Scaling Effect of Self-Supervised Speech Models
conference of the international speech communication association(2021)
摘要
The success of modern deep learning systems is built on two cornerstones, massive amount of annotated training data and advanced computational infrastructure to support large-scale computation. In recent years, the model size of state-of-the-art deep learning systems has rapidly increased and sometimes reached to billions of parameters. Herein we take a close look into this phenomenon and present an empirical study on the scaling effect of model size for self-supervised speech models. In particular, we investigate the quantitative relationship between the model size and the loss/accuracy performance on speech tasks. First, the power-law scaling property between the number of parameters and the L-1 self-supervised loss is verified for speech models. Then the advantage of large speech models in learning effective speech representations is demonstrated in two downstream tasks: i) speaker recognition and ii) phoneme classification. Moreover, it has been shown that the model size of self-supervised speech networks is able to compensate the lack of annotation when there is insufficient training data. Index Terms: model size, self-supervised learning, power-law scaling, speaker recognition
更多查看译文
关键词
model size,self-supervised learning,power-law scaling,speaker recognition
AI 理解论文
溯源树
样例
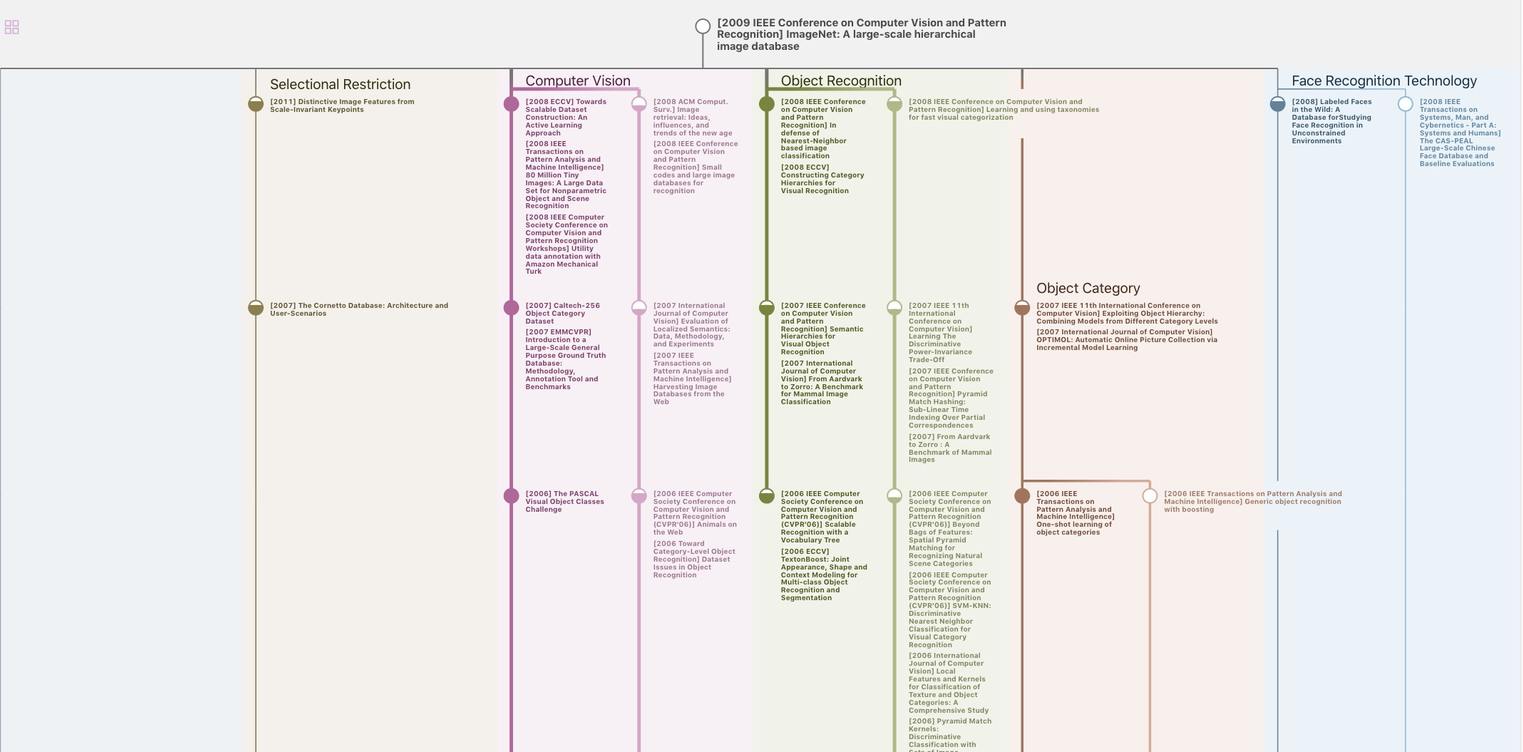
生成溯源树,研究论文发展脉络
Chat Paper
正在生成论文摘要