Rainy season precipitation forecasts in coastal Peru from the North American Multi-Model Ensemble
International Journal of Climatology(2022)
摘要
We assessed operational forecasts of rainy season (January-March [JFM]) precipitation over coastal Peru from seven global climate models (GCMs) in the North American Multi-Model Ensemble (NMME) from 1982 to 2020. Similar to previous representations by GCMs, regional climate models, and atmospheric reanalyses, the NMME models produce excessive precipitation (more than twice the observed values) over all of coastal Peru. Based on a set of commonly used metrics (correlation, mean error, RMSE, and Heidke skill score), there is a wide range in accuracy among the NMME models. As expected, forecast accuracy degrades with increasing lead time from December to July, but the rate of decline varies greatly by region and among models. The cause(s) of differences in model performance remains unclear, but resolution does not appear to be a significant factor. Highest forecast skill is found for southwestern Peru (correlations with observations are significant at all lead times), probably because of its stronger relationship between rainfall and El Nino-Southern Oscillation (ENSO), and lowest skill occurs for west-central Peru (insignificant correlations at all lead times). The distribution of interannual rainy season precipitation in northwestern Peru is extremely positively skewed (skewness >2), making evaluation of forecasts difficult from only a few years that deviate strongly from the mean. Improved correlations with observations across regions are generally found by subsetting multi-model mean forecasts using the better-performing models. Over northwest Peru at short lead times, NMME rainy season precipitation forecasts outperform a simple statistical correlation between JFM rainfall and ENSO, but not at longer leads, while NMME's forecasts for west-central Peru underperform statistical correlations at all lead times. Over southwest Peru, the NMME models are consistently more skilful than a statistical correlation, and these differences become larger with increasing lead time.
更多查看译文
关键词
bias, coastal, forecast, model, NMME, Peru, precipitation, season
AI 理解论文
溯源树
样例
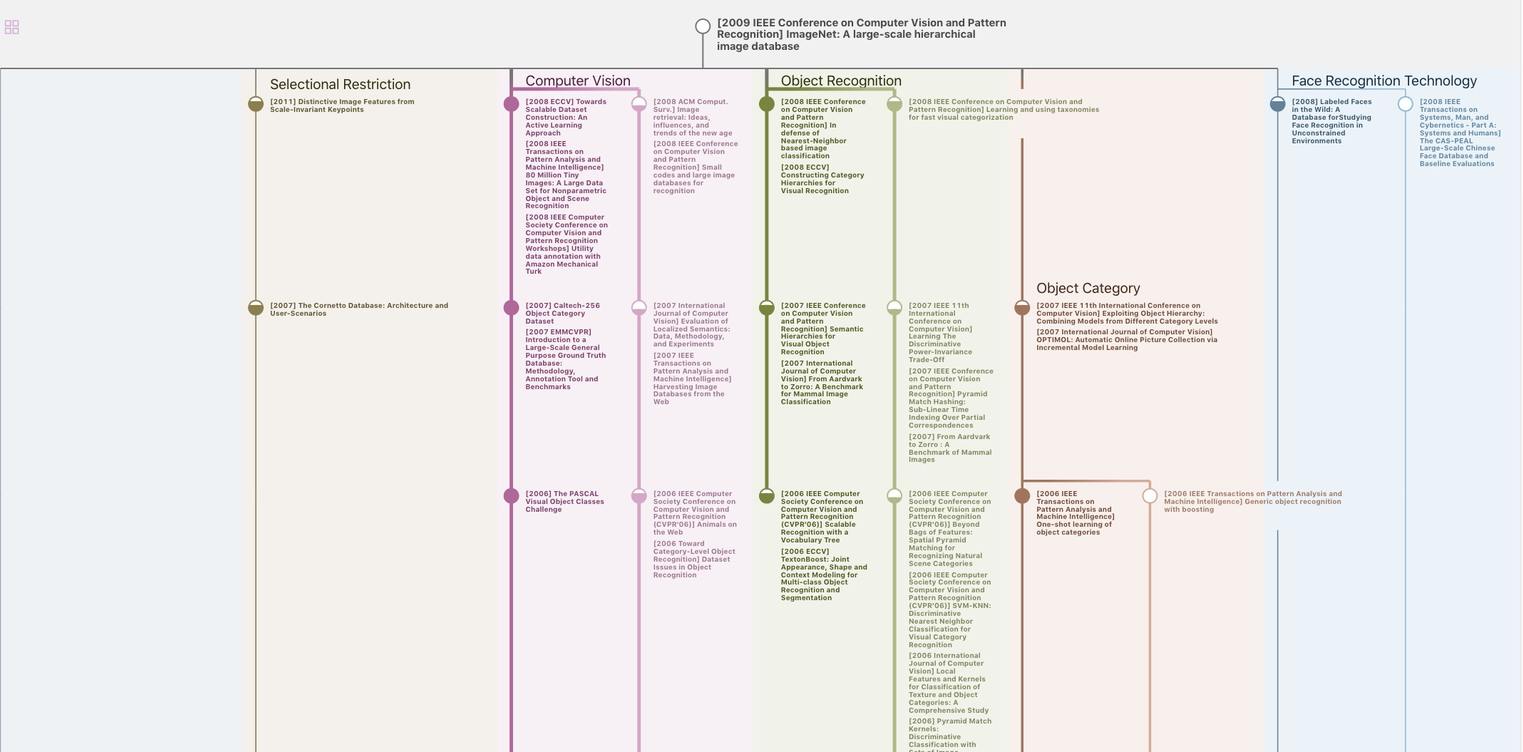
生成溯源树,研究论文发展脉络
Chat Paper
正在生成论文摘要