Interpretability-Guided Defense Against Backdoor Attacks to Deep Neural Networks
IEEE Transactions on Computer-Aided Design of Integrated Circuits and Systems(2022)
摘要
As an emerging threat to deep neural networks (DNNs), backdoor attacks have received increasing attentions due to the challenges posed by the lack of transparency inherent in DNNs. In this article, we develop an efficient algorithm from the interpretability of DNNs to defend against backdoor attacks to DNN models. To extract critical neurons, we deploy sets of control gates following neurons in layers, and the function of a DNN model can be interpreted as semantic sensitivities of neurons to input samples. A backdoor identification approach, derived from the activation frequency distribution on critical neurons, is proposed to reveal anomalies of particular neurons produced by backdoor attacks. Subsequently, a feasible and fine-grained pruning strategy is introduced to eliminate backdoors hidden in DNN models, without the need of retraining. Extensive experiments demonstrate that the proposed algorithm can identify and eliminate malicious backdoors efficiently in both single-target and multitarget scenarios with the performance of a DNN model retained to a large extent.
更多查看译文
关键词
Backdoor attack defense,backdoor elimination,backdoor identification,interpretability of deep neural networks (DNNs)
AI 理解论文
溯源树
样例
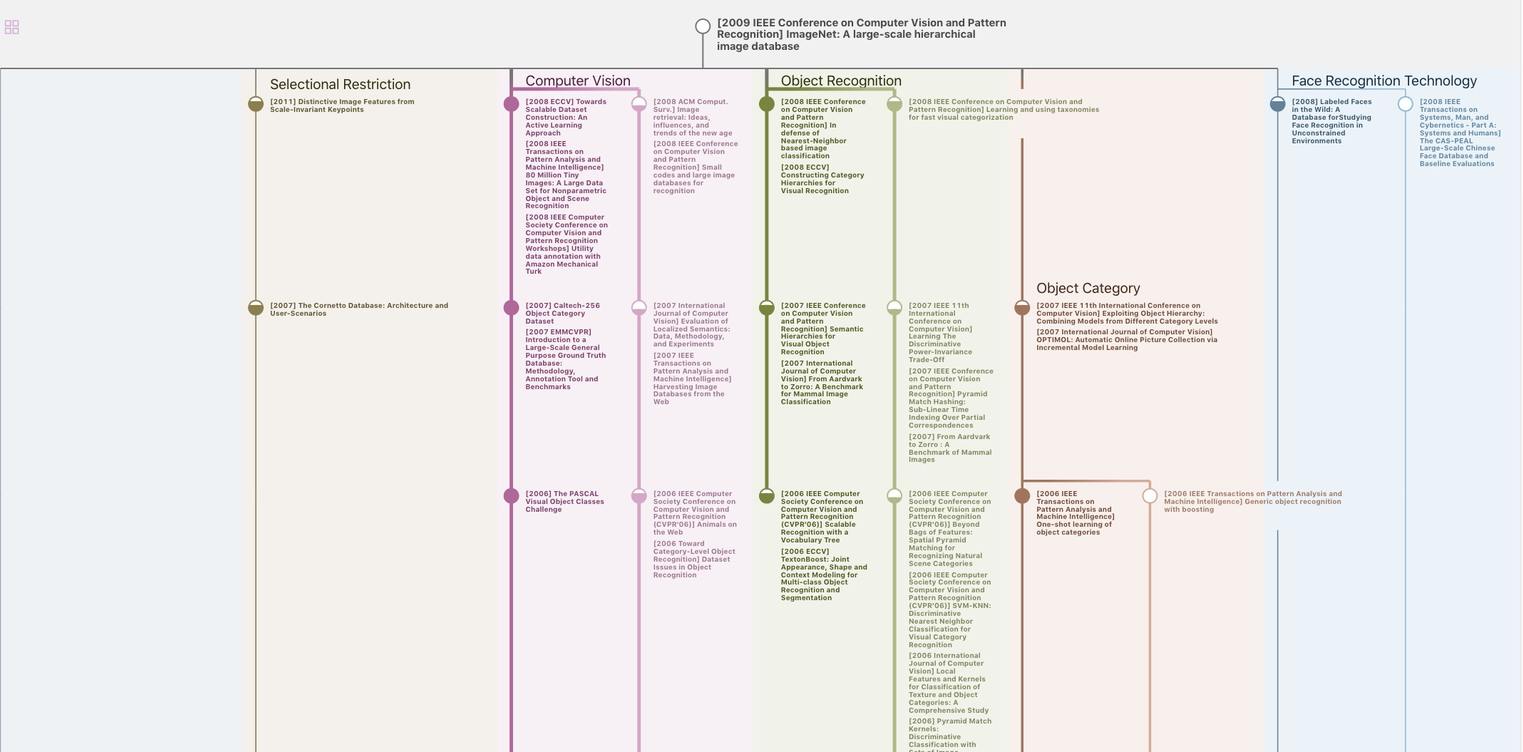
生成溯源树,研究论文发展脉络
Chat Paper
正在生成论文摘要