Cross-Lingual Transfer Learning in Drug-Related Information Extraction from User-Generated Texts
Proceedings of the Institute for System Programming of the RAS(2023)
摘要
Aggregating knowledge about drug, disease, and drug reaction entities across a broader range of domains and languages is critical for information extraction applications. In this work, we present a fine-grained evaluation intended to understand the efficiency of multilingual BERT-based models for biomedical named entity recognition (NER) and multi-label sentence classification. We investigate the role of transfer learning strategies between two English corpora and a novel annotated corpus of Russian reviews about drug therapy. In these corpora, labels for sentences indicate health-related issues or their absence. Sentences that belong to a certain class are additionally labeled at the entity level to identify fine-grained subtypes such as drug names, drug indications, and drug reactions. The evaluation results demonstrate that the BERT training on Russian and English raw reviews (5M in total) provides the best transfer capabilities for adverse drug reactions detection task on the Russian data. The macro F1 score of 74.85% in the NER task was achieved by our RuDR-BERT model. For the classification task, our EnRuDR-BERT model achieved the macro F1 score of 70%, gaining 8.64% over the score of a general-domain BERT model.
更多查看译文
关键词
natural language processing,text classification,information extraction,named entity recognition,BERT
AI 理解论文
溯源树
样例
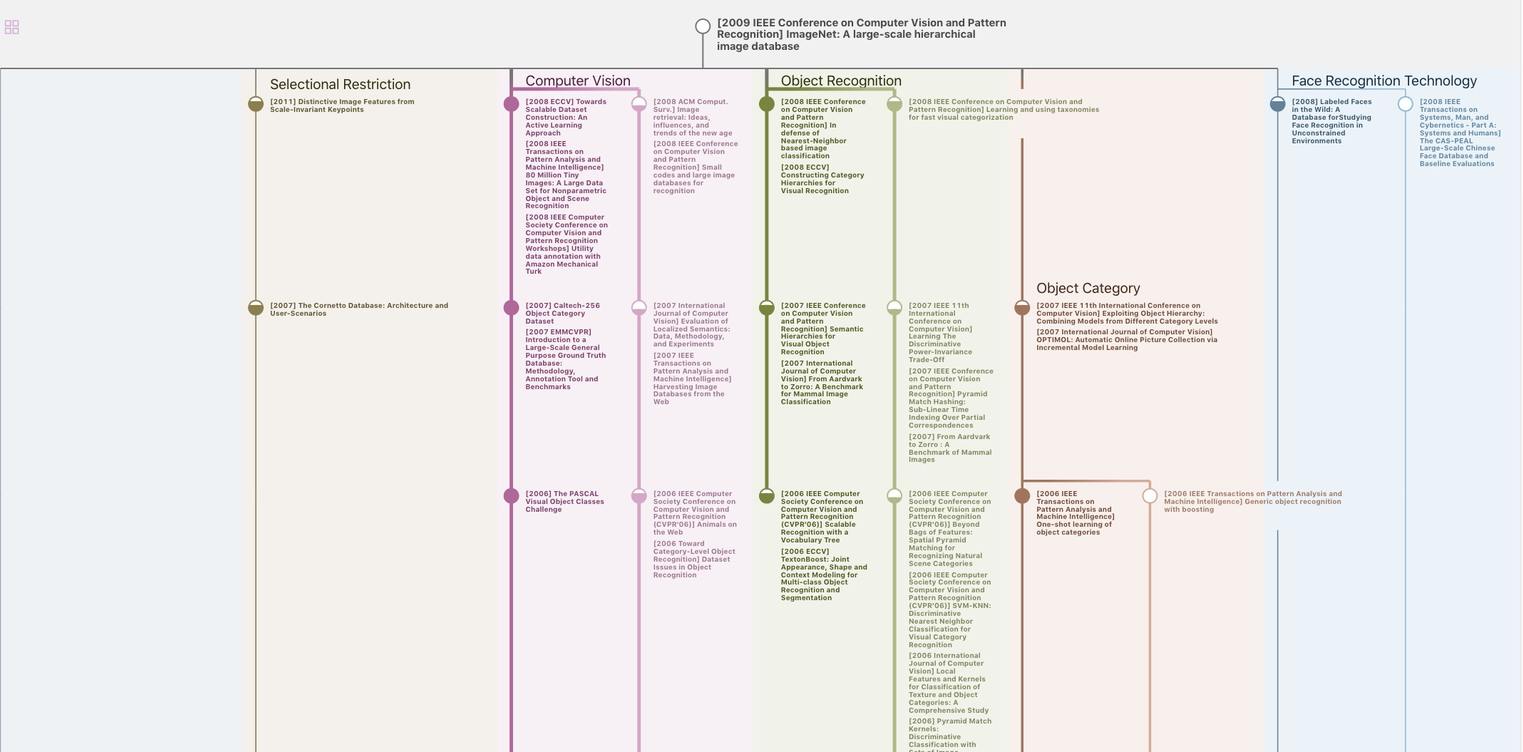
生成溯源树,研究论文发展脉络
Chat Paper
正在生成论文摘要