Learning with limited supervision
Advanced Methods and Deep Learning in Computer Vision(2022)
摘要
The recent successes in computer vision, and machine learning in general, have been mostly around using a huge corpus of intricately labeled data for training models. But, in real-world cases, acquiring such large datasets will require a lot of manual annotation, which may be strenuous, out of budget, or even prone to errors. Thus, it is necessary to develop methods where the amount of labeled data is minimized or can be obtained at low to no annotation cost. This chapter is a brief summary of methods that can be used to learn recognition models from such data which involve limited manual supervision. We will look into two dimensions of learning with limited supervision – first, reducing the number of manually labeled data required to learn recognition models, and second, reducing the level of supervision from strong to weak which can be mined from the web, easily queried from an oracle, or imposed as rule-based labels derived from domain knowledge. We will provide an overview of the theoretical frameworks and some experimental results.
更多查看译文
关键词
supervision,learning,limited
AI 理解论文
溯源树
样例
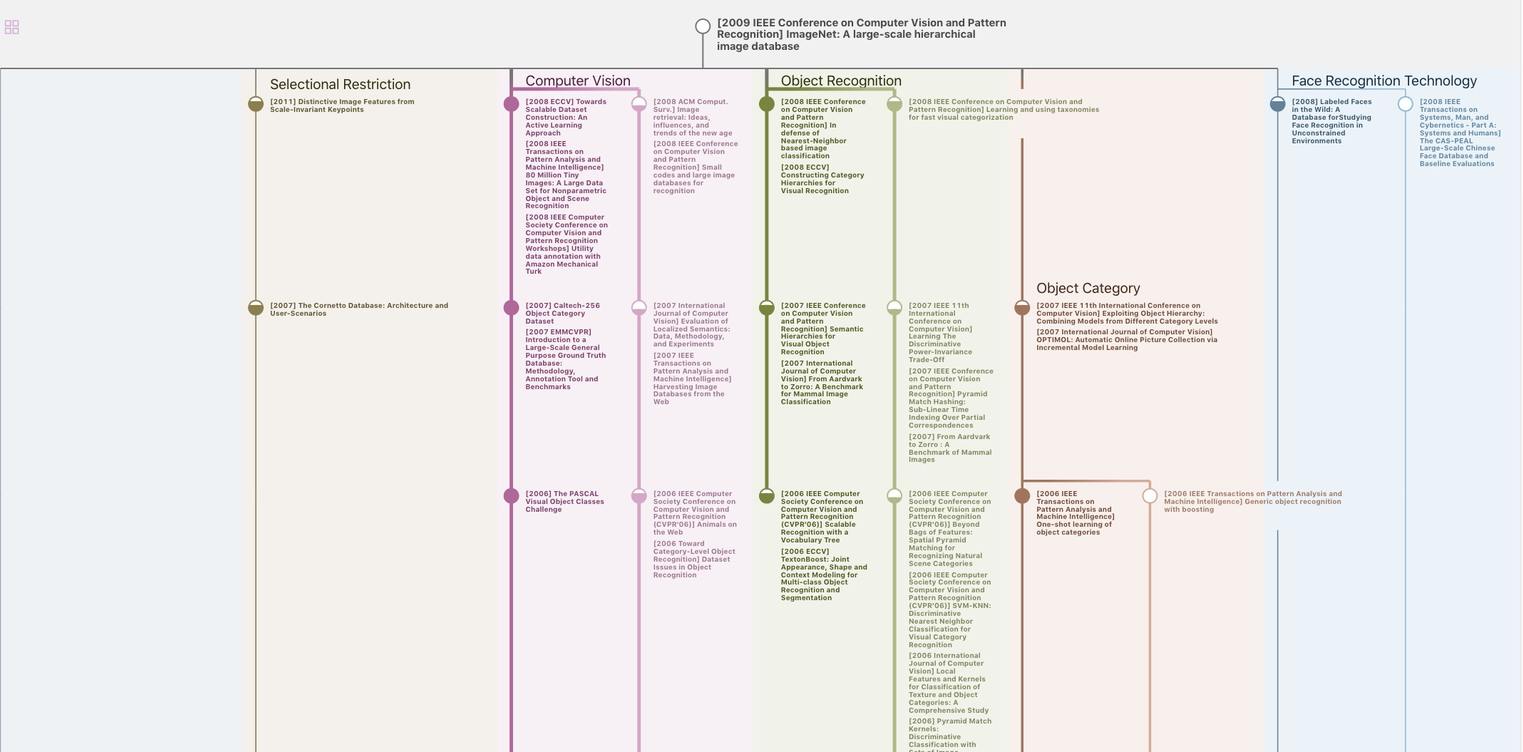
生成溯源树,研究论文发展脉络
Chat Paper
正在生成论文摘要