Class-Incremental Learning for Semantic Segmentation in Aerial Imagery via Distillation in All Aspects
IEEE TRANSACTIONS ON GEOSCIENCE AND REMOTE SENSING(2022)
摘要
Incremental learning using neural networks achieves great success in semantic segmentation but still suffers from catastrophic forgetting. In this article, we propose an effective class-incremental segmentation method without storing old data. To alleviate the issue of forgetting, we present two important modules, i.e., the deep feature distillation (DFD) module and the label mixed (LM) module. The DFD module is established to learn a good feature representation of old classes by distilling a new compact feature representation from different layers of networks. The proposed LM module first identifies the examples (pixels) of old classes with high confidences utilizing the output of old models, and then, they are combined with examples of new classes to supervise the training of new models, which can achieve a good balance between learning new classing and avoiding forgetting old ones. Our ablation studies show that the DFD module and the LM module can make the learning network obtain 6.2 & x0025; and 15 & x0025; performance gains [mean Intersection over Union (mIOU)], respectively. Furthermore, by introducing the supervision of output distillation loss, we compare our method with several state-of-the-art methods in the extensive experiments, and the experimental results all show that our method is significantly superior to them on the dataset of aerial images.
更多查看译文
关键词
Image segmentation,Task analysis,Semantics,Training,Computational modeling,Data models,Knowledge engineering,Class-incremental segmentation,deep feature distillation (DFD) module,incremental learning,label mixed (LM) module,semantic segmentation
AI 理解论文
溯源树
样例
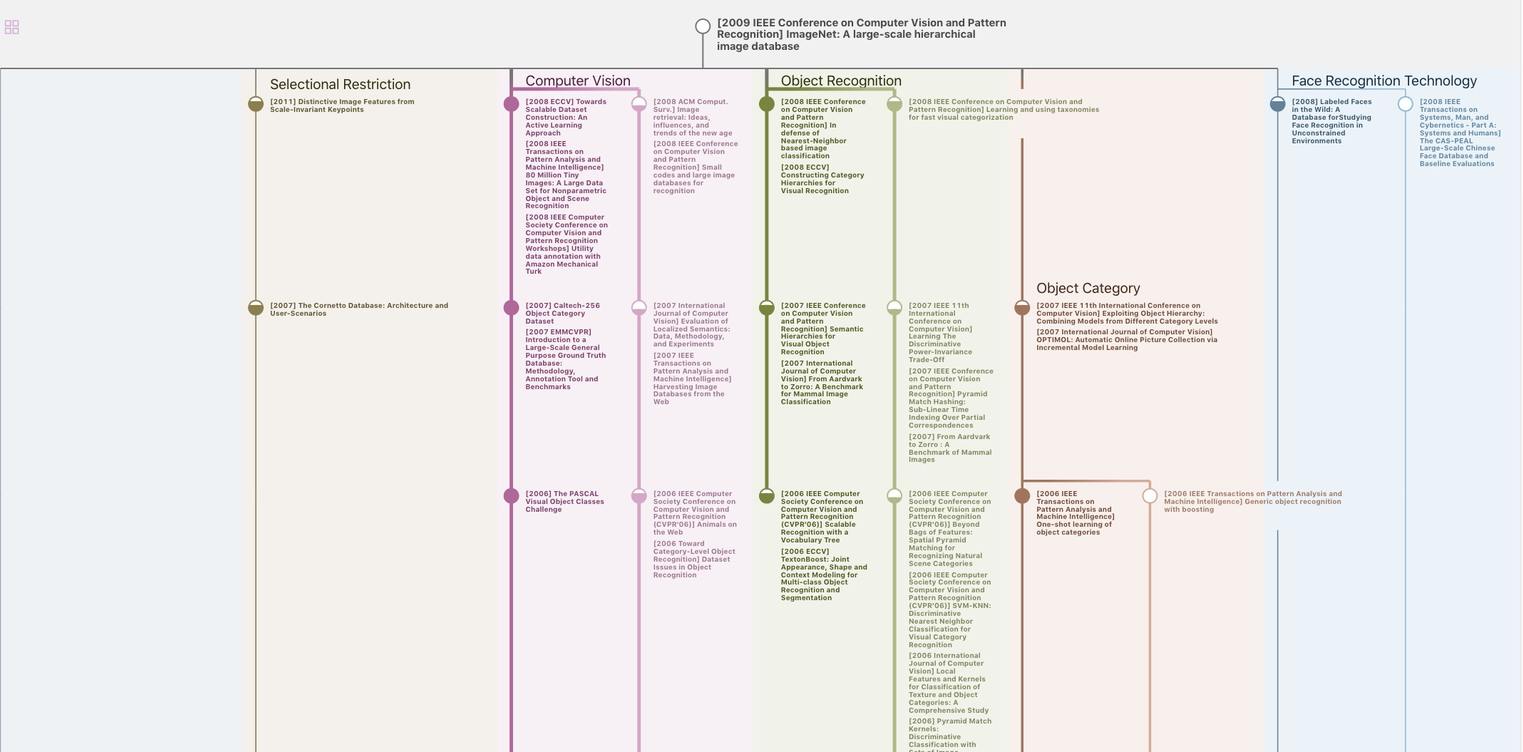
生成溯源树,研究论文发展脉络
Chat Paper
正在生成论文摘要