Domain Generalization with Small Data
International Journal of Computer Vision(2024)
摘要
In this work, we propose to tackle the problem of domain generalization in the context of insufficient samples . Instead of extracting latent feature embeddings based on deterministic models, we propose to learn a domain-invariant representation based on the probabilistic framework by mapping each data point into probabilistic embeddings. Specifically, we first extend empirical maximum mean discrepancy (MMD) to a novel probabilistic MMD that can measure the discrepancy between mixture distributions (i.e., source domains) consisting of a series of latent distributions rather than latent points. Moreover, instead of imposing the contrastive semantic alignment (CSA) loss based on pairs of latent points, a novel probabilistic CSA loss encourages positive probabilistic embedding pairs to be closer while pulling other negative ones apart. Benefiting from the learned representation captured by probabilistic models, our proposed method can marriage the measurement on the distribution over distributions (i.e., the global perspective alignment) and the distribution-based contrastive semantic alignment (i.e., the local perspective alignment). Extensive experimental results on three challenging medical datasets show the effectiveness of our proposed method in the context of insufficient data compared with state-of-the-art methods.
更多查看译文
关键词
Domain generalization,Healthcare,Small data,Medical imaging
AI 理解论文
溯源树
样例
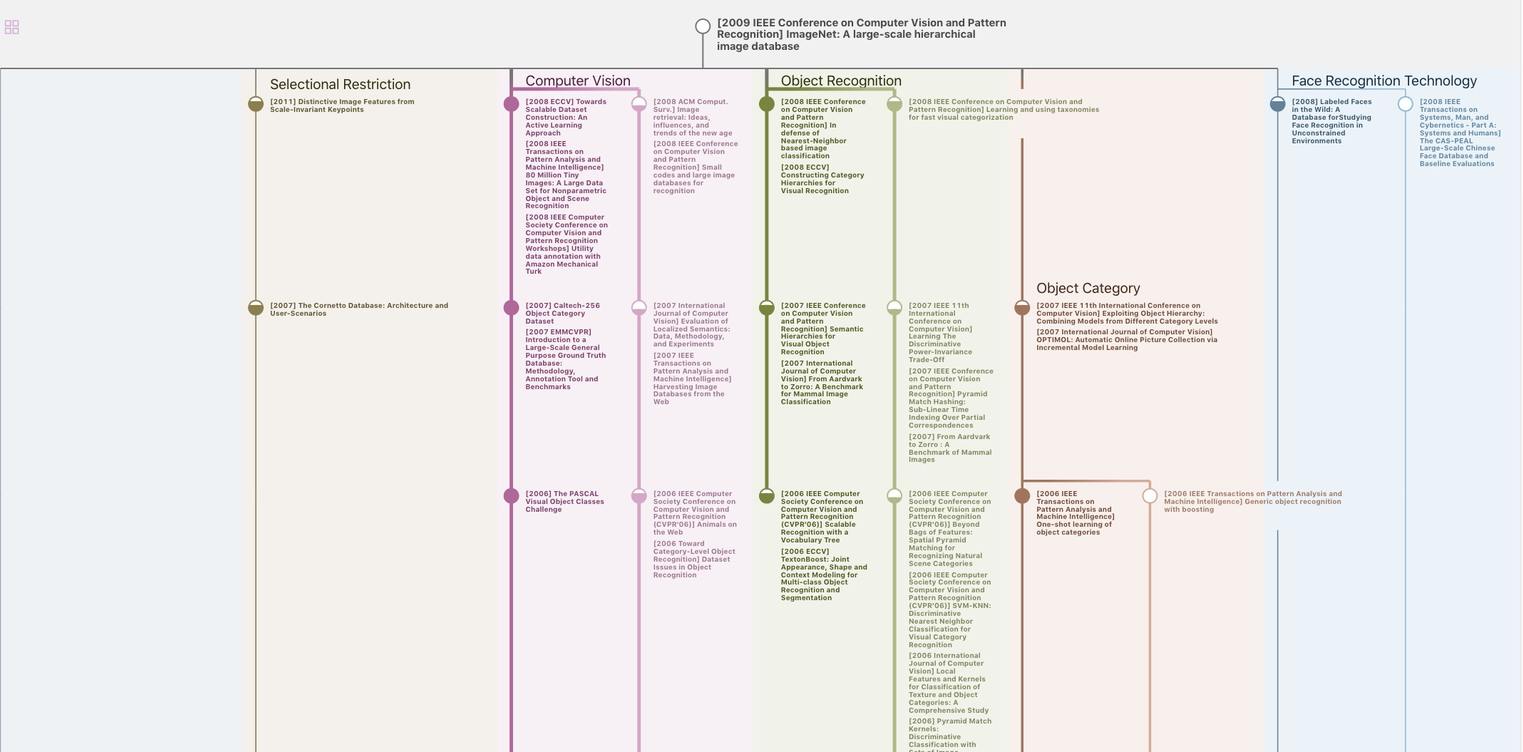
生成溯源树,研究论文发展脉络
Chat Paper
正在生成论文摘要