Learning point cloud shapes with geometric and topological structures
Communications in Information and Systems(2022)
摘要
3D point cloud semantic analysis is challenging due to irregular locations and ill-posed sparse representations. In this study, we explore the intrinsic structures of point clouds, which assist convolutional neural networks in classification and segmentation tasks. The network is referred to as a geometric and topological structures based convolutional neural network (GTS-CNN). Firstly, the method extracts meaningful geometric adjacency for each surface point as well as the topological persistence information for the whole point cloud. Then the GTS-CNN processes this information with a multi-head mechanism. There are three branches within the network executing graph neighborhood message passing, point position-related inference, and persistence image feature embedding, respectively. In this way, an expressive descriptor is obtained with a combination of three kinds of features, leading to a robust and finely grained representation. Experiments on standard benchmarks, such as ModelNet40 and ShapeNet, show that our network achieves promising performance compared to state-of-the-art methods.
更多查看译文
AI 理解论文
溯源树
样例
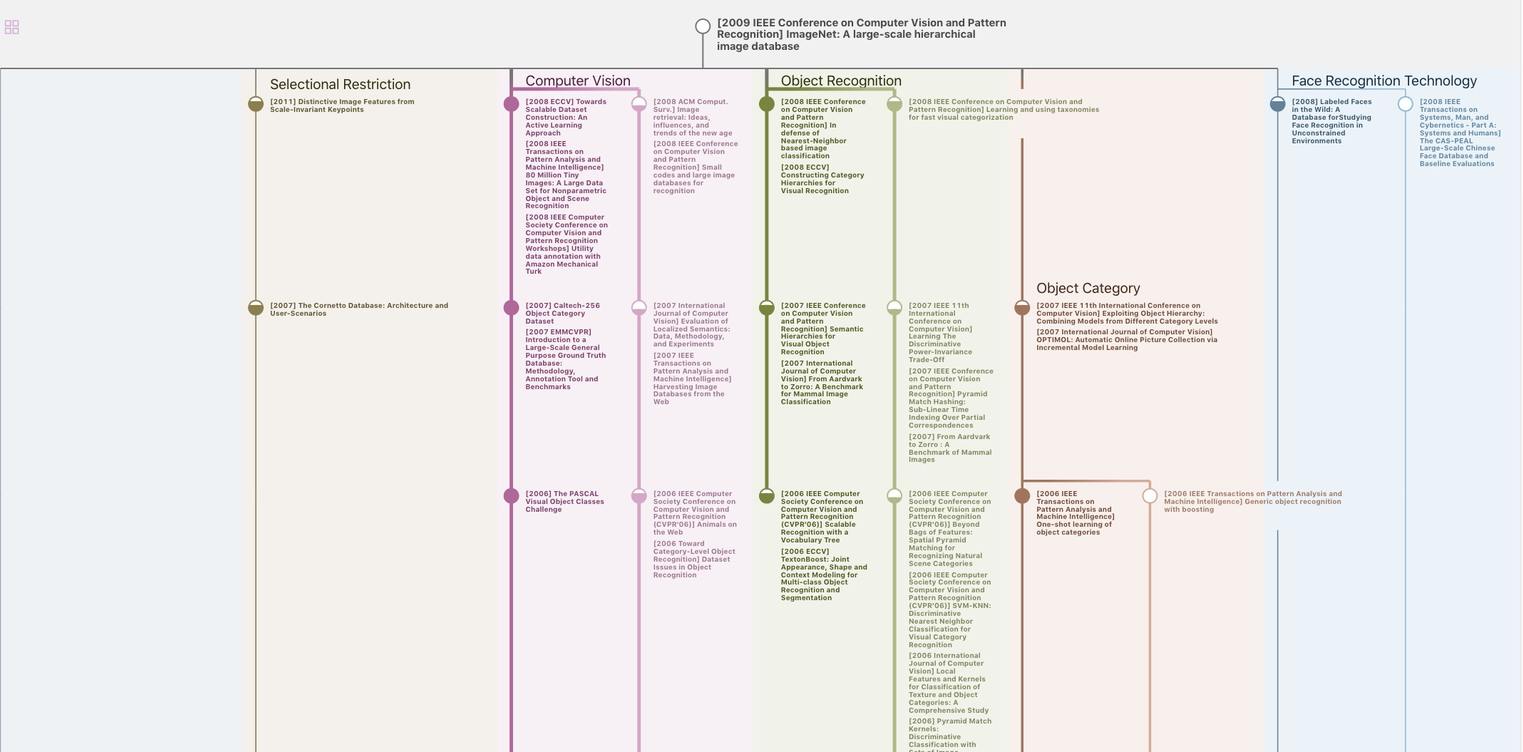
生成溯源树,研究论文发展脉络
Chat Paper
正在生成论文摘要