Deep Learning Investigation of Mass Spectrometry Analysis from Melanoma Samples
2019 IEEE International Symposium on Olfaction and Electronic Nose (ISOEN)(2019)
摘要
Deep learning has yet to be widely applied in the field of chemical gas sensing, in part due to the nature of this data. Many applications of chemical gas sensing suffer from a limited number of samples of high dimensional data. In this study, a novel data approach is introduced to address these issues and is applied to a dataset from mass spectrometry (MS) for melanoma detection. Samples were taken from 32 patients presenting with a dermatological mole. Various data analyses were performed. Traditional analysis such as primary component analysis (PCA), linear discriminant analysis (LDA), and a perceptron were used. In addition, a 1-hidden layer, fully connected neural network (also known as a multilayer perceptron) and a deeper 5-hidden layer, fully connected neural network was trained to classify inputs as deriving from a melanoma or non-melanoma mole. We segmented each sample and trained the network to assign a probabilistic output interpreted as “confidence in melanoma” to each segment. Traditional inference testing on these confidence measures found highly significant differences in the outputs of the multilayer perceptrons between melanoma versus non-melanoma samples. This provides a statistically grounded approach to deep learning-based classification of small amounts of high dimensional data, given the ability to segment the samples into a sufficient number of inputs for model training.
更多查看译文
关键词
deep learning,machine learning,tandem mass spectrometry,melanoma
AI 理解论文
溯源树
样例
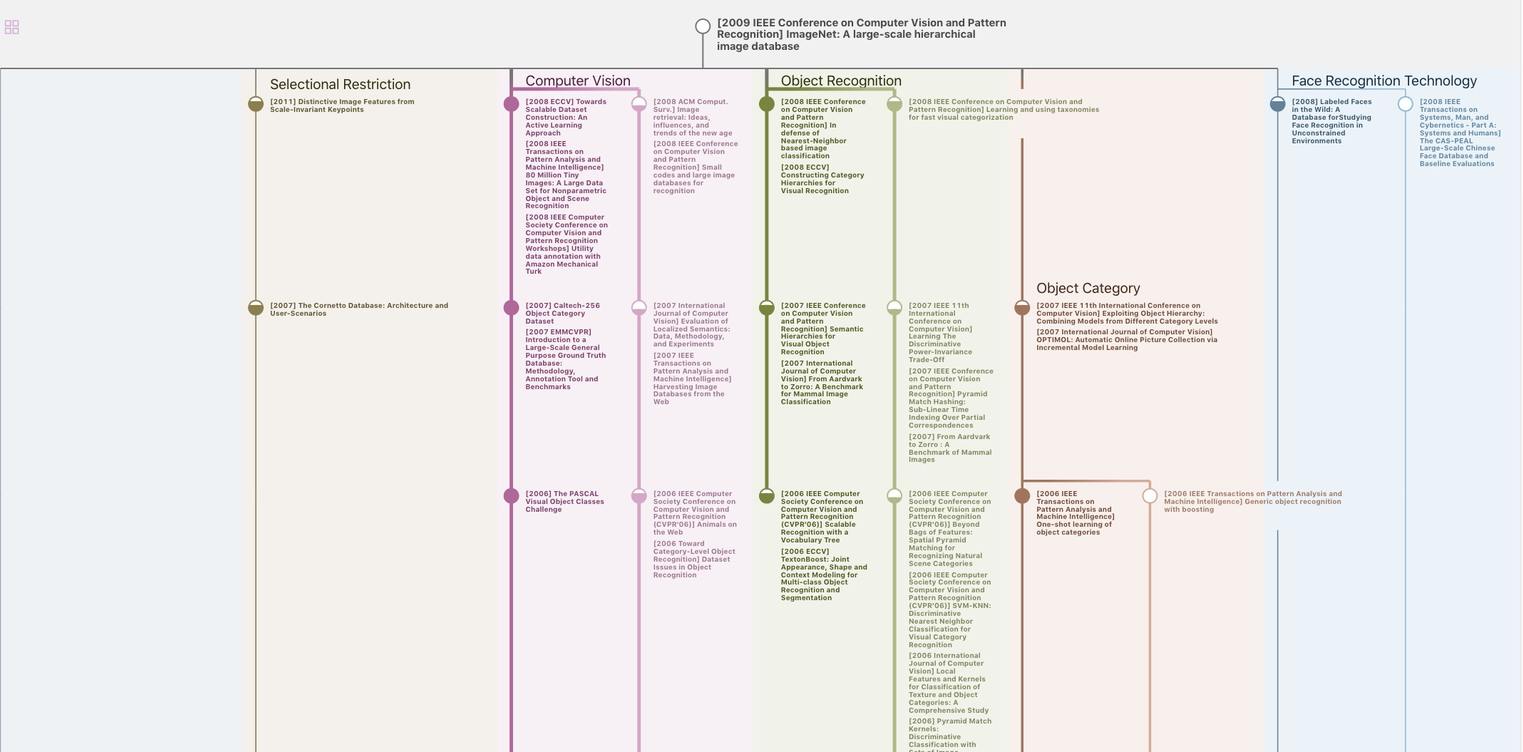
生成溯源树,研究论文发展脉络
Chat Paper
正在生成论文摘要