CT Metal Artifact Correction Assisted by the Deep Learning-based Metal Segmentation on the Projection Domain
2021 IEEE International Conference on Medical Imaging Physics and Engineering (ICMIPE)(2021)
摘要
Background and objective: Metal artifact seriously effects the quality of the image and reduce the accurate of the diagnosis. To mitigate the effect of the metal artifacts and improve the image quality of computed tomography (CT), we propose a CT metal artifact correction method assisted by the deep learning-based metal segmentation on the projection domain. Method: The metal artifact is firstly segmented in the projection image via an attention U-net (AU-Net). We can obtain the metal-only projection image with the accurate metal segmentation. With the image interpolation, the metal region can be removed in the projection image and produce a metal-free projection image. The metal-only and the metal-free reconstructed images are derived from the corresponding projection images using the iterative and filtered back-projection (FBP) reconstruction method, respectively. The final corrected image is combined by the metal-only and the metal-free reconstructed images. The proposed framework was evaluated by the pig's feet with the implanted metal. Results: Compared with the traditional methods, the spatial non-uniformity (SNU) of the corrected image is reduced from 61 HU to 21 HU. The proposed method based on deep learning was more efficient and accurate. Besides, the proposed method can not only alleviate metal artifacts, but also preserve the metal information well. Conclusions: In this study, we incorporate an automatic metal segmentation based deep learning into the metal artifact correction method. The results demonstrated that the proposed metal artifact correction framework is efficient and accurate. In the future, more clinical patient data will be included in the evaluation.
更多查看译文
关键词
Metal artifact,convolutional neural network,deep learning,computed tomography,segmentation
AI 理解论文
溯源树
样例
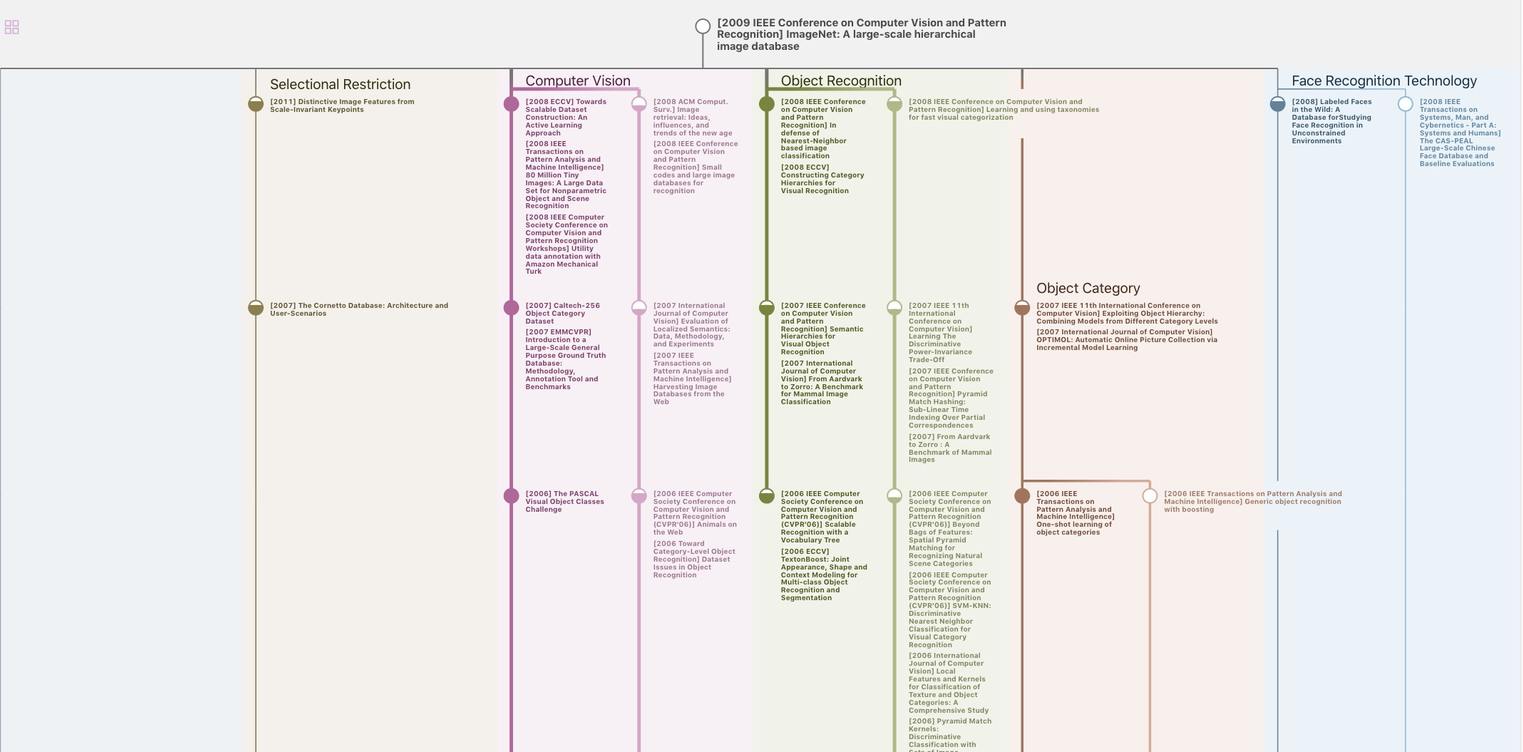
生成溯源树,研究论文发展脉络
Chat Paper
正在生成论文摘要