Autoregressive based Drift Detection Method
IEEE International Joint Conference on Neural Network (IJCNN)(2022)
摘要
In the classic machine learning framework, models are trained on historical data and used to predict future values. It is assumed that the data distribution does not change over time (stationarity). However, in real-world scenarios, the data generation process changes over time and the model has to adapt to the new incoming data. This phenomenon is known as concept drift and leads to a decrease in the predictive model's performance. In this study, we propose a new concept drift detection method based on autoregressive models called ADDM. This method can be integrated into any machine learning algorithm from deep neural networks to simple linear regression model. Our results show that this new concept drift detection method outperforms the state-of-the-art drift detection methods, both on synthetic data sets and real-world data sets. Our approach is theoretically guaranteed as well as empirical and effective for the detection of various concept drifts. In addition to the drift detector, we proposed a new method of concept drift adaptation based on the severity of the drift.
更多查看译文
关键词
Concept drift detection,Data streams,Autoregressive model,Machine learning,Deep neural networks
AI 理解论文
溯源树
样例
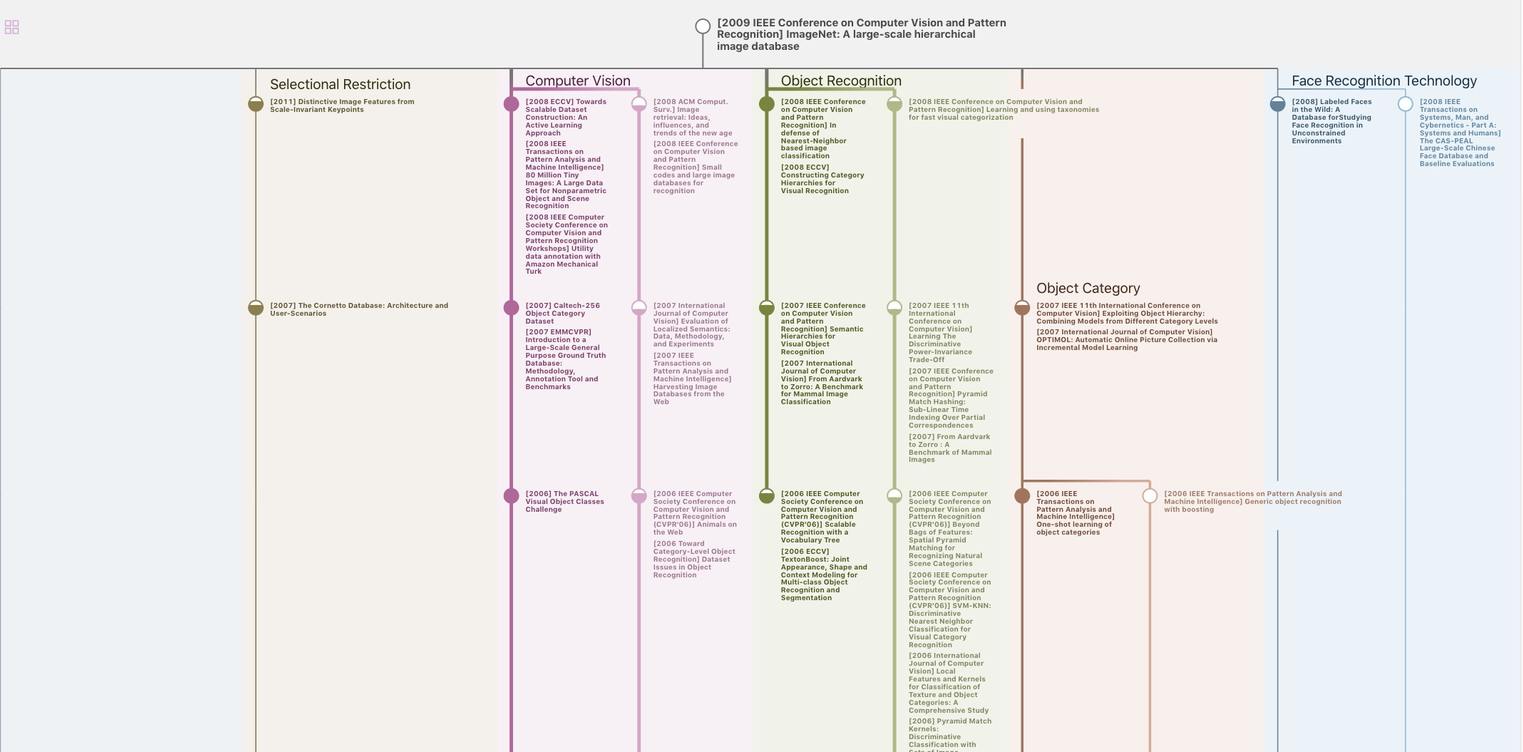
生成溯源树,研究论文发展脉络
Chat Paper
正在生成论文摘要