Classification of EEG Signals for Prediction of Seizure using Multi-Feature Extraction
2017 1st International Conference on Electronics, Materials Engineering and Nano-Technology (IEMENTech)(2017)
摘要
Pre-diagnosis of epileptic seizure using electroencephalogram (EEG) signals detecting seizure activity automatically using electroencephalogram (EEG) signals is of great importance and significance. Recording and reviewing of entire length of EEG is used to analyse epileptic activity by an expert. The classical method thus being a tedious task many intelligent automatic seizure detection schemes have been realised. Non linear approaches depict greater accuracy and have much higher significance. Our work focusses on obtaining a matrix of multi feature dimension including Brownian motion, Mean Absolute Value and Root Mean Square as key features. Features obtained from artefact free EEG signal are fed to intelligent classifiers in order to obtain the accuracy specificity and sensitivity values. Our proposed approach yields an overall accuracy of 74.78% for SVM, 89.13% for Weighted KNN and 91.09% for Ensemble Bagged Tree classifier. The ROC curve obtained by plotting the true positive rate against the false positive rate also shows significant region of convergence for the classifiers. This method can be utilised in designing intelligent seizure prediction systems.
更多查看译文
关键词
Seizure detection,Electroencephalogram(EEG),Mean Absolute Value(MAV),Root Mean Square(RMS),Brownian motion exponent,Multiple Classification Algorithms
AI 理解论文
溯源树
样例
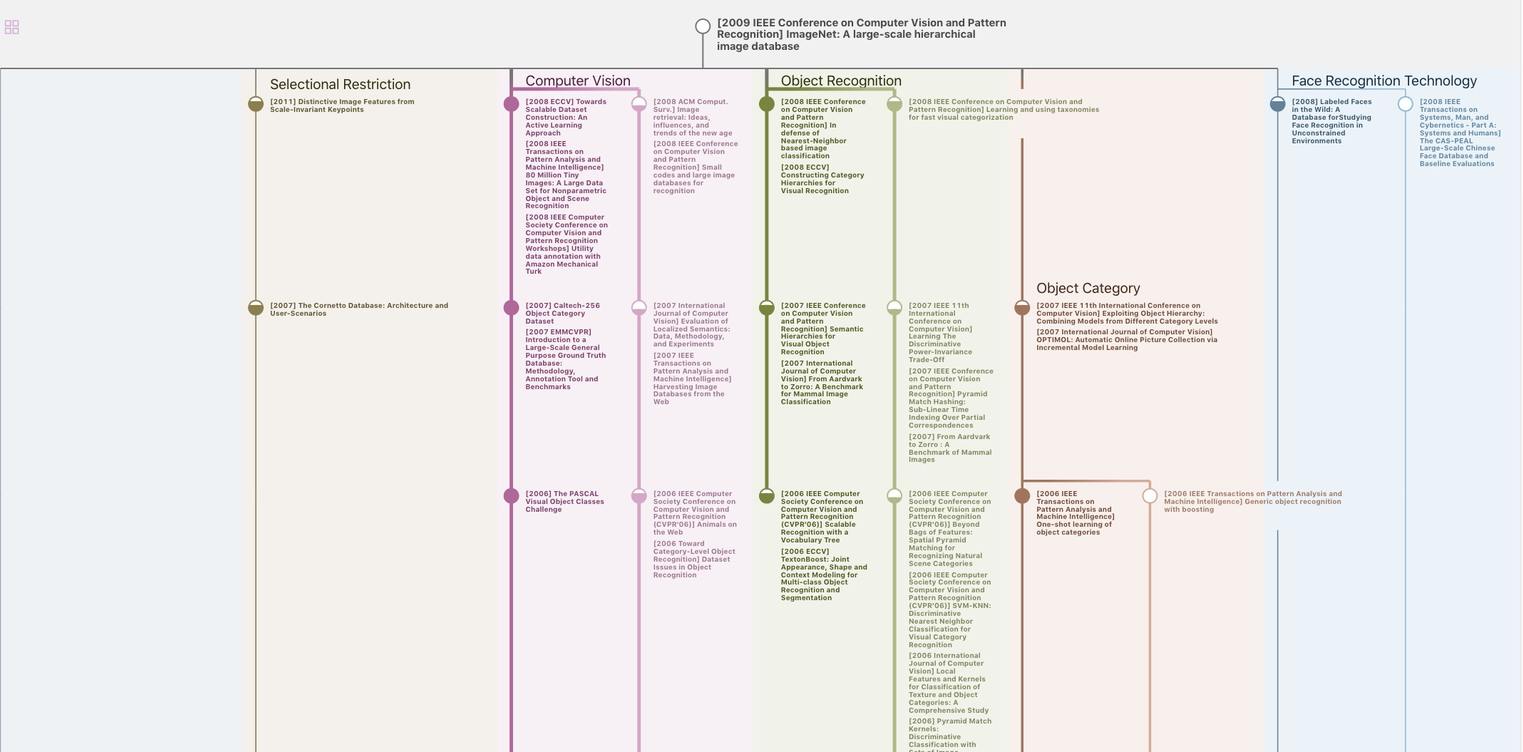
生成溯源树,研究论文发展脉络
Chat Paper
正在生成论文摘要