Bilinear Scoring Function Search for Knowledge Graph Learning
IEEE Transactions on Pattern Analysis and Machine Intelligence(2023)
摘要
Learning embeddings for entities and relations in knowledge graph (KG) have benefited many downstream tasks. In recent years, scoring functions, the crux of KG learning, have been human designed to measure the plausibility of triples and capture different kinds of relations in KGs. However, as relations exhibit intricate patterns that are hard to infer before training, none of them consistently perform the best on benchmark tasks. In this paper, inspired by the recent success of automated machine learning (AutoML), we search bilinear scoring functions for different KG tasks through the AutoML techniques. However, it is non-trivial to explore domain-specific information here. We first set up a search space for AutoBLM by analyzing existing scoring functions. Then, we propose a progressive algorithm (AutoBLM) and an evolutionary algorithm (AutoBLM+), which are further accelerated by filter and predictor to deal with the domain-specific properties for KG learning. Finally, we perform extensive experiments on benchmarks in KG completion, multi-hop query, and entity classification tasks. Empirical results show that the searched scoring functions are KG dependent, new to the literature, and outperform the existing scoring functions. AutoBLM+ is better than AutoBLM as the evolutionary algorithm can flexibly explore better structures in the same budget.
更多查看译文
关键词
Automated machine learning,graph embedding,knowledge graph,neural architecture search
AI 理解论文
溯源树
样例
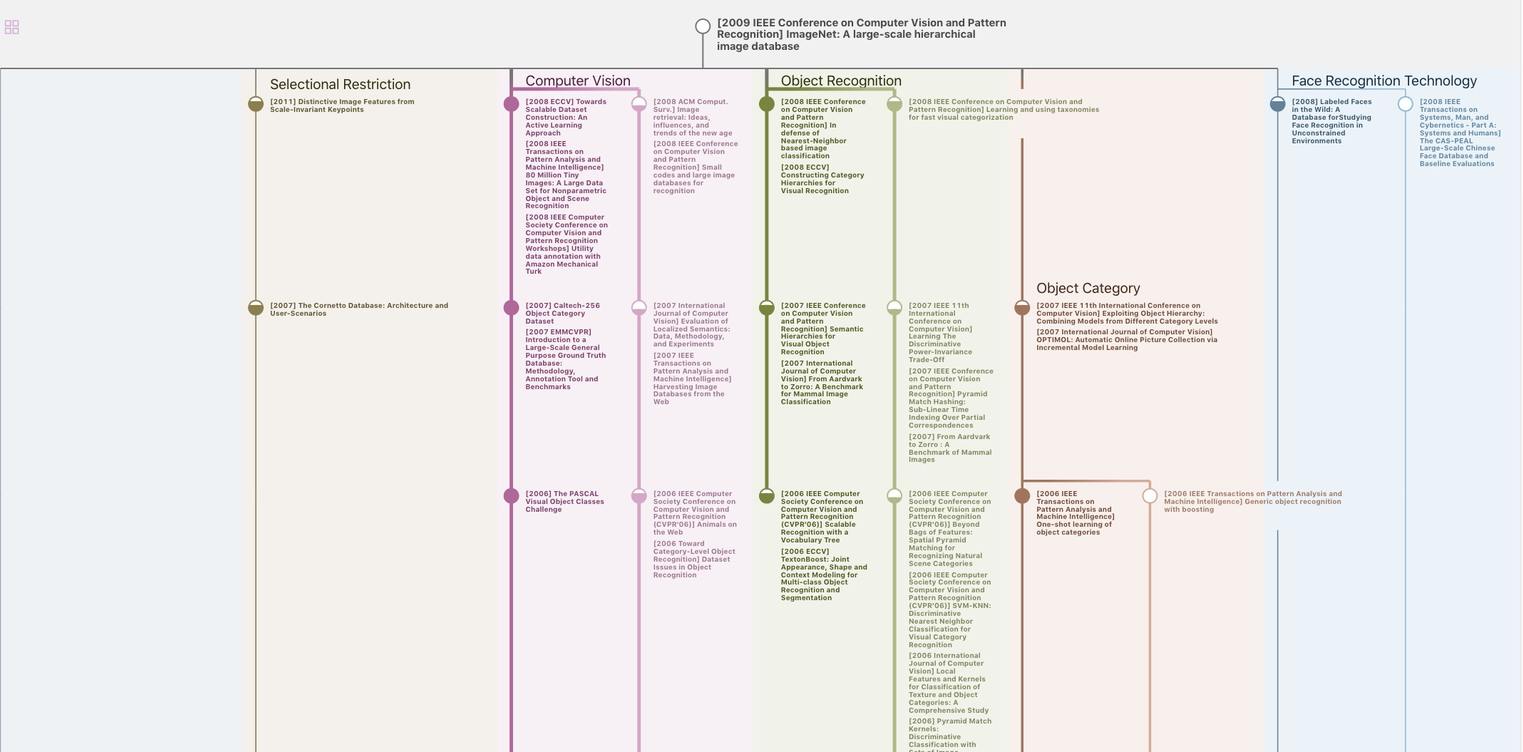
生成溯源树,研究论文发展脉络
Chat Paper
正在生成论文摘要