Open-Set Recognition for Deep Neural Networks-based Underwater Acoustic Target Classification
OCEANS 2021: San Diego – Porto(2021)
摘要
Underwater acoustic target classification is a popular research topic in sonar signal processing. With the development of deep learning in recent years, deep neural networks-based approaches have received increasingly attention in this field. But conventional deep learning methods are powerless against the occurrence of unknown classes during the testing, which is a very common situation in practice. The scenario with the incomplete target categories in the training set is considered in this article, and a reconstruction-based approach is proposed to deal with it. The proposed approach introduces an additional decoder to calculate the reconstruction error, and a detector to process the error and give the confidence of the current classification made by the network. By rejecting low-confidence classifications and including them into the uncertainty set, the approach achieves better precision and F1 score than the selected baseline under different signal-to-noise ratio. The gains are verified experimentally on a ship noise dataset, and those in F1 score are about 0.07 and 0.10 at 5 dB and 10 dB, respectively.
更多查看译文
关键词
underwater acoustic target classification,open-set recognition,deep neural networks,reconstruction,Weibull distribution
AI 理解论文
溯源树
样例
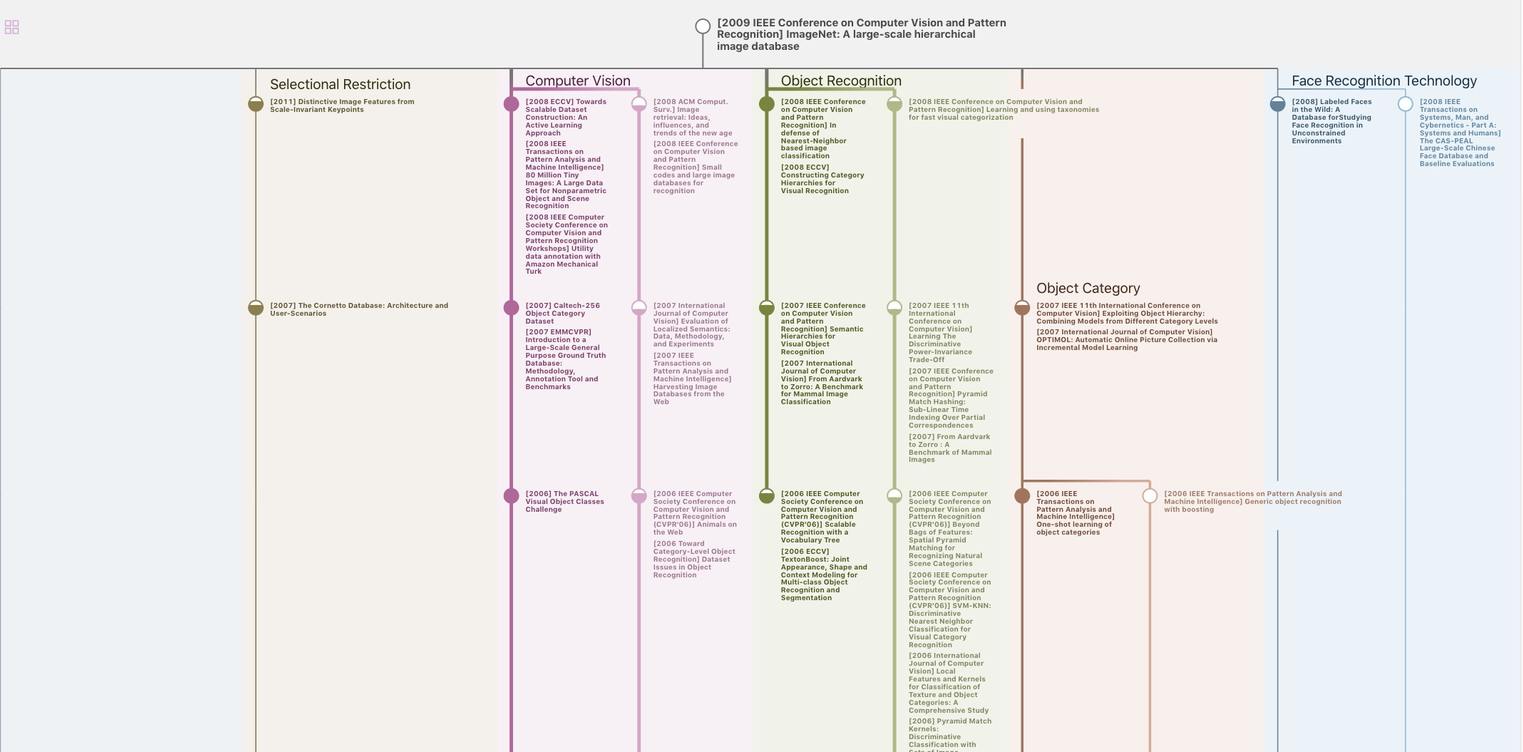
生成溯源树,研究论文发展脉络
Chat Paper
正在生成论文摘要