Challenge Accepted – Paratope States in Solution Improve Structure Prediction and Docking
Social Science Research Network(2021)
摘要
The rise of antibodies as biotherapeutic proteins has attracted the interest in characterizing and understanding the antibody binding interface. Structure-based antibody design and accurate predictions of antibody-antigen interactions remain major challenges in computational biology. By using molecular dynamics simulations in combination with enhanced sampling techniques, we show that a single static X-ray structure is not sufficient to identify determinants of antibody recognition and to characterize functionally relevant conformational rearrangements. Thus, we suggest that antibodies should be described as ensembles of paratope states in solution, which are defined by a combination of correlated loop movements, which favor distinct interface orientations and elbow angles. In this study, we focus our attention on characterizing paratope states in solution of antibodies which undergo substantial conformational changes upon antigen binding. Because of their conformational changes, they have been classified as “difficult” cases for antibody-antigen docking in the Critical Assessment of PRediction of Interactions (CAPRI) blind docking challenge. Here, we present transition kinetics and thermodynamics of these conformational rearrangements of the paratope and show that kinetically relevant states can be used to improve antibody-antigen docking. We use the Rosetta SnugDock docking algorithm to show that by even using the unbound antibody X-ray structure as starting structures for molecular dynamics simulations, a binding competent conformation substantially different to the unbound antibody X-ray structure, can be retained. We also observe that the kinetically dominant antibody conformations are chosen by the bound antigen conformation with the highest probability. Thus, we show that kinetically relevant antibody conformations obtained by a combination of molecular dynamics simulations and Markov-state models can improve antibody-antigen docking and structure prediction.
更多查看译文
AI 理解论文
溯源树
样例
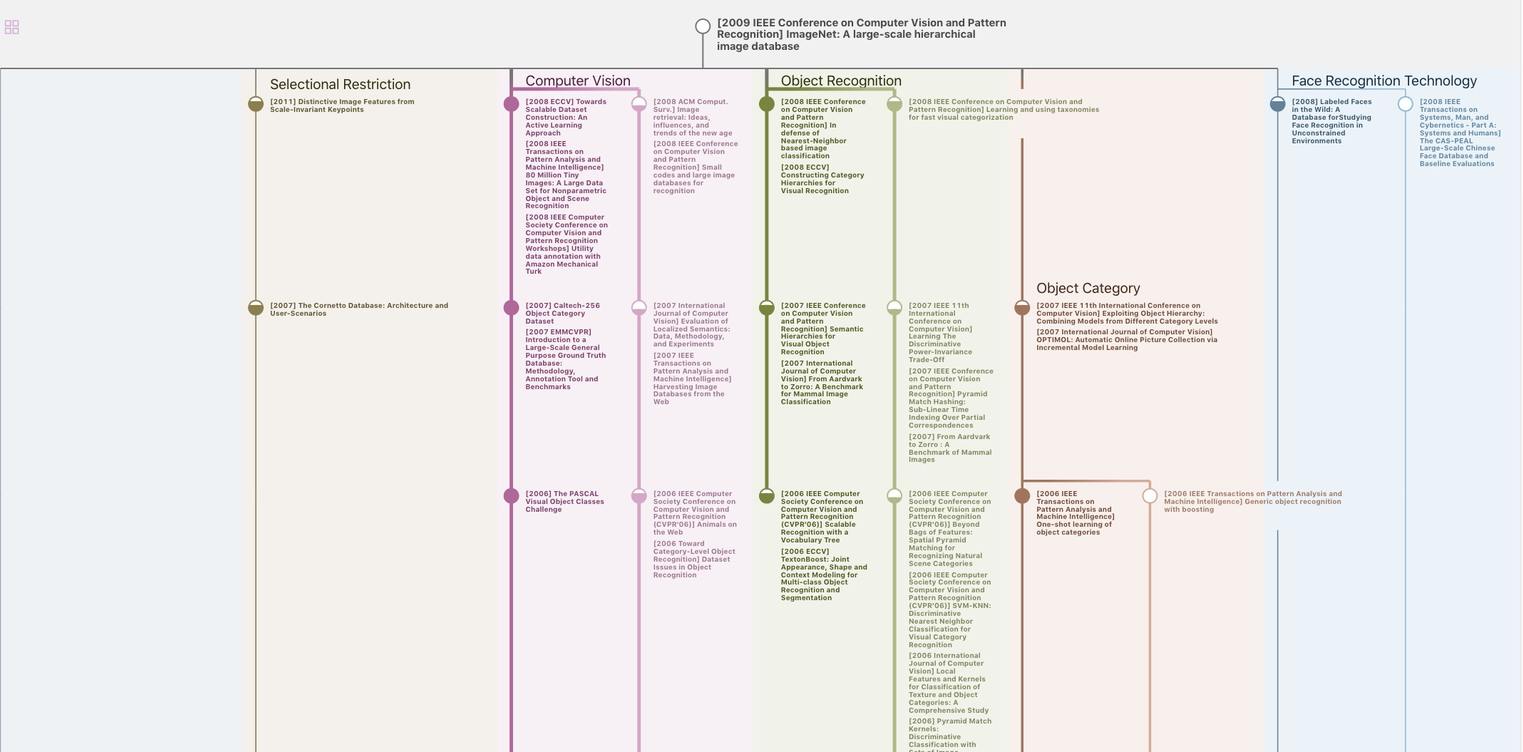
生成溯源树,研究论文发展脉络
Chat Paper
正在生成论文摘要