Conditional Synthetic Data Generation for Personal Thermal Comfort Models
arxiv(2022)
摘要
Personal thermal comfort models aim to predict an individual's thermal comfort response, instead of the average response of a large group. Recently, machine learning algorithms have proven to be having enormous potential as a candidate for personal thermal comfort models. But, often within the normal settings of a building, personal thermal comfort data obtained via experiments are heavily class-imbalanced. There are a disproportionately high number of data samples for the "Prefer No Change" class, as compared with the "Prefer Warmer" and "Prefer Cooler" classes. Machine learning algorithms trained on such class-imbalanced data perform sub-optimally when deployed in the real world. To develop robust machine learning-based applications using the above class-imbalanced data, as well as for privacy-preserving data sharing, we propose to implement a state-of-the-art conditional synthetic data generator to generate synthetic data corresponding to the low-frequency classes. Via experiments, we show that the synthetic data generated has a distribution that mimics the real data distribution. The proposed method can be extended for use by other smart building datasets/use-cases.
更多查看译文
关键词
personal thermal comfort
AI 理解论文
溯源树
样例
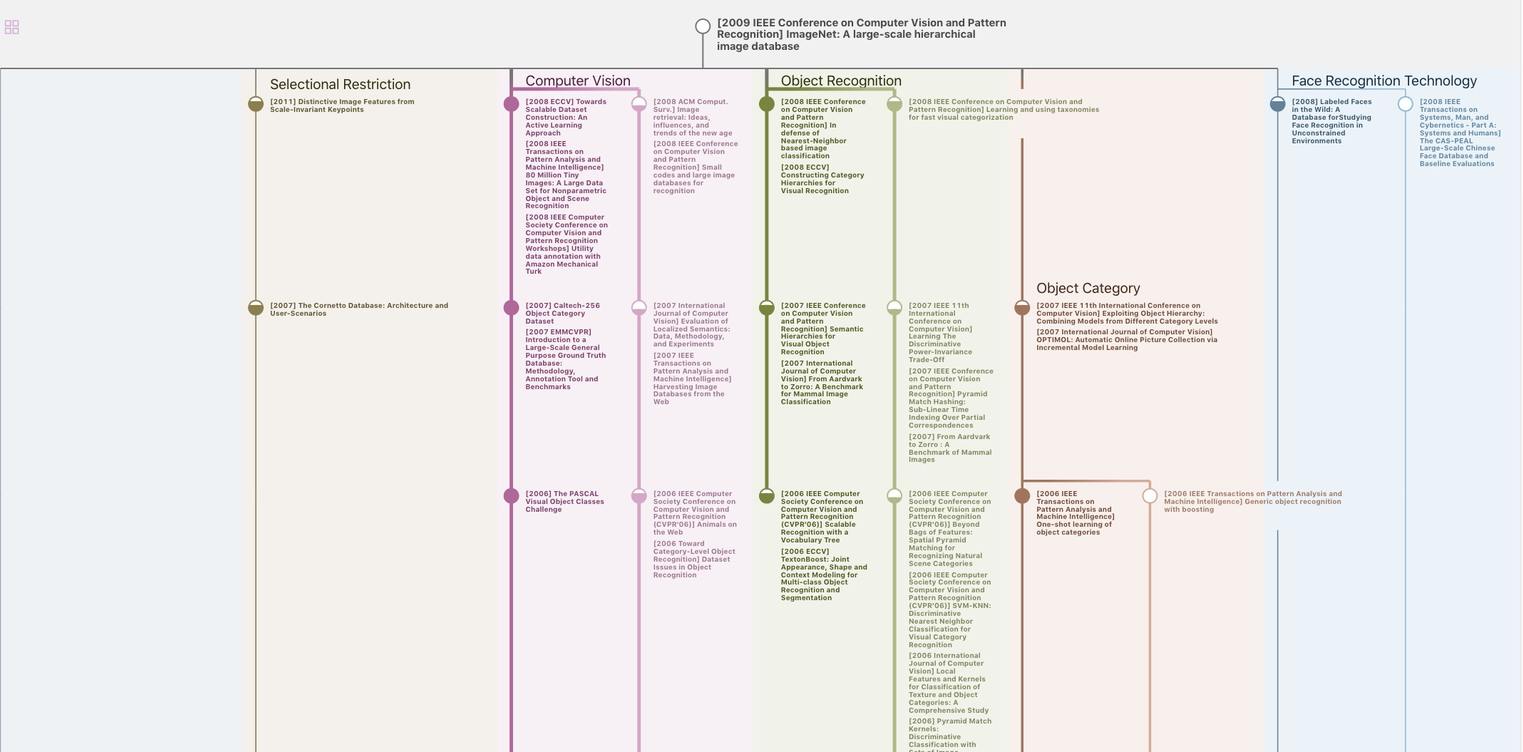
生成溯源树,研究论文发展脉络
Chat Paper
正在生成论文摘要