Clustering and Analysis of Gps Trajectory Data Using Distance-Based Features
SSRN Electronic Journal(2022)
摘要
The proliferation of smartphones has accelerated mobility studies by largely increasing the type and volume of mobility data available. One such source of mobility data is from GPS technology, which is becoming increasingly common and helps the research community understand mobility patterns of people. However, there lacks a standardized framework for studying the different mobility patterns created by the non-Work, non-Home locations of Working and Nonworking users on Workdays and Offdays using machine learning methods. We propose a new mobility metric, Daily Characteristic Distance, and use it to generate features for each user together with Origin-Destination matrix features. We then use those features with an unsupervised machine learning method, $k$-means clustering, and obtain three clusters of users for each type of day (Workday and Offday). Finally, we propose two new metrics for the analysis of the clustering results, namely User Commonality and Average Frequency. By using the proposed metrics, interesting user behaviors can be discerned and it helps us to better understand the mobility patterns of the users.
更多查看译文
关键词
Urban mobility,insight extraction,daily characteristic distance,GPS trajectories
AI 理解论文
溯源树
样例
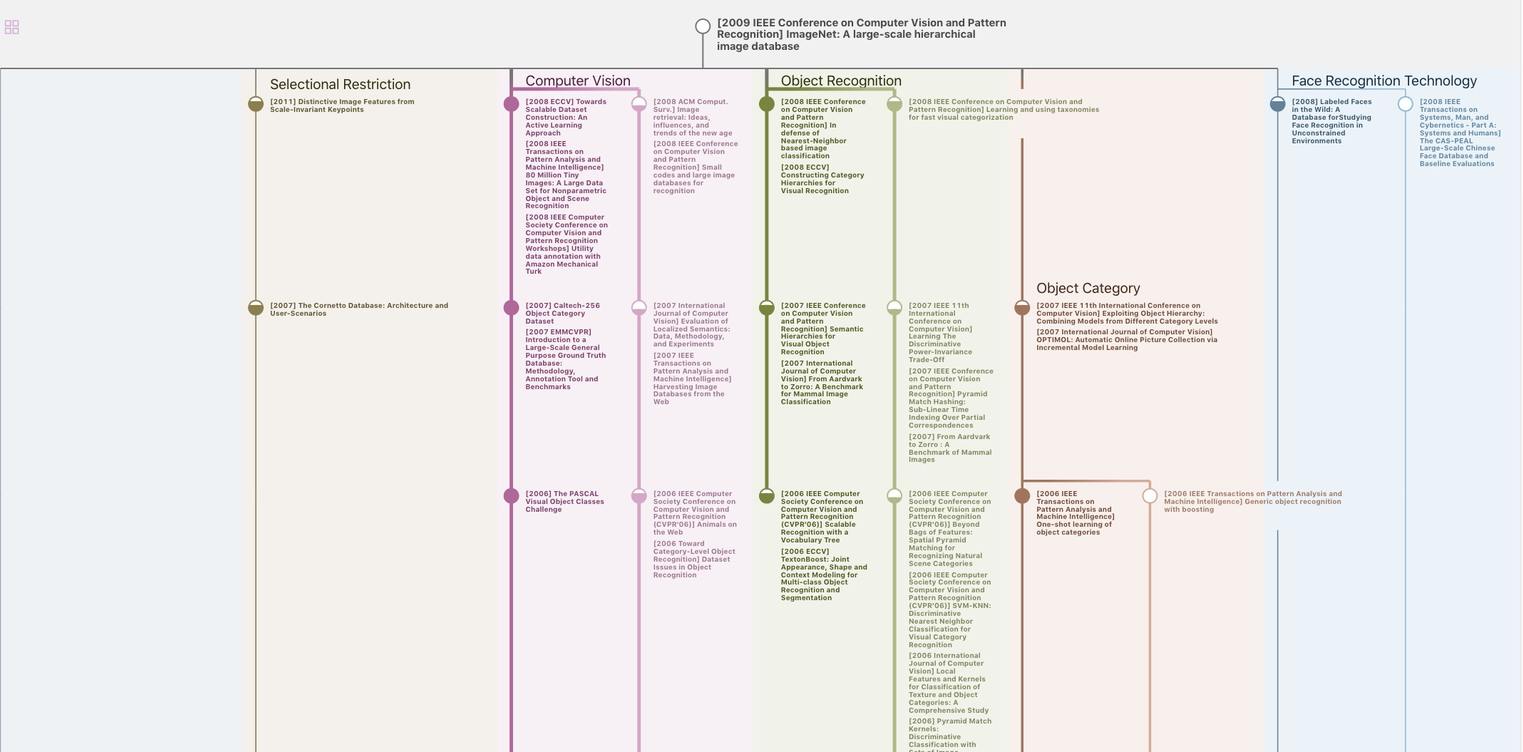
生成溯源树,研究论文发展脉络
Chat Paper
正在生成论文摘要