More than a Feeling: Benchmarks for Sentiment Analysis Accuracy
Social Science Research Network(2020)
摘要
The written word is the oldest and most common type of data. Today, mass literacy and cheap technology allow for greater word output per capita than ever before in human history. To keep pace, companies and scholars increasingly depend on automated analyses — not only of what people say (content) but also how they feel (sentiment). This makes it pertinent to understand the accuracy of these automated analyses. While information systems research has produced remarkable leaps of progress, the emphasis has been on innovation rather than evaluation. From an applied perspective, it is not clear whether leaderboard results for selected problems generalize across data sets and domains. In this article, we focus on sentiment analysis methods and assess performance across applications by combining a meta-analysis of 216 comparative computer science publications on 271 unique data sets with experimental evaluations of novel language models. To the best of our knowledge, this constitutes the most comprehensive assessment of sentiment analysis accuracy to date. We find that method choice explains only 10% of the variance in accuracy. Controlling for contextual factors such as data set and paper characteristics increases explanatory power to over 75%, suggesting differences across research problems matter. We find that accuracy of sentiment analysis can indeed approach 95% but can also fall below 50%. This shows that more nuanced benchmarks, rather than best attainable values for selected use cases, are more meaningful for an applied audience. We compute benchmark values that take both methodological choices and application context into account.
更多查看译文
AI 理解论文
溯源树
样例
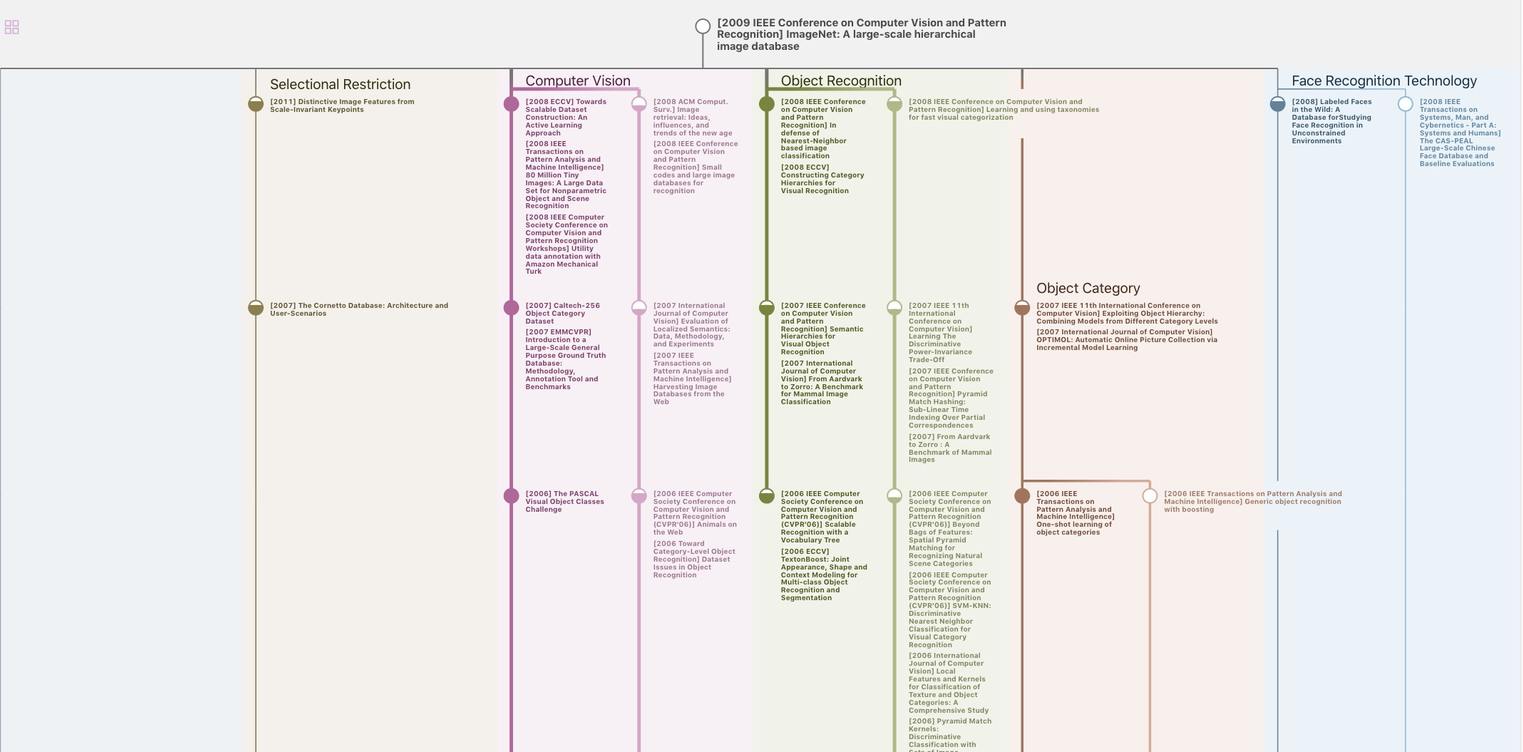
生成溯源树,研究论文发展脉络
Chat Paper
正在生成论文摘要