Prediction of Neonatal Respiratory Distress Biomarker Concentration by Application of Machine Learning to Mid-Infrared Spectra
SENSORS(2022)
摘要
The authors of this study developed the use of attenuated total reflectance Fourier transform infrared spectroscopy (ATR-FTIR) combined with machine learning as a point-of-care (POC) diagnostic platform, considering neonatal respiratory distress syndrome (nRDS), for which no POC currently exists, as an example. nRDS can be diagnosed by a ratio of less than 2.2 of two nRDS biomarkers, lecithin and sphingomyelin (L/S ratio), and in this study, ATR-FTIR spectra were recorded from L/S ratios of between 1.0 and 3.4, which were generated using purified reagents. The calibration of principal component (PCR) and partial least squares (PLSR) regression models was performed using 155 raw baselined and second derivative spectra prior to predicting the concentration of a further 104 spectra. A three-factor PLSR model of second derivative spectra best predicted L/S ratios across the full range (R-2: 0.967; MSE: 0.014). The L/S ratios from 1.0 to 3.4 were predicted with a prediction interval of +0.29, -0.37 when using a second derivative spectra PLSR model and had a mean prediction interval of +0.26, -0.34 around the L/S 2.2 region. These results support the validity of combining ATR-FTIR with machine learning to develop a point-of-care device for detecting and quantifying any biomarker with an interpretable mid-infrared spectrum.
更多查看译文
关键词
ATR-FTIR, spectroscopy, machine learning, neonatal respiratory distress syndrome, point-of-care devices
AI 理解论文
溯源树
样例
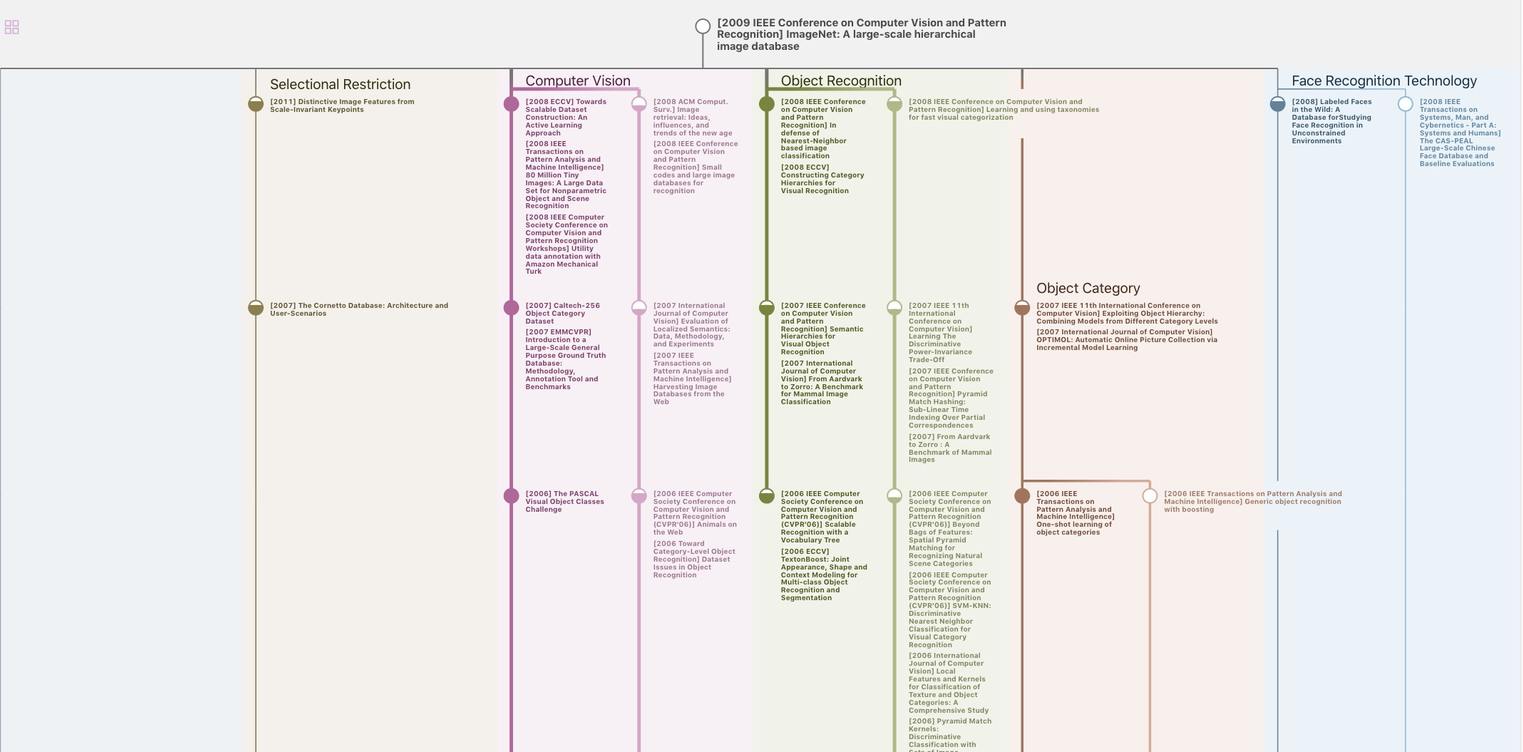
生成溯源树,研究论文发展脉络
Chat Paper
正在生成论文摘要