Early Warning of Incipient Faults for Power Transformer Based on DGA Using a Two-Stage Feature Extraction Technique
IEEE Transactions on Power Delivery(2022)
摘要
Early warning for transformer faults is valuable for maintenance decision-making. However, limited work has been done in this area due to the difficulty of the model establishment. This paper proposes a two-stage feature extraction method for early warning of power transformer faults, where a novel feature extraction process is applied by combining feature ranking and genetic programming (GP). In the first stage, the data is labeled as normal and fault states and the feature extraction is evaluated on the data. Then, extracted key features and their growth rates are relabeled as normal and warning states, after which the feature extraction process is re-evaluated on the relabeled data. Obtained features and logic expression can finally be used for early warning. The proposed framework can implement an early warning with about 100 days in advance for transformer faults and is verified through 8 sequences of data. The comparisons with two recently reported methods show the superiority of the proposed method.
更多查看译文
关键词
Dissolved gas analysis,transformer diagnosis,feature extraction,early warning,genetic programming
AI 理解论文
溯源树
样例
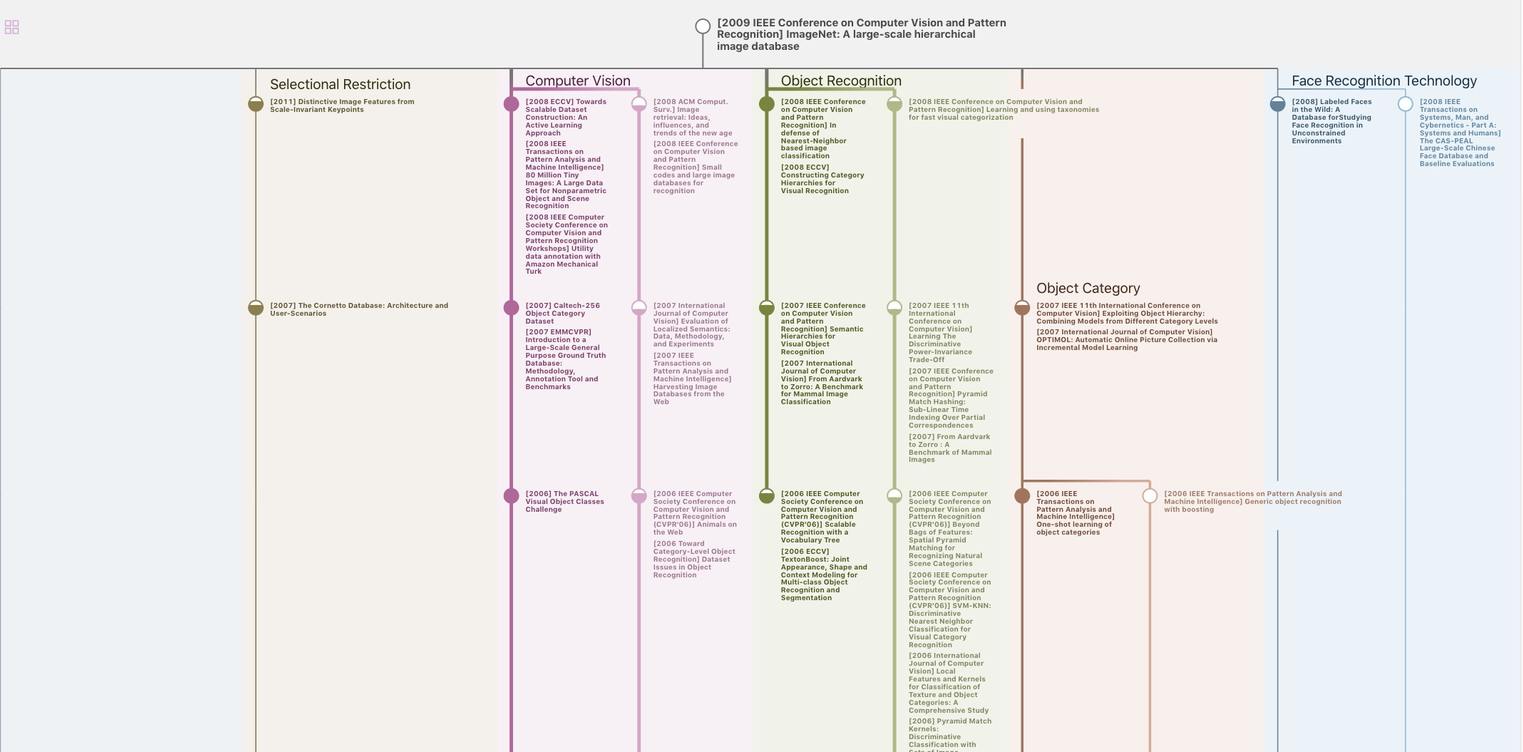
生成溯源树,研究论文发展脉络
Chat Paper
正在生成论文摘要