Spectral-Spatial Fusion Sub-Pixel Mapping Based on Deep Neural Network
IEEE GEOSCIENCE AND REMOTE SENSING LETTERS(2022)
摘要
Sub-pixel mapping (SPM) has been widely adopted to alleviate the mixed pixel problem in hyperspectral image, as an extension of spectral unmixing (SU), providing a way to observe the spatial location of the endmember within mixed pixel. However, most of the SPM methods are unmixing-then-mapping (UTM), i.e., SPM process relies on the abundance images generated from SU, in which process uncertainty inherently exists and would be propagated to SPM. Furthermore, the prior knowledge toward the sub-pixel scale distribution is mainly model-driven/handcrafted, which has limitation for geographical-realistic distribution representation. In this letter, we proposed spectral-spatial fusion SPM based on deep neural network (SSNET), to realize the integrative modeling of SU and SPM problem in a unified network fashion to avoid uncertainty accumulation in UTM process, and it can simultaneously generate SU result and SPM result. Besides, SSNET provides a supervised manner to learn prior knowledge with external exemplar pairs of low- and high-resolution images for a geographical-realistic distribution representation. The experiment with two hyperspectral images validated the superiority of the proposed SSNET.
更多查看译文
关键词
Hyperspectral imaging,Deep learning,Uncertainty,Training,Optimization,Mixture models,Imaging,Deep neural network,hyperspectral imagery,mixed pixel problem,spectral unmixing (SU),sub-pixel mapping (SPM)
AI 理解论文
溯源树
样例
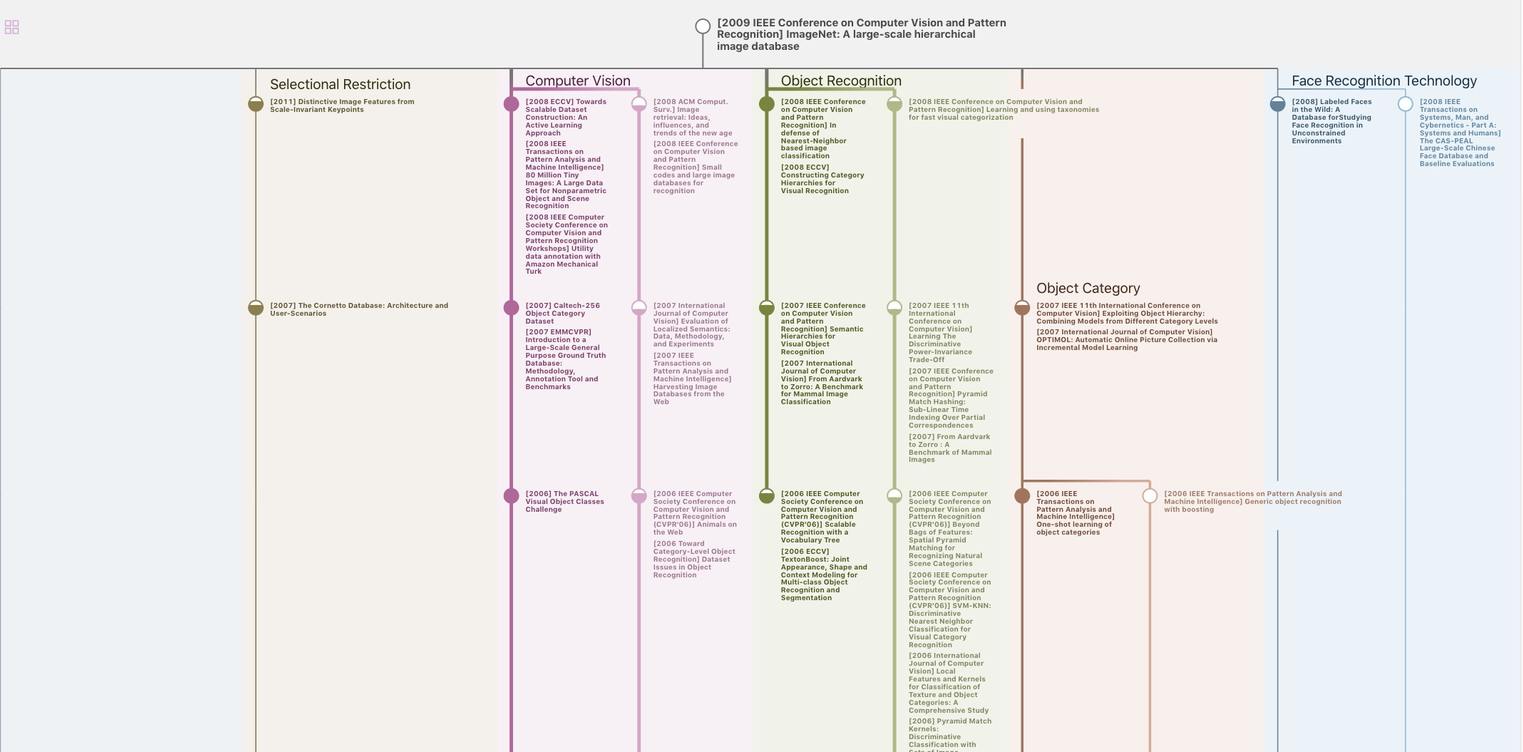
生成溯源树,研究论文发展脉络
Chat Paper
正在生成论文摘要