Central Attention Network for Hyperspectral Imagery Classification
IEEE transactions on neural networks and learning systems(2023)
摘要
In this article, the intrinsic properties of hyperspectral imagery (HSI) are analyzed, and two principles for spectral–spatial feature extraction of HSI are built, including the foundation of pixel-level HSI classification and the definition of spatial information. Based on the two principles, scaled dot-product central attention (SDPCA) tailored for HSI is designed to extract spectral–spatial information from a central pixel (i.e., a query pixel to be classified) and pixels that are similar to the central pixel on an HSI patch. Then, employed with the HSI-tailored SDPCA module, a central attention network (CAN) is proposed by combining HSI-tailored dense connections of the features of the hidden layers and the spectral information of the query pixel. MiniCAN as a simplified version of CAN is also investigated. Superior classification performance of CAN and miniCAN on three datasets of different scenarios demonstrates their effectiveness and benefits compared with state-of-the-art methods.
更多查看译文
关键词
Feature extraction,Data mining,Convolutional neural networks,Atomic measurements,Hyperspectral imaging,Transformers,Spectral analysis,Central attention,hyperspectral imagery (HSI),spectral-spatial feature extraction,transformer
AI 理解论文
溯源树
样例
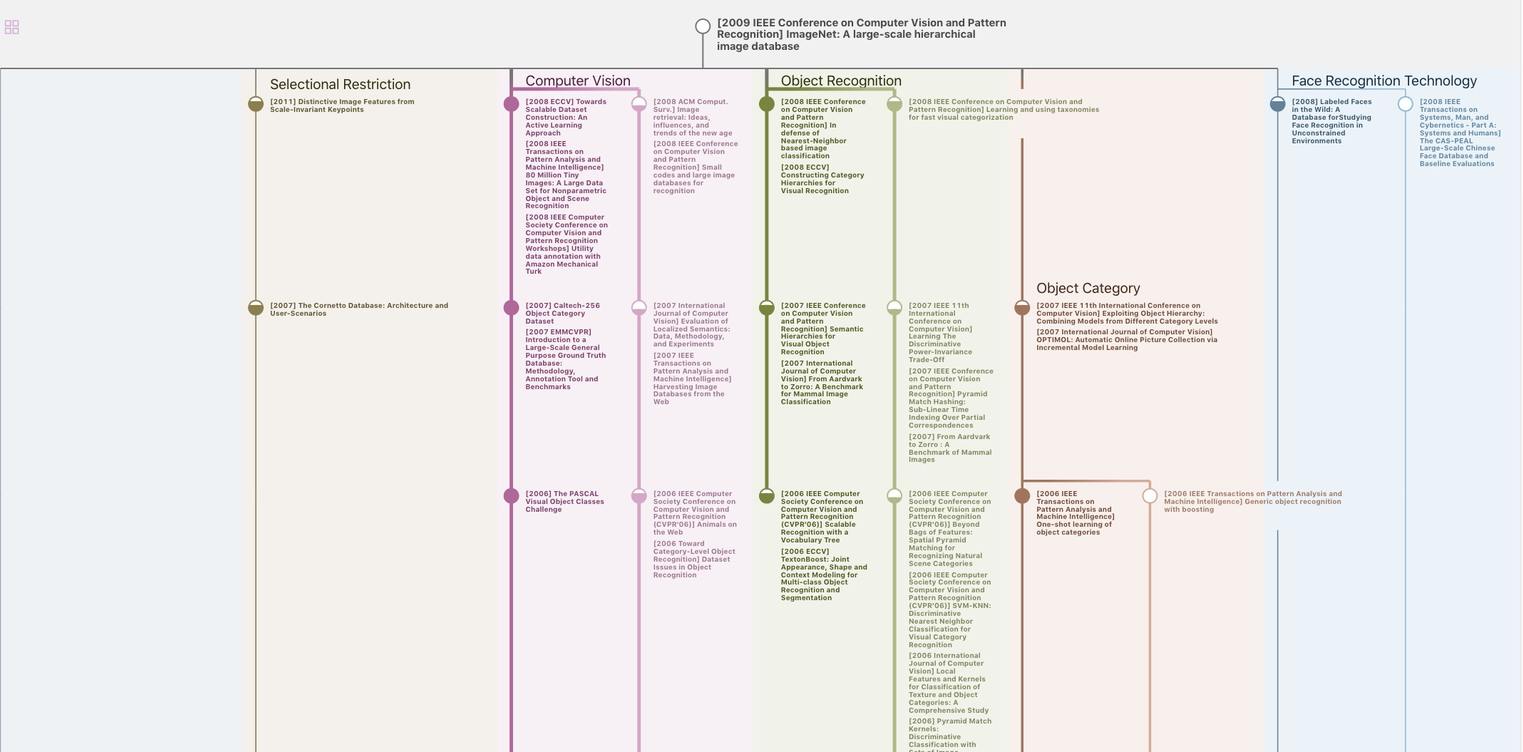
生成溯源树,研究论文发展脉络
Chat Paper
正在生成论文摘要