Ultrafast Learning-Based Nonlinear Model Predictive Control and its Embedded Realization
2021 Seventh Indian Control Conference (ICC)(2021)
摘要
The core idea of nonlinear model predictive control (NMPC) is to solve an online optimization problem at each sample instant, considering the initial conditions and constraints into account. The online optimization process requires huge computations and is therefore hard to realize on resource-limited hardware. In this paper, a versatile, data-driven, deep learning-based NMPC is proposed for the embedded control applications, which eliminates the burden of solving online optimization problems. The learned controller is intended to reduce the numerical intricacy involved in classical NMPC while keeping its advantages intact. Our idea is to develop a deep neural network (DNN) NMPC based on the data generated by solving an open-loop optimization problem. Then the learned-based NMPC is implemented on resource-limited embedded hardware (Raspberry Pi v4), and its performance is analyzed on a continuous stirred tank reactor (CSTR) system. Furthermore, the performance of developed DNN-NMPC is compared with the classical NMPC implemented on Raspberry Pi v4. The hardware-in-the-line co-simulation results show that the DNN-NMPC imitates the behavior of NMPC while reducing the time complexity. Thus, eliminating the main bottleneck of classical NMPC, this paper elucidates an alternative algorithm to increase the use of NMPC for large-scale industrial applications for which classical NMPC is often limited due to its computational complexity.
更多查看译文
AI 理解论文
溯源树
样例
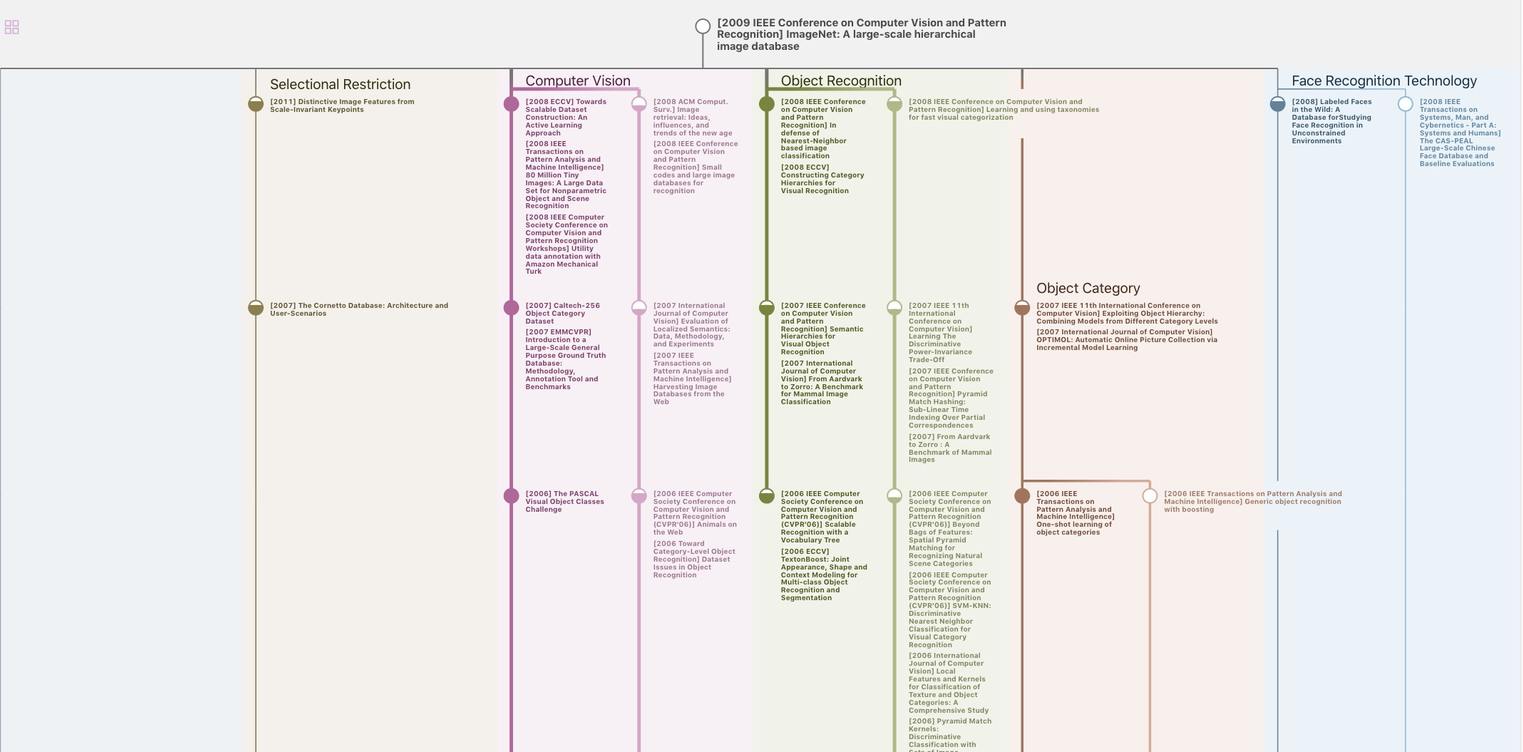
生成溯源树,研究论文发展脉络
Chat Paper
正在生成论文摘要