APE-V: Athlete Performance Evaluation using Video
2022 IEEE/CVF Winter Conference on Applications of Computer Vision Workshops (WACVW)(2022)
摘要
Athletes typically undergo regular evaluations by trainers and coaches to assess performance and injury risk. One of the most popular movements to examine in athletes needing lower extremity strength and power is the vertical jump. Specifically, maximal effort countermovement and drop jumps performed on bilateral force plates provide a wealth of metrics. However, the expense of the equipment and expertise needed to interpret the results limits their use. Computer vision techniques applied to videos of such movements are a less expensive alternative for extracting complementary metrics. Blanchard et al. [4] collected a dataset of 89 athletes performing these movements and showcased how OpenPose could be applied to the data. However, athlete error calls into question 46.2% of movements — in these cases, an expert assessor would have the athlete redo the movement to eliminate the error. Here, we augmented [4] with expert labels of error and established benchmark performance on automatic error identification. In total, 14 different types of errors were identified by trained annotators. Our benchmark models identified errors with an F1 score of 0.710 and a Kappa of 0.457 (Kappa measures accuracy over chance). All code and augmented labels can be found at https://blanchard-lab.github.io/apev.github.io/.
更多查看译文
AI 理解论文
溯源树
样例
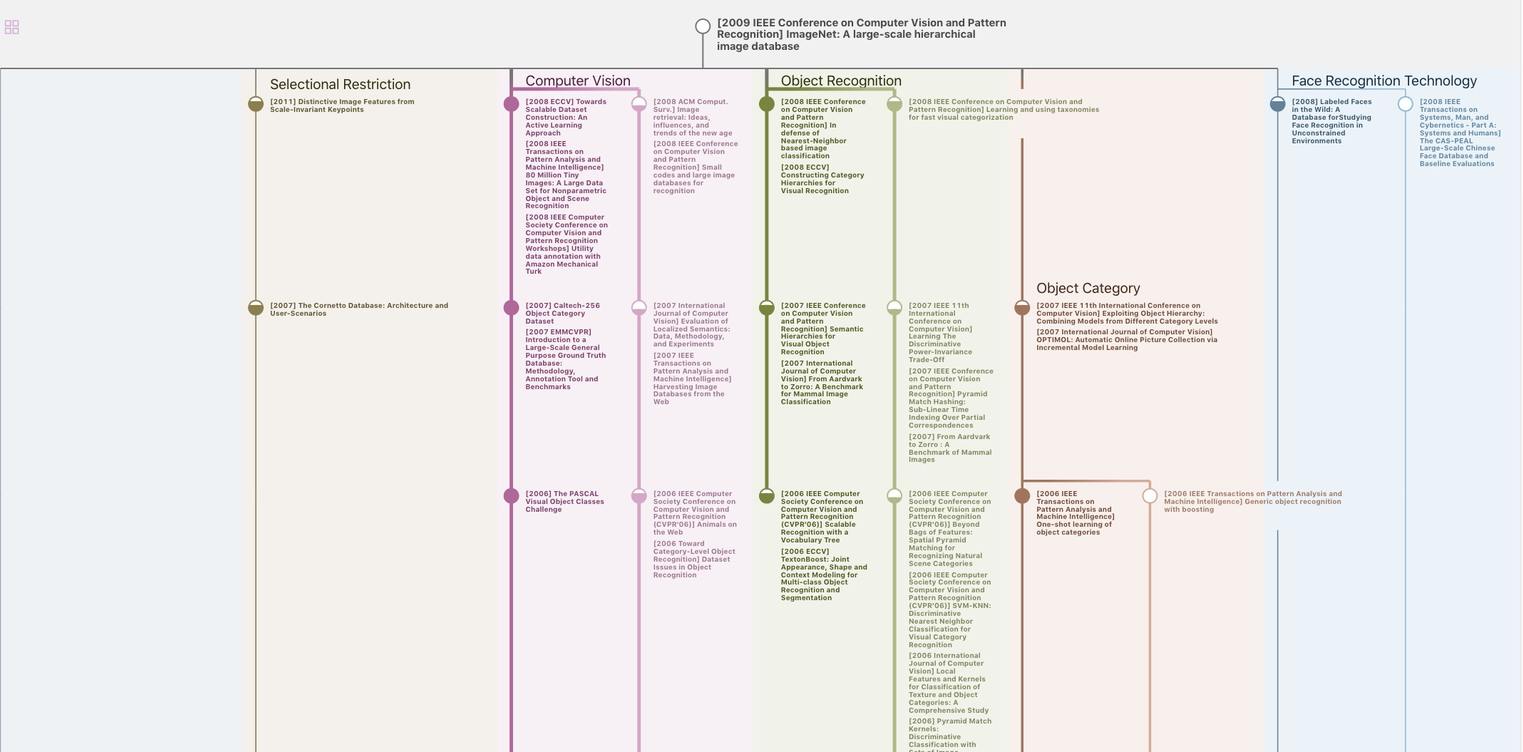
生成溯源树,研究论文发展脉络
Chat Paper
正在生成论文摘要