Joint Communication and Computation Resource Allocation in Fog-Based Vehicular Networks
IEEE Internet of Things Journal(2022)
摘要
To satisfy the low-latency requirements of emerging computation-intensive vehicular services, offloading these services to edge or cloud servers has been recognized as an effective solution. Due to the limited resources of edge servers and the faraway distance of cloud servers, it is challenging to provide an efficient resource allocation strategy to balance the latency, throughput and the resource utilization. In this paper, an end–edge–cloud collaboration paradigm is presented for computation offloading in fog-based vehicular networks (FVNETs) by incorporating vehicles with idle resources as fog user equipments (F-UEs). To adaptively orchestrate end–edge–cloud resources in different load cases, a two-timescale resource reservation and allocation framework is proposed. Wherein, a Stackelberg-game-based dynamic F-UE incentive problem is first formulated with the cloud server as the leader and multiple F-UEs as the followers, and then an iterative algorithm is proposed to achieve the Stackelberg equilibrium of the computation resource pricing and reservation. On a small timescale, the joint communication and computation resource allocation problem is transferred into a multiagent stochastic game and a lenient multiagent deep-reinforcement-learning-based distributed algorithm is developed to minimize the sum latency. When latency performance deteriorates, F-UE incentive optimization will be triggered to reserve more resources of F-UEs. Simulation results show that the proposed end–edge–cloud orchestrated computation offloading scheme in FVNETs outperforms baselines in terms of average latency.
更多查看译文
关键词
Computation offloading,fog-computing-based vehicular networks (VNs),multiagent deep reinforcement learning (DRL),resource allocation
AI 理解论文
溯源树
样例
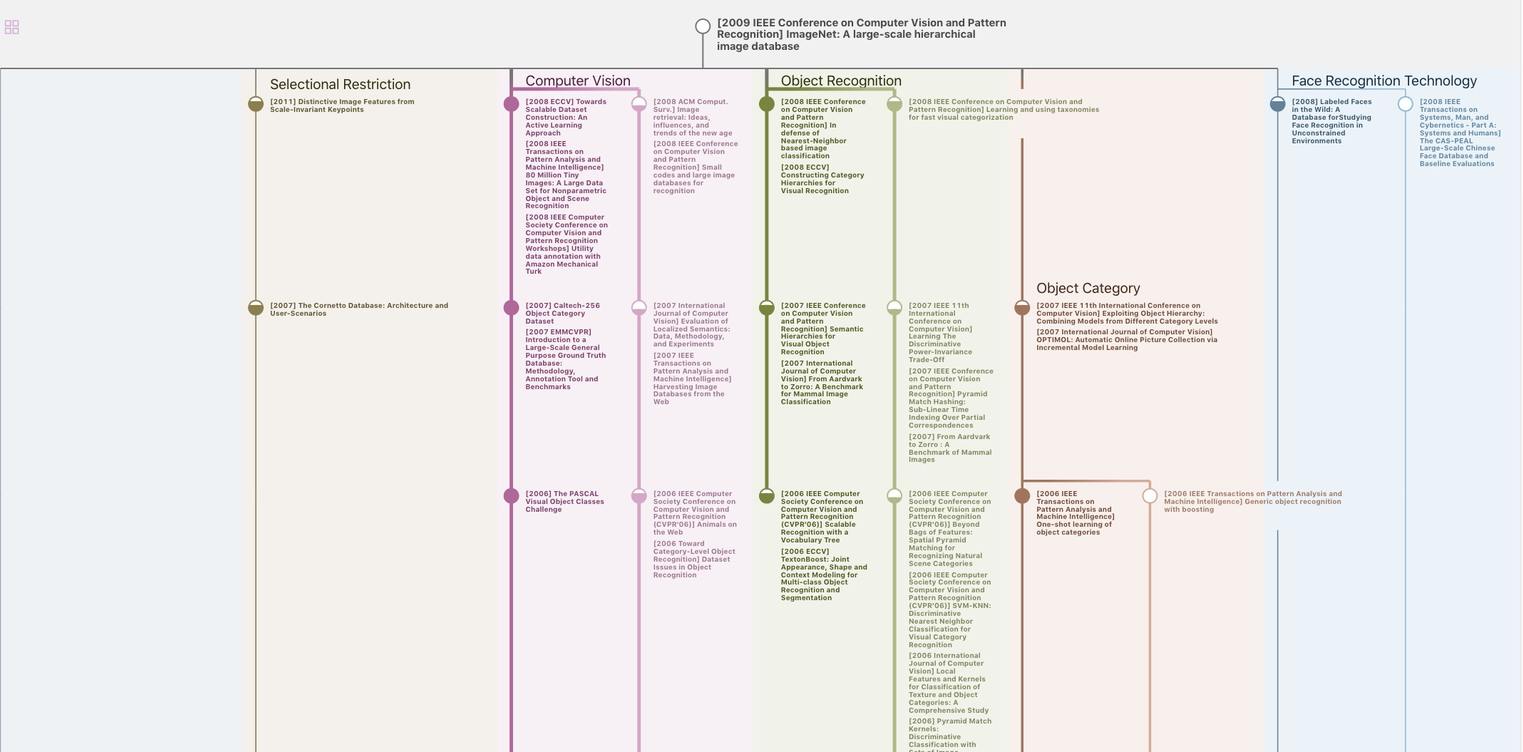
生成溯源树,研究论文发展脉络
Chat Paper
正在生成论文摘要