Price forecasting for real estate using machine learning: A case study on Riyadh city
CONCURRENCY AND COMPUTATION-PRACTICE & EXPERIENCE(2022)
摘要
Real estate is potentially contributing to the economic growth. It has a strong correlation between property owners and beneficiaries. The accurate forecast of future property prices is particularly important. Therefore, real estate prices are reflecting the economic level of counties, and their price ranges are of great interest to both buyers and sellers. Developing a land-price forecast model could significantly assist in predicting future land prices and setting real estate regulations. In contrast, machine learning (ML) algorithms have demonstrated a great potential to perform predictions. Motivated by these assumptions, we develop in this article a set of ML algorithms to build models capable to increase the effectiveness of land price estimation. The ML algorithms adopted in this work include the decision tree, random forest (RF), and linear regression. We collected data from 5946 lands localized in the northern area of Riyadh, KSA. This data has been collected using GeoTech's DAAL website. The performance of the developed models has been assessed based on state-of-art performance metrics including mean absolute error, mean squared error, and median squared error. The experiments show that the RF based model outperforms the remaining models.
更多查看译文
关键词
decision tree,linear regression,machine learning,price prediction,random forest
AI 理解论文
溯源树
样例
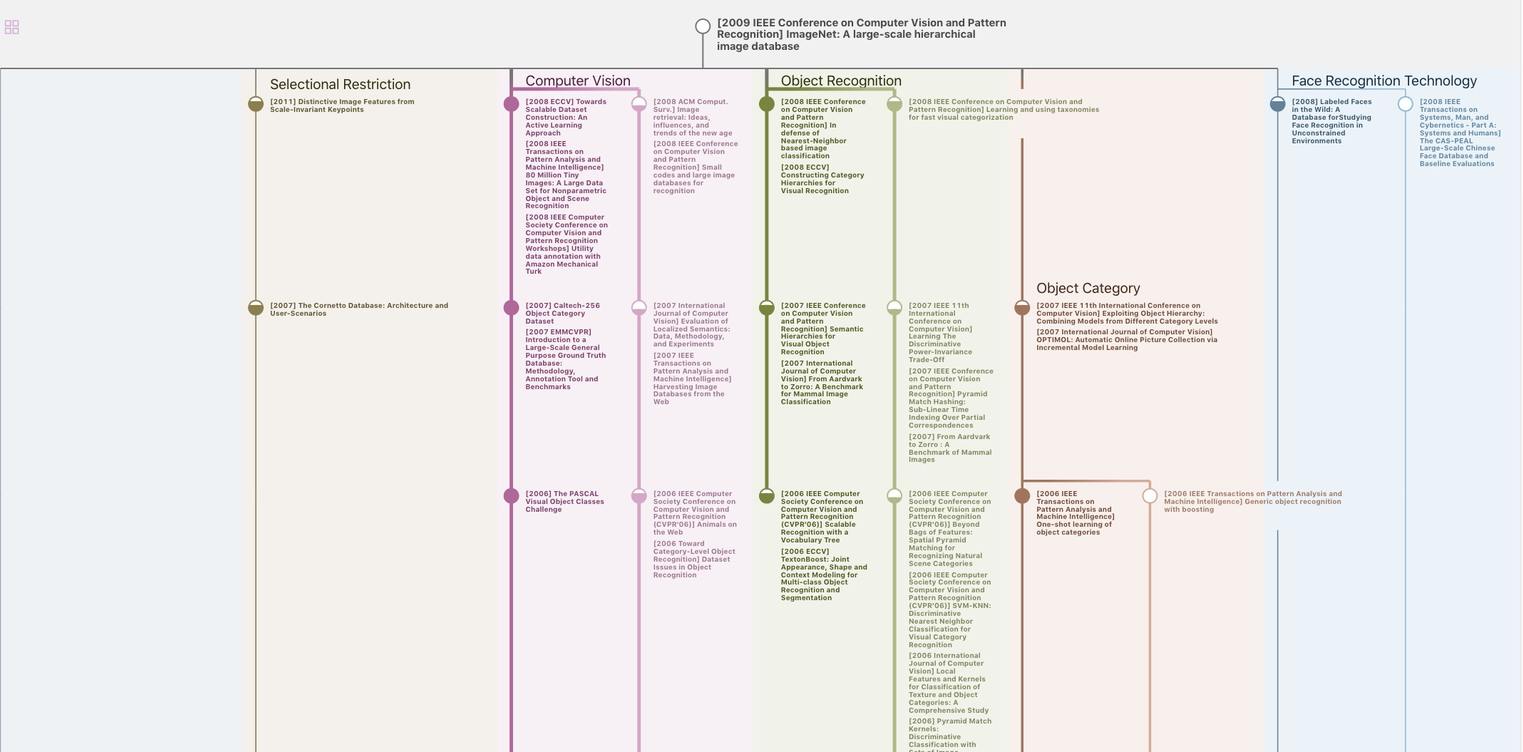
生成溯源树,研究论文发展脉络
Chat Paper
正在生成论文摘要