Complexity-based attentive interactive student performance prediction for personalized course study planning
Education and Information Technologies(2022)
摘要
Planning course study is critical to facilitate strategic intervention in education. As a significant basis of planning course study, student performance prediction aims to utilize students existing relevant information to predict their future learning performance including course grades, course failure, grade point average, etc. We target course grade prediction for course study planning, provide reminders for students’ learning and teachers’ teaching. A student’s performance is usually influenced by a variety of factors, such as the difficulty of the test, personal performance, and learning conditions. Recent studies focus on the various factors that influence student performance. However, most of them ignore the differences in the impact of these factors on different students. Moreover, existing prediction models pay less attention to the impact of test complexity on students’ performance. To tackle these problems, we propose a novel Complexity-based Attentive Interactive Student Performance prediction model (CAISP) for personalized course study planning. In CAISP, the individual differences among students can be considered by assigning dynamically different importance using attention network to facilitate personalized planning. Especially, to effectively alleviate the student performance bias caused by test complexity, we fuse test complexity features to enhance the ability of feature representation via deep neural networks. Meanwhile, we combine factorization machines to develop a deep joint representation based on interaction learning and further boost the correlation between features, leading to optimal prediction results. Extensive experiments on two real-world datasets show that CAISP outperforms the state-of-the-art solutions.
更多查看译文
关键词
Performance prediction,Factorization machines,Interaction learning,Attention network,Educational data mining
AI 理解论文
溯源树
样例
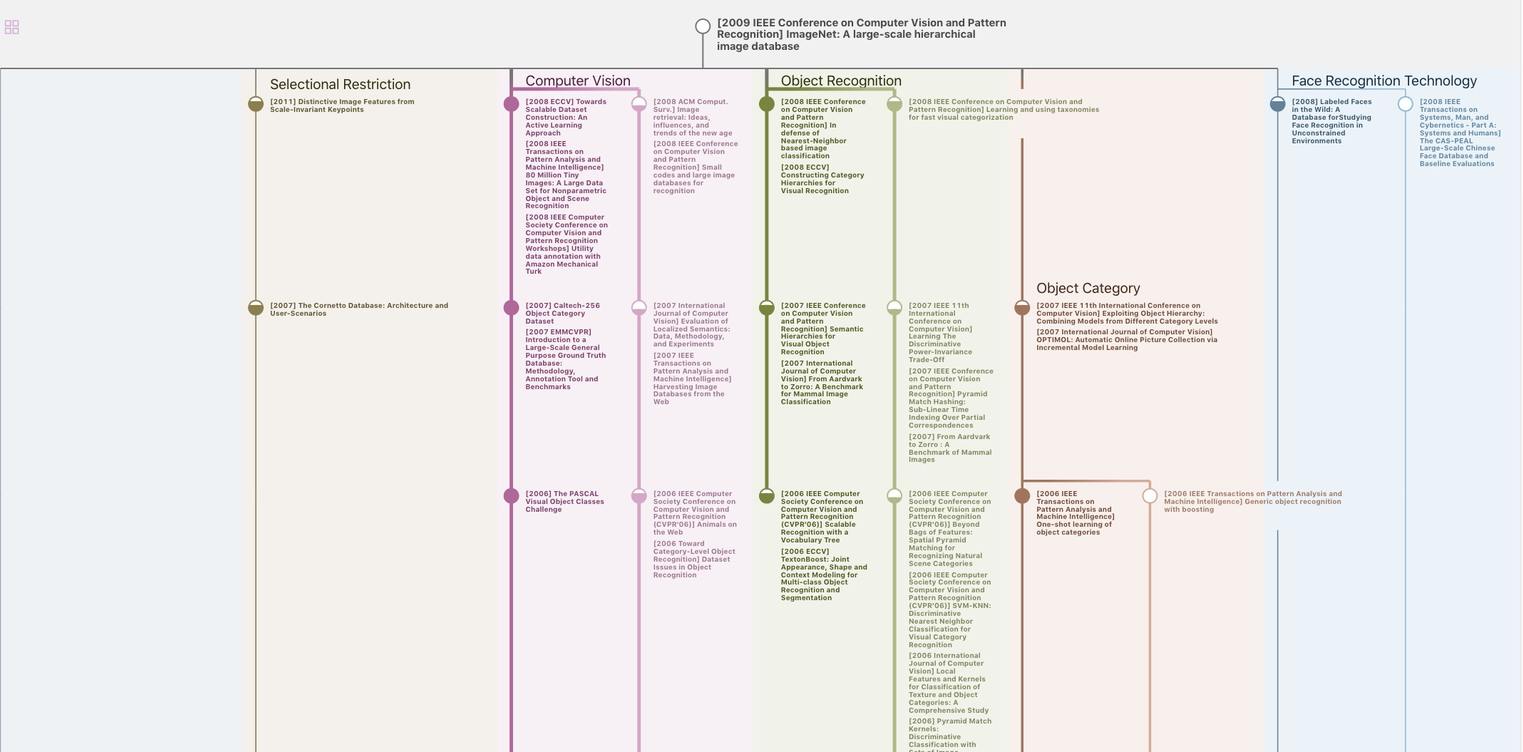
生成溯源树,研究论文发展脉络
Chat Paper
正在生成论文摘要