Thai Fingerspelling Recognition Using Hand Landmark Clustering
2021 25th International Computer Science and Engineering Conference (ICSEC)(2021)
摘要
As of June 2021, there are around 393,000 registered hearing-impaired persons in Thailand. Sign language is the only communication tool for them but, besides the deaf, only very small groups of people have learned how to use it. The deaf can learn things and the sign language interpreters may come to translate the voices for them, but it is tough for them to explain things to normal people that do not know anything about sign language. To overcome this obstacle, several groups of researchers are developing systems for sign language recognition. Although sign languages are different from region to region, their basis, fingerspelling, is somehow similar. Gestures of fingerspelling are simple, but they can be similar to others with different meanings. In this paper, we propose a two-level approach for Thai fingerspelling recognition. We focus on 25 basic gestures that can be combined to spell all Thai alphabets. The contribution of our paper is three folds. First, Hand Landmark, recently introduced in 2020, has been used as the data representation. Thus, instead of using a whole figure of size, says 640x480 pixels, our data representation is very compact as merely 21 points per hand. Second, two-level classification has been introduced for handling similar fingerspelling gestures by using key point clustering to grouping similar hand postures. Third, different from other studies, completely unseen data has been used in the experiments. Most studies just split the data to both training set and testing set. In our study, we firstly split people into two groups: one for training and one for testing. Only the gestures of the people in the former group are used during the training process. The latter group will be used during the testing process. Then, no leak of information from the latter group to the model. We evaluated our model by using precision, recall, accuracy, and F-measure. The results show that our method is performing well on the traditional train/test splitting scheme while it is still robust on the completely unseen data.
更多查看译文
关键词
Thai fingerspelling,Key Point,Hand Landmark,Data clustering,2-level classification
AI 理解论文
溯源树
样例
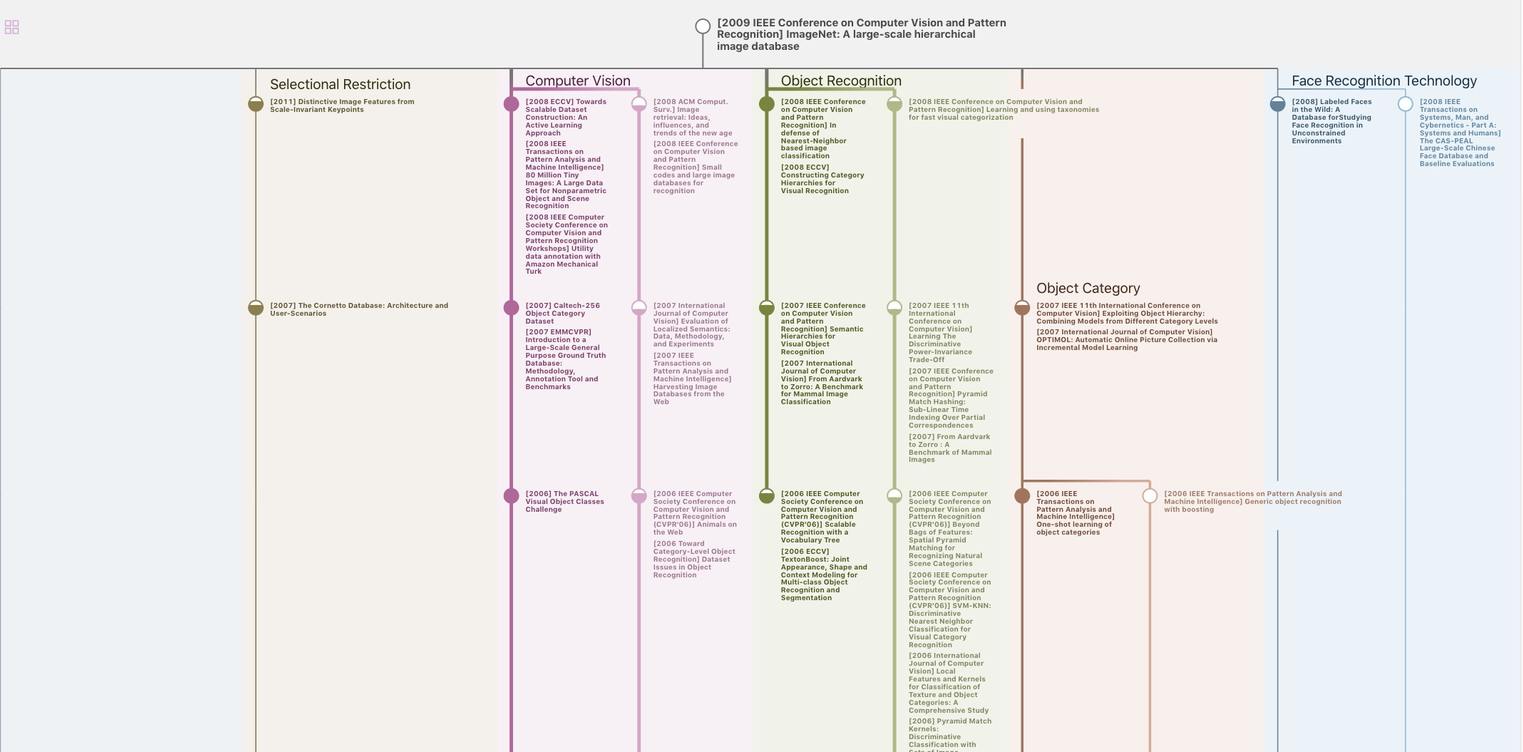
生成溯源树,研究论文发展脉络
Chat Paper
正在生成论文摘要