Nonlinear directed information flow estimation for fNIRS brain network analysis based on the modified multivariate transfer entropy
Biomedical Signal Processing and Control(2022)
摘要
Due to the specific advantages of functional near-infrared spectroscopy (fNIRS), fNIRS-based brain network research, especially directed causal coupling analysis, is emerging gradually. The transfer entropy (TE) method has proved its superiority and effectiveness in identifying directed information flow in the brain network, which helps us further understand the neurodynamic mechanisms. However, there remain three inherent defects in TE: (1) lack of multivariable effect, (2) poor robustness, and (3) dimensional curse in the high dimensional system. This study introduces a novel multivariate transfer entropy based on the nonuniform embedding, abbreviated “MTE NUE ,” to solve the above limits and construct the whole brain's directed coupling network. The MTE NUE thoroughly considers the potential influence of remaining confounding variables in conditional mutual information estimation, guaranteeing its applicability in a multivariable system. By combining low-dimensional approximation and search strategy of particle swarm optimization, a novel nonuniform embedding (NUE) algorithm was introduced to establish the MTE NUE methodology. Using a well-known Hénon map model, we carried out a series of numerical simulations to verify the performances of MTE NUE and compared them with the traditional TE methods. The numerical simulations prove that the proposed MTE NUE allows directional coupling estimation for a high dimensional, nonlinear, nonstationary system. The MTE NUE achieves the best robustness to data length and noise. Meanwhile, it presents the best linearity for coupling parameters “C,” with its higher fitting coefficients of determination (R-square) than other methods. Moreover, by avoiding the dimensional curse, the MTE NUE achieves the highest accuracy and specificity, which are higher than 98%; in contrast, the traditional methods are less than 80%. Finally, we applied the proposed method to the actual fNIRS research. The results demonstrate a typical information flow pattern changes during the working memory tasks, which is expected to provide new insights into the underlying neural dynamics in the cognitive process.
更多查看译文
关键词
fNIRS,Directed information flow,Brain network,Multivariate transfer entropy,Nonuniform embedding
AI 理解论文
溯源树
样例
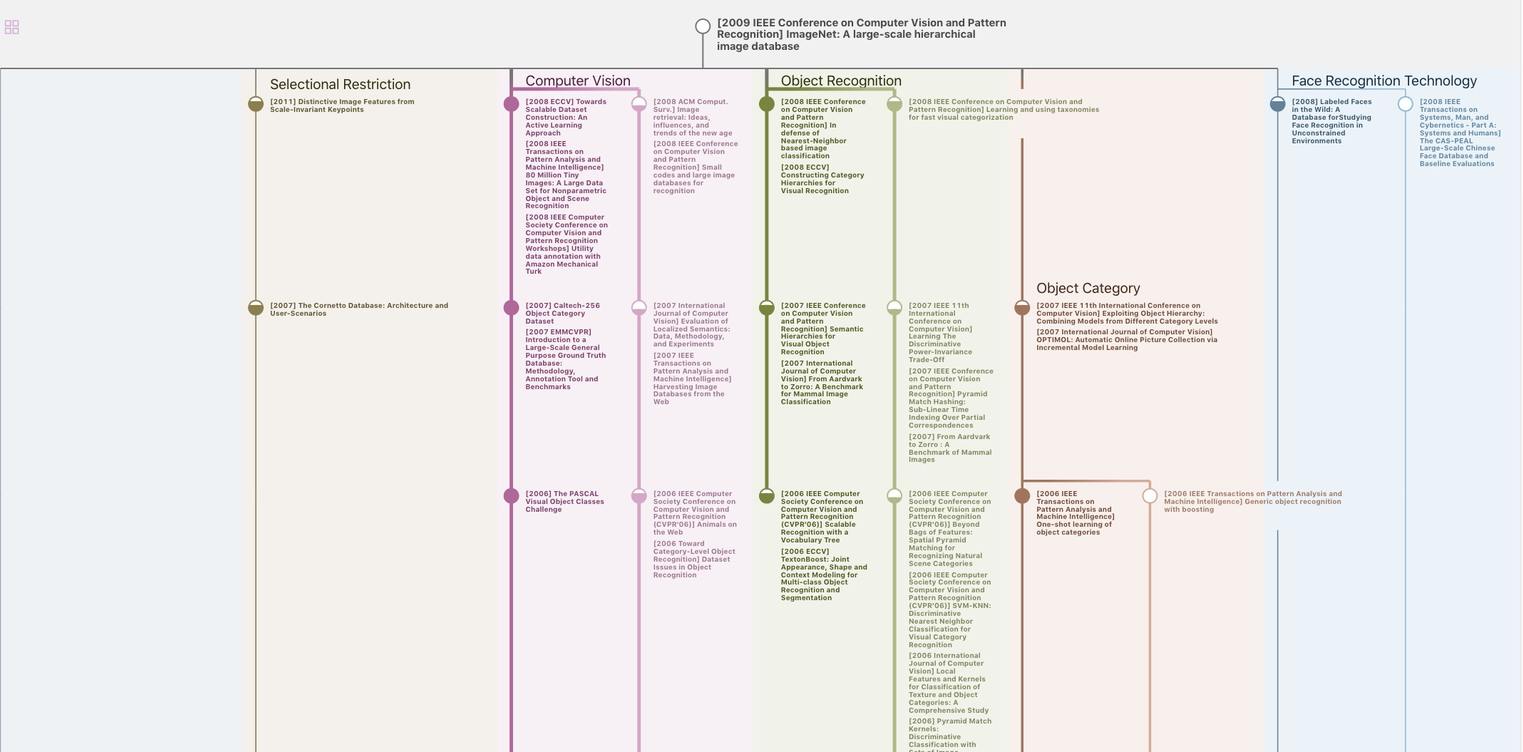
生成溯源树,研究论文发展脉络
Chat Paper
正在生成论文摘要