DIAG: A Deep Interaction-Attribute-Generation model for user-generated item recommendation
Knowledge-Based Systems(2022)
摘要
Most existing recommendation methods assume that all the items are provided by separate producers rather than users. However, it could be inappropriate in some recommendation tasks since users may generate some items. Considering the user–item generation relation may benefit recommender systems that only use implicit user–item interactions. However, it may suffer from a dramatic imbalance. The number of user–item generation relations may be far smaller than the number of user–item interactions because each item is generated by at most one user. At the same time, this item can be interacted with by many users. To overcome the challenging imbalance issue, we propose a novel Deep Interaction-Attribute-Generation (DIAG) model. It integrates the user–item interaction relation, the user–item generation relation, and the item attribute information into one deep learning framework. The novelty lies in the design of a new item–item co-generation network for modeling the user–item generation information. Then, graph attention network is adopted to learn the item feature vectors from the user–item generations and the item attribute information by considering the adaptive impact of one item on its co-generated items. Extensive experiments conducted on two real-world datasets confirm the superiority of the DIAG method.
更多查看译文
关键词
Recommendation,User-generated item,User-item interaction,User-item generation,Item attribute,Item-item co-generation network,Deep learning
AI 理解论文
溯源树
样例
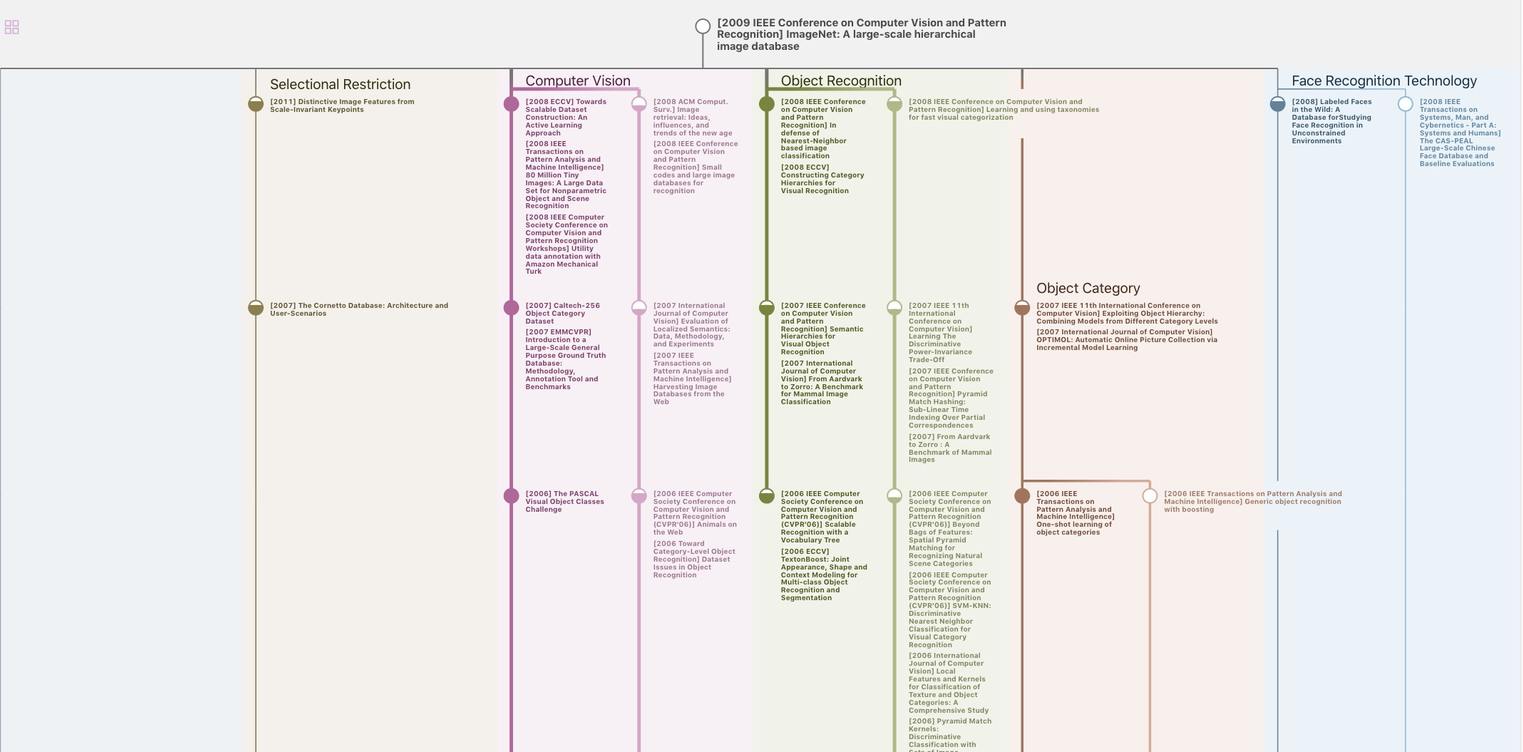
生成溯源树,研究论文发展脉络
Chat Paper
正在生成论文摘要