An Evolutionary Algorithm With Constraint Relaxation Strategy for Highly Constrained Multiobjective Optimization.
IEEE transactions on cybernetics(2023)
摘要
Highly constrained multiobjective optimization problems (HCMOPs) refer to constrained multiobjective optimization problems (CMOPs) with complex constraints and small feasible regions, which are commonly encountered in many real-world applications. Current constraint-handling techniques will face two difficulties when dealing with HCMOPs: 1) feasible solution is hard to be found and too much search effort is spent in locating the feasible region and 2) since the total feasible region of an HCMOP can consist of several disconnected subregions, the search process might be stuck in the comparatively larger feasible subregion, which does not contain the whole Pareto front (PF). To address these two issues, an evolutionary algorithm with constraint relaxation strategy based on differential evolution algorithm, that is, CRS-DE, is proposed in this article. In each generation, the CRS-DE relaxes the constraints by dividing the infeasible solutions into two subpopulations based on total constraint violation, that is, the "semifeasible" subpopulation (SF) and "infeasible" subpopulation (IF), respectively. The SF provides information on the promising regions of finding the feasible solution and is the driving force for convergence toward the PF, while the IF focuses on global exploration for new promising regions. Corresponding reproduction and selection strategies are devised for the SF, IF, and feasible subpopulations, which create a clear division of labor with cooperation to facilitate the search for feasible solutions. To leverage the influence of CRS and prevent the population from premature convergence, a mobility restriction mechanism is developed to restrict the individuals in the SF and IF from entering the feasible subpopulation and enhance the diversity of the whole population. Comprehensive experiments on a series of benchmark test problems and a real-world CMOP demonstrate the competitiveness of our method compared with other representative algorithms in terms of effectiveness and reliability in finding a set of well-distributed optimal solutions for HCMOPs.
更多查看译文
关键词
Constraint-handling techniques,differential evolution,evolutionary algorithms,multiobjective optimization problems (MOPs)
AI 理解论文
溯源树
样例
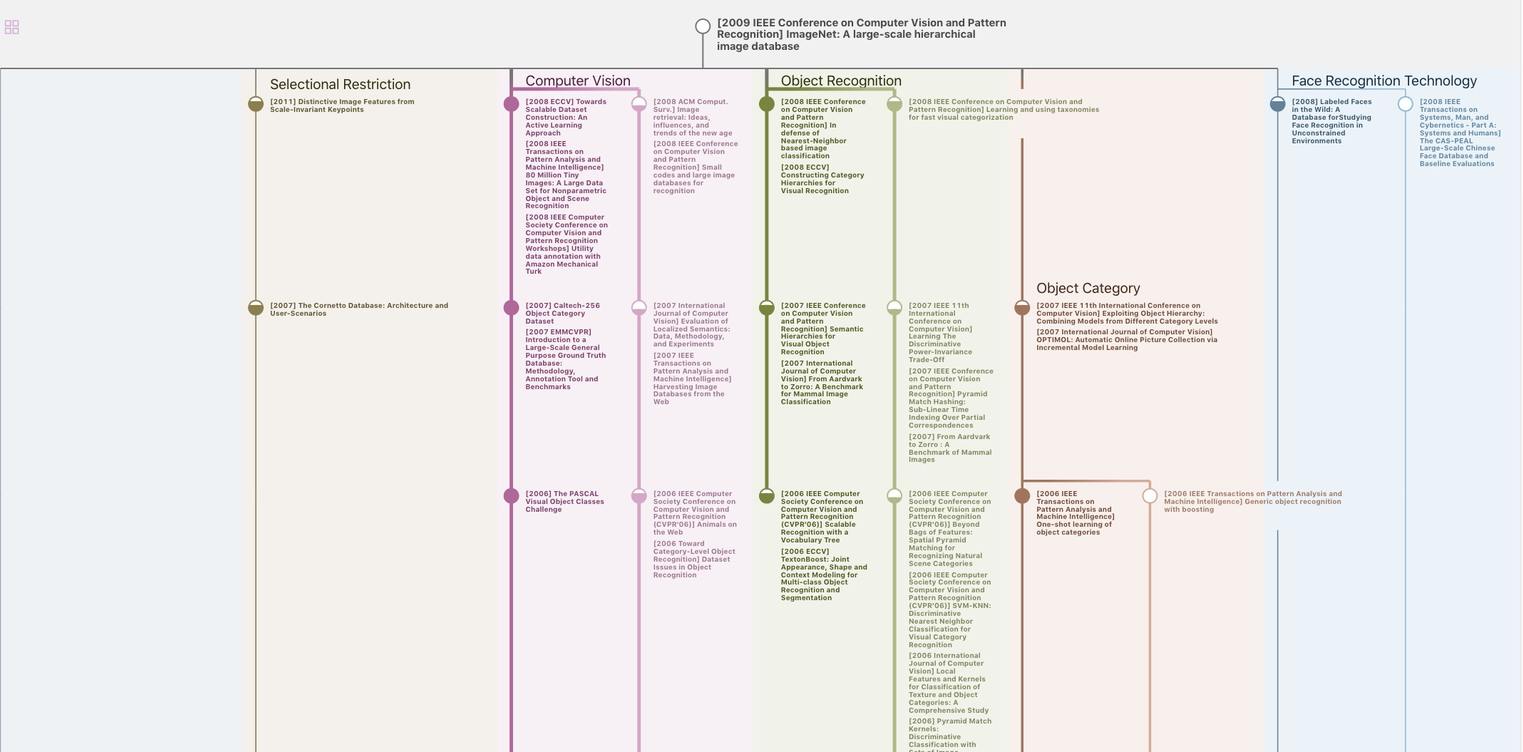
生成溯源树,研究论文发展脉络
Chat Paper
正在生成论文摘要