Profit vs. Equality? The Case of Financial Risk Assessment and A New Perspective on Alternative Data.
Social Science Research Network(2023)
摘要
While it is well noted the importance of pursuing inclusive finance to accelerate economic growth and financial sustainability, few actionable insights have been developed toward how financial institutions can balance the potential socioeconomic trade-off between equality and profitability. The challenges are largely three-folds: (1) lack of sufficient information (collateral); (2) lack of understanding of the impact of information on financial equality beyond economic profitability; and (3) lack of real-world causal evaluations under a (large) alternative set of counterfactual policies. Enlightened by the emerging sources of digitized user-behavior data (a.k.a. “alternative data”) enabled by the high penetration of mobile devices and internet access, we study how alternative data from smartphones and social media can help mitigate the potential financial inequality while preserving business profitability in the context of financial credit risk assessment. For evaluation, we partnered with a leading microloan platform and designed a “meta” field experiment that allowed us to recover and compare the “what-if” scenarios in the real world under an exhaustive set of counterfactual policies. Interestingly, we find that compared to using online social media information, profiling user financial risk using smartphone activities is 1.3 times more effective in improving financial inclusion (an increase of 23.05% vs. 18.11%), and likewise 1.3 times more effective in improving business profitability (an increase of 42% vs. 33%). Surprisingly, using consumers’ online shopping activities for credit risk profiling can hurt financial inclusion. Furthermore, we investigate the potential underlying mechanism for improved financial inclusion. Our findings suggest that alternative data, especially users’ smartphone activities, not only demonstrate higher ubiquity but also appear more orthogonal to conventional sensitive demographic attributes. This, in turn, can help better mitigate the statistical bias driven by unobserved factors or under-representative training samples during machine-based risk assessment processes.
更多查看译文
AI 理解论文
溯源树
样例
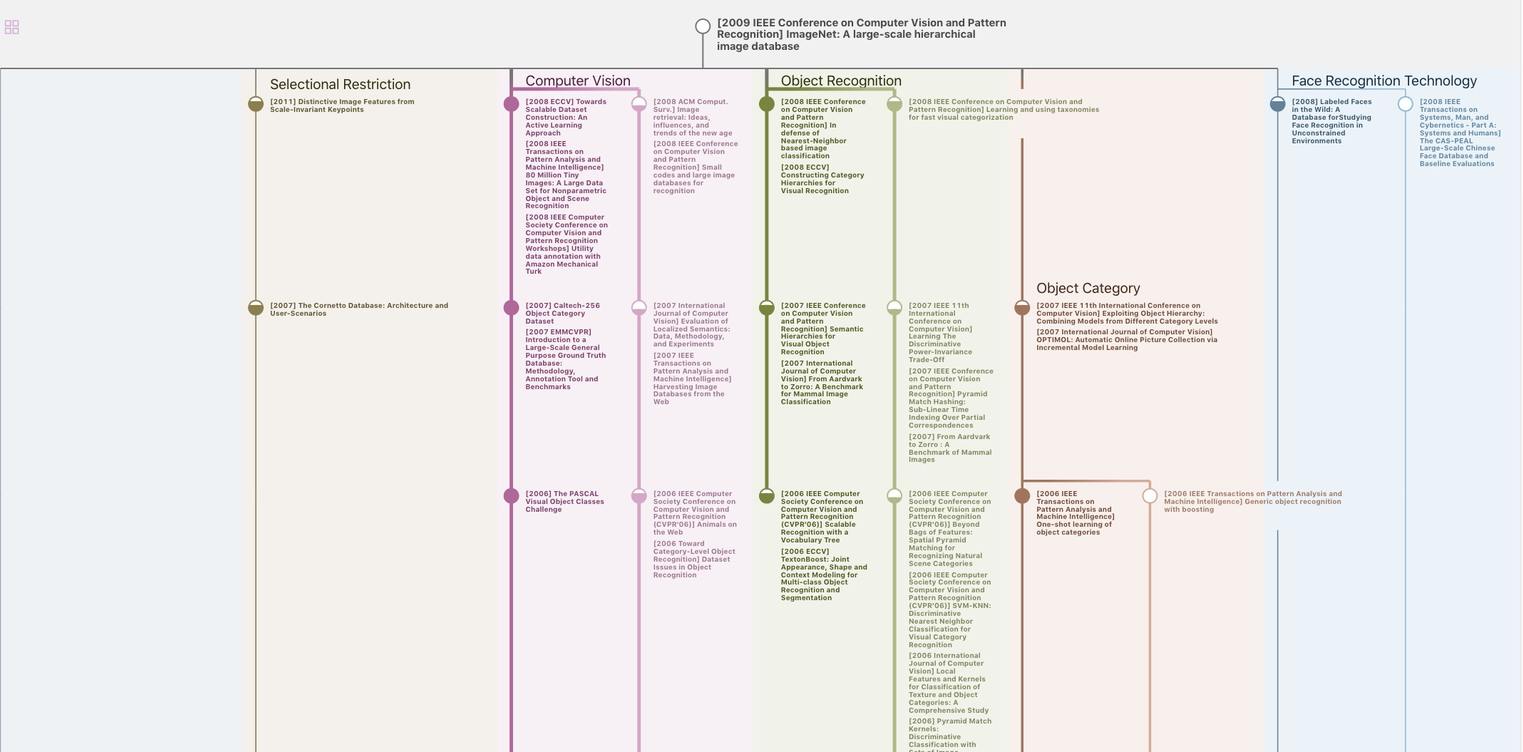
生成溯源树,研究论文发展脉络
Chat Paper
正在生成论文摘要