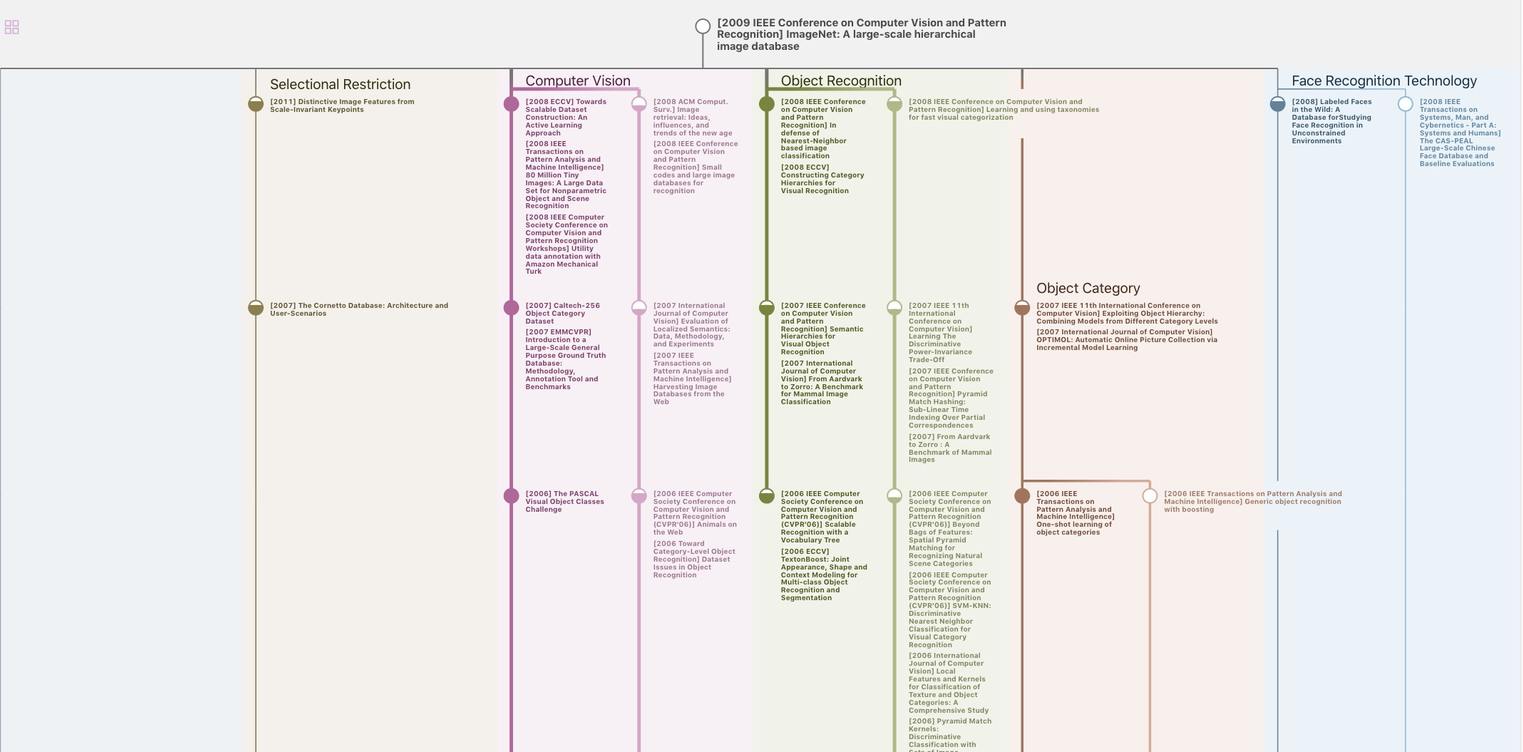
生成溯源树,研究论文发展脉络
Chat Paper
正在生成论文摘要