Cycle-Free Weakly Referring Expression Grounding With Self-Paced Learning
IEEE Transactions on Multimedia(2023)
摘要
In this paper, we are tackling the weakly referring expression grounding task to localize the target object in an image according to a given query sentence, where the mapping between the query sentence and image regions is blind during the training period. Previous methods all follow a cyclic forward-backward pipeline to handle this task, where the query sentence is firstly converted to the result region through the forward module, and then the result region is converted back to a sentence through the backward module, with the difference between the reconstructed sentence and original query used as the loss to optimize the entire network. These existing methods, however, suffer from the deviation issue when the result region, generated through the forward module, totally deviates from the target area, but the backward module still reconstructs a similar sentence. The aforementioned loss function cannot penalize this kind of deviation because of the consistent prediction of the sentence. To overcome this limitation, we propose a cycle-free pipeline, where a region describer network is designed to predict the textual description for each candidate region, and a result region is selected according to the similarity between the predicted description and the query sentence. Furthermore, a self-paced learning mechanism is designed to avoid the drift issue during the warm-up period of the optimization process. The proposed method achieves a higher average accuracy on RefCOCO and RefCOCO+ datasets, compared with all previous state-of-the-art methods.
更多查看译文
关键词
Referring expression grounding,weakly supervised learning,self-paced learning
AI 理解论文
溯源树
样例
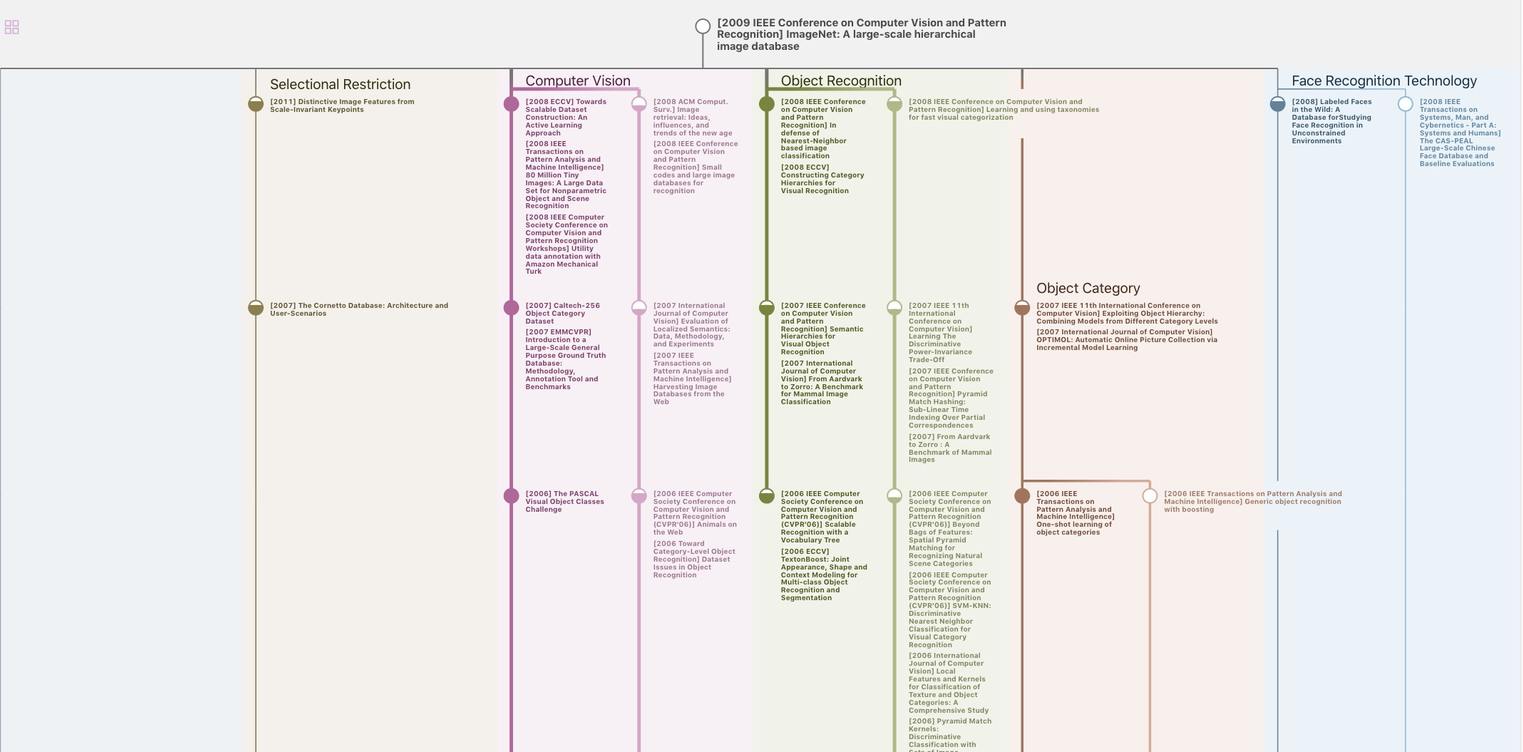
生成溯源树,研究论文发展脉络
Chat Paper
正在生成论文摘要