Interpretable machine learning-derived nomogram model for early detection of diabetic retinopathy in type 2 diabetes mellitus: a widely targeted metabolomics study
Social Science Research Network(2022)
摘要
Objective Early identification of diabetic retinopathy (DR) is key to prioritizing therapy and preventing permanent blindness. This study aims to propose a machine learning model for DR early diagnosis using metabolomics and clinical indicators. Methods From 2017 to 2018, 950 participants were enrolled from two affiliated hospitals of Wenzhou Medical University and Anhui Medical University. A total of 69 matched blocks including healthy volunteers, type 2 diabetes, and DR patients were obtained from a propensity score matching-based metabolomics study. UPLC-ESI-MS/MS system was utilized for serum metabolic fingerprint data. CART decision trees (DT) were used to identify the potential biomarkers. Finally, the nomogram model was developed using the multivariable conditional logistic regression models. The calibration curve, Hosmer–Lemeshow test, receiver operating characteristic curve, and decision curve analysis were applied to evaluate the performance of this predictive model. Results The mean age of enrolled subjects was 56.7 years with a standard deviation of 9.2, and 61.4% were males. Based on the DT model, 2-pyrrolidone completely separated healthy controls from diabetic patients, and thiamine triphosphate (ThTP) might be a principal metabolite for DR detection. The developed nomogram model (including diabetes duration, systolic blood pressure and ThTP) shows an excellent quality of classification, with AUCs (95% CI) of 0.99 (0.97–1.00) and 0.99 (0.95–1.00) in training and testing sets, respectively. Furthermore, the predictive model also has a reasonable degree of calibration. Conclusions The nomogram presents an accurate and favorable prediction for DR detection. Further research with larger study populations is needed to confirm our findings.
更多查看译文
关键词
Diabetes complications,Risk factors,Medicine/Public Health,general,Diabetes,Clinical Nutrition,Epidemiology,Metabolic Diseases,Internal Medicine
AI 理解论文
溯源树
样例
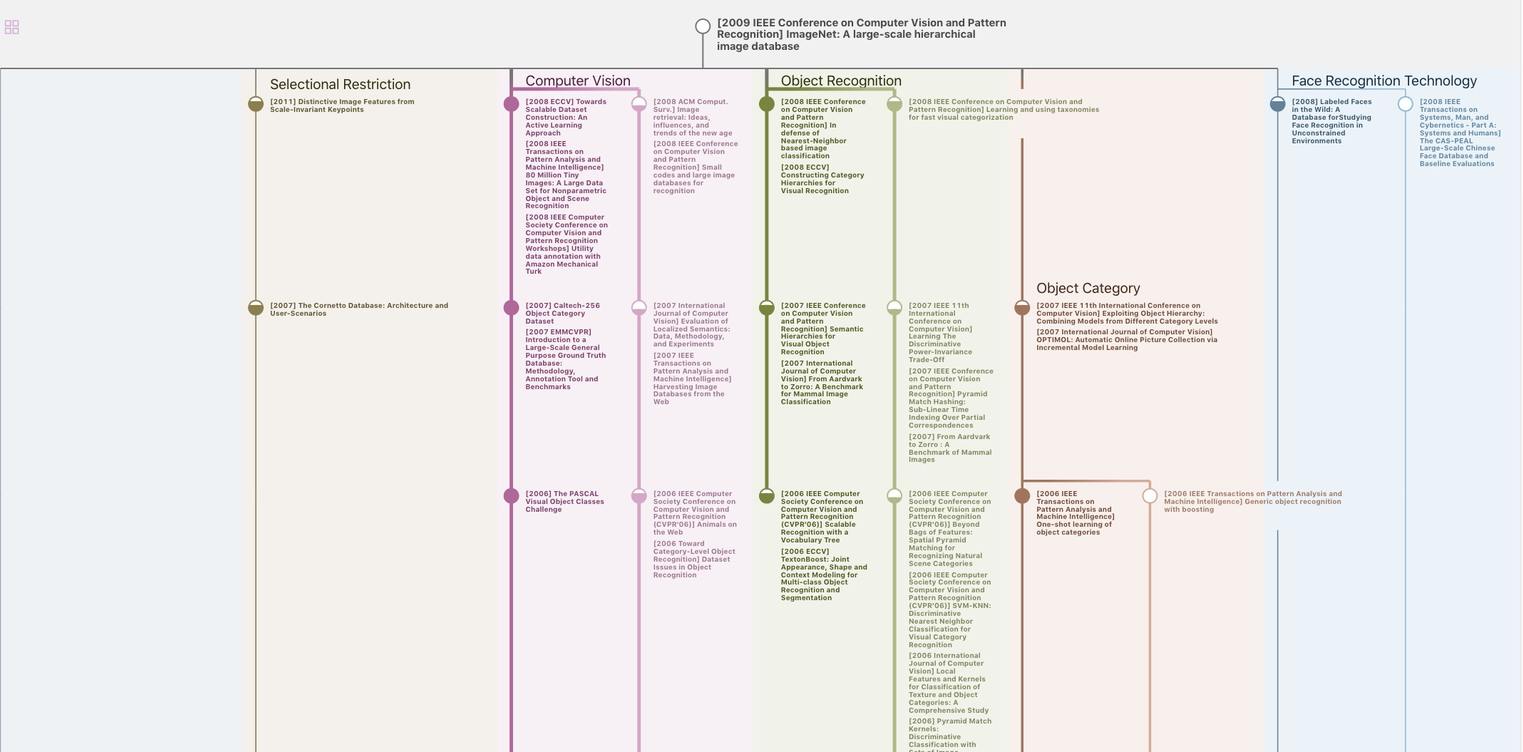
生成溯源树,研究论文发展脉络
Chat Paper
正在生成论文摘要