Learning Progressive Distributed Compression Strategies From Local Channel State Information
IEEE Journal of Selected Topics in Signal Processing(2022)
摘要
This paper proposes a deep learning framework to design distributed compression strategies in which distributed agents need to compress high-dimensional observations of a source, then send the compressed bits via bandwidth limited links to a fusion center for source reconstruction. Further, we require the compression strategy to be progressive so that it can adapt to the varying link bandwidths between the agents and the fusion center. Moreover, to ensure scalability, we investigate strategies that depend only on the local channel state information (CSI) at each agent. Toward this end, we use a data-driven approach in which the progressive linear combination and uniform quantization strategy at each agent are trained as a function of its local CSI. To deal with the challenges of modeling the quantization operations (which always produce zero gradients in the training of neural networks), we propose a novel approach of exploiting the statistics of the batch training data to set the dynamic ranges of the uniform quantizers. Numerically, we show that the proposed distributed estimation strategy designed with only local CSI can significantly reduce the signaling overhead and can achieve a lower mean-squared error distortion for source reconstruction than state-of-the-art designs that require global CSI at comparable overall communication cost.
更多查看译文
关键词
Deep learning,distributed compression,distributed estimation,progressive transmission,quantization
AI 理解论文
溯源树
样例
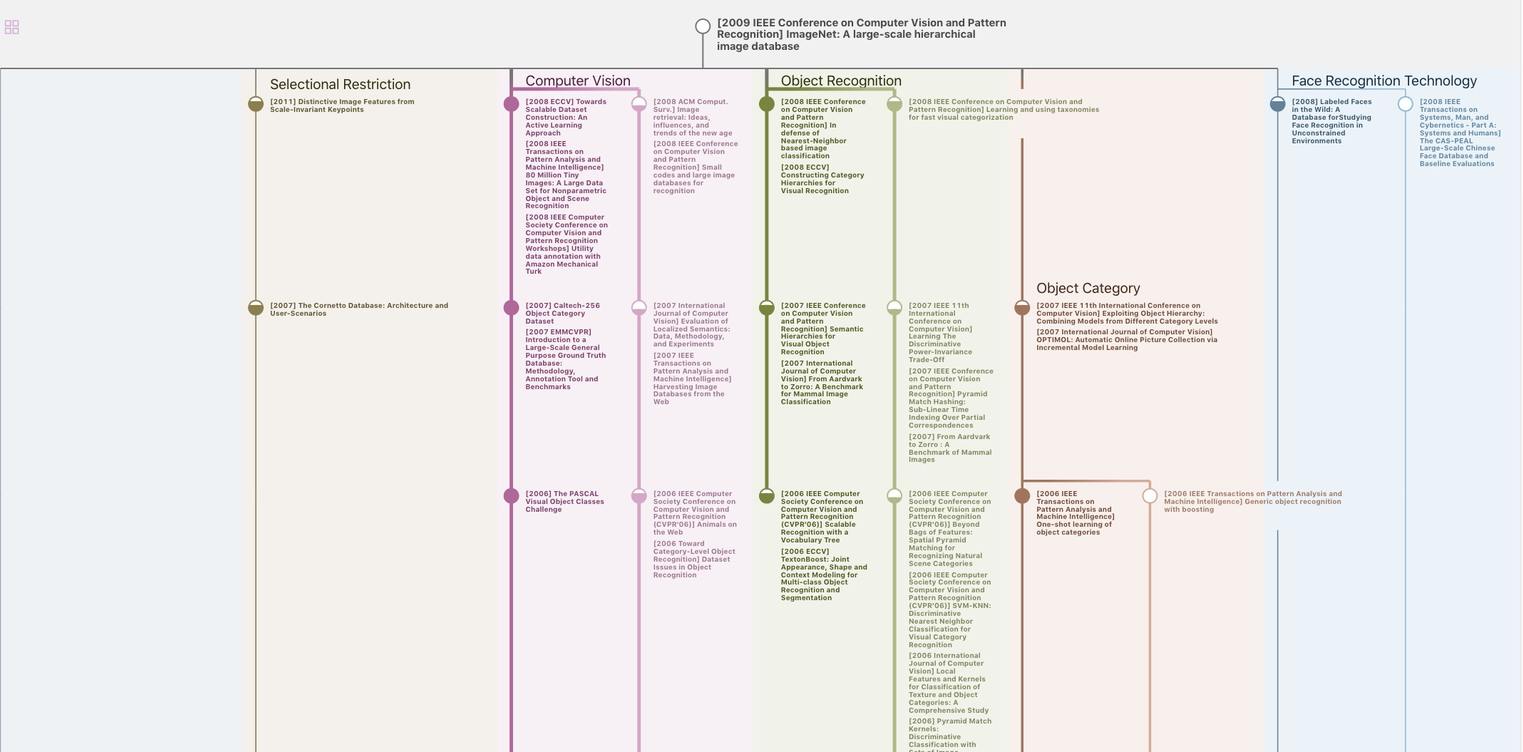
生成溯源树,研究论文发展脉络
Chat Paper
正在生成论文摘要